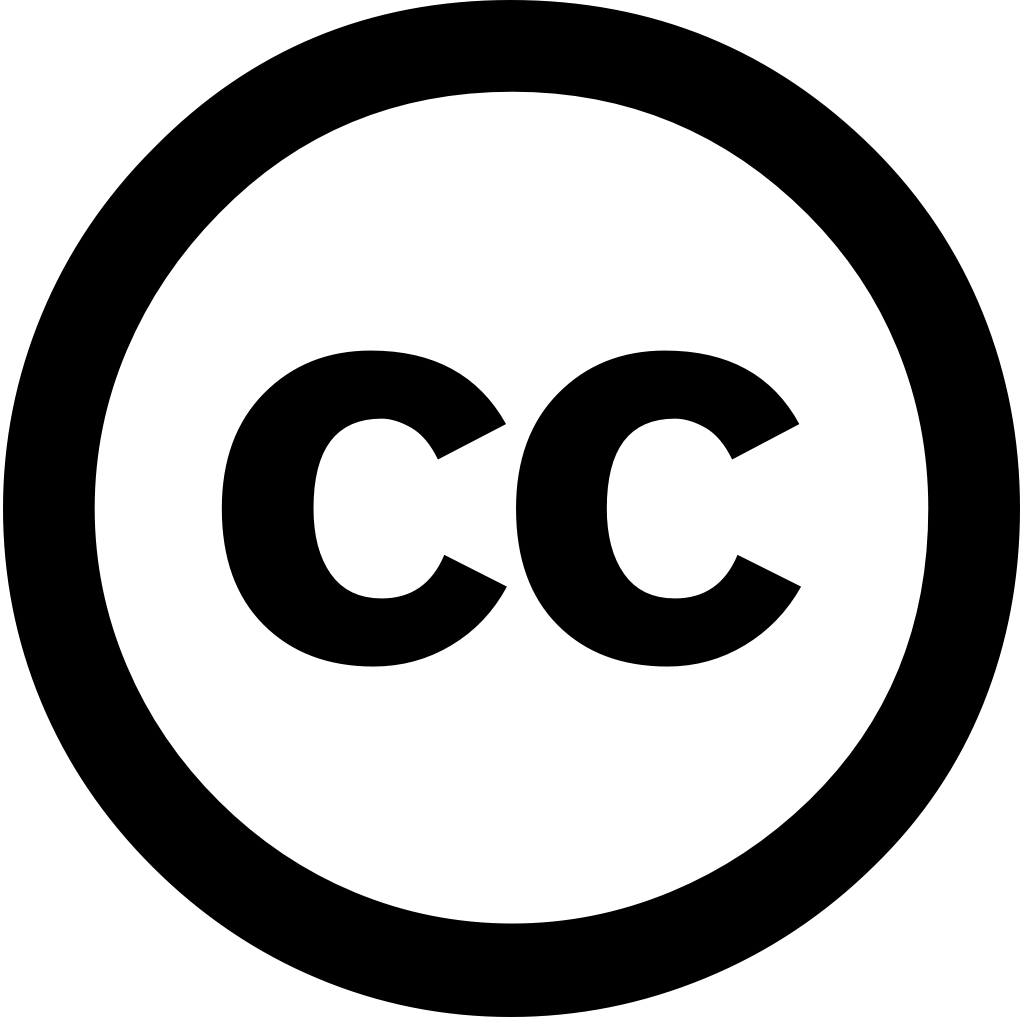
Research Square (Research Square), Journal Year: 2024, Volume and Issue: unknown
Published: Nov. 4, 2024
Language: Английский
Research Square (Research Square), Journal Year: 2024, Volume and Issue: unknown
Published: Nov. 4, 2024
Language: Английский
Results in Engineering, Journal Year: 2025, Volume and Issue: unknown, P. 103934 - 103934
Published: Jan. 1, 2025
Language: Английский
Citations
3Published: July 1, 2023
Handover is a crucial issue in ensuring the continuity of edge services computing (EC) systems. Failure to handle hand-off properly may result delays, data loss, or service interruption during switching. Therefore, optimizing process ensure and satisfactory user experience significant challenge design In this paper, we propose resource reservation algorithm that reserves portion resources each base station meet quality (QoS) requirements We use an LSTM model predict number new existing users at future time point provide decision guidance for algorithm. Extensive simulation experiments demonstrate proposed outperforms benchmark algorithms variety environmental conditions.
Language: Английский
Citations
3IEEE Access, Journal Year: 2023, Volume and Issue: 11, P. 89520 - 89534
Published: Jan. 1, 2023
With the rise in smart devices, challenges such as traffic management particularly indoor scenarios have also increased. Wireless technologies seek to address these needs by using new radio access that offer faster connectivity and Internet. While 4G 5G provide outdoor, Wi-Fi still remains dominant technology due its low cost easy deployment. However, IEEE 802.11 standard does not fairness of load user-access point (AP) associations, resulting unequal user distributions subpar resource utilization. Densification has been employed past aforementioned issue, but it is costly requires more hardware. Load balancing necessary high-density networks order guarantee quality service (QoS). To avoid densification issues related at APs this paper introduces tetra indicator associations (TiWA), a combined SDN-based association channel assignment solution for networks. TiWA considers multi-criteria make better decisions. The wireless stations from an overloaded OpenFlow enabled AP (OAP) are handed underloaded OAP considering multi-metrics packet loss rate, received signal strength (RSSI), throughput occupancy. Extensive experimentations on EmPOWER platform show surpasses previous research methods based terms aggregated 39%, offering superior level supporting greater numbers users prior hitting which required.
Language: Английский
Citations
3Optical and Quantum Electronics, Journal Year: 2023, Volume and Issue: 56(1)
Published: Dec. 13, 2023
Language: Английский
Citations
2Research Square (Research Square), Journal Year: 2024, Volume and Issue: unknown
Published: Nov. 4, 2024
Language: Английский
Citations
0