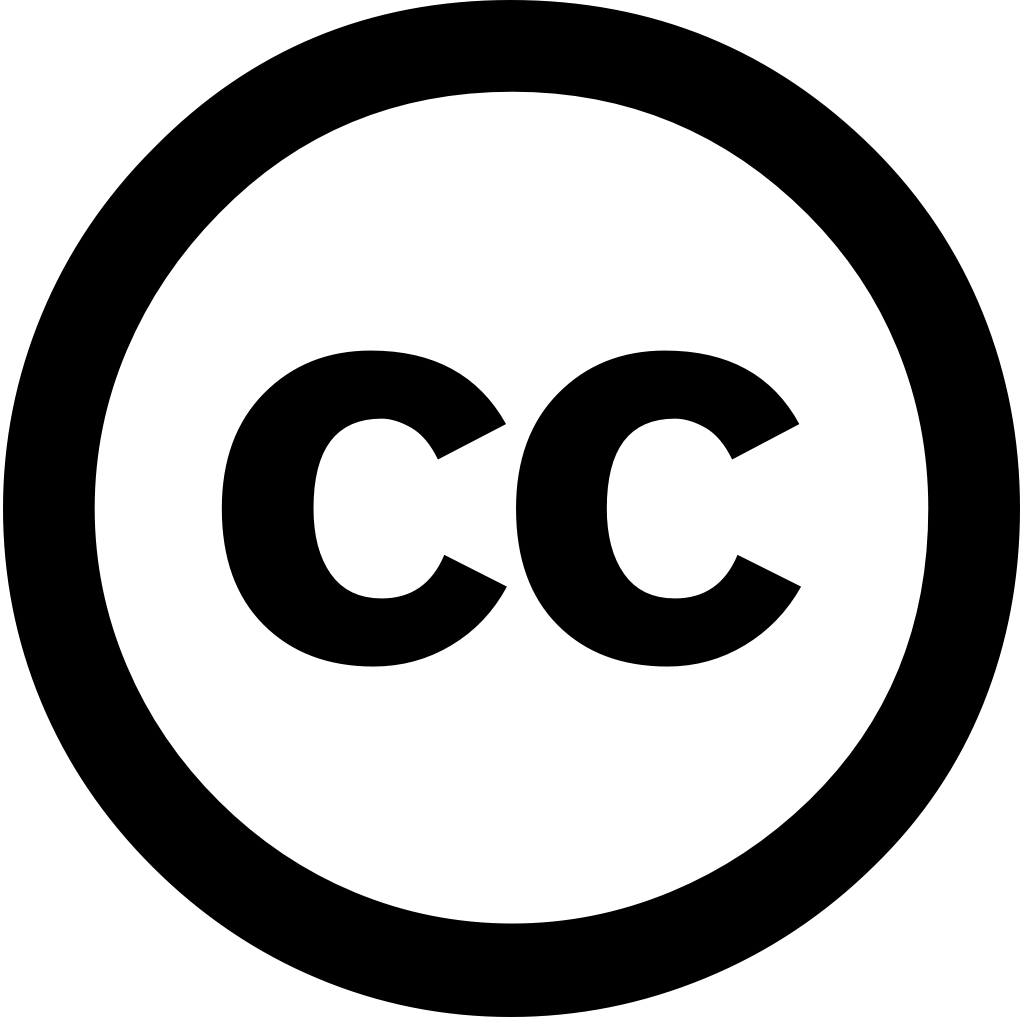
Ain Shams Engineering Journal, Journal Year: 2024, Volume and Issue: unknown, P. 103127 - 103127
Published: Oct. 1, 2024
Language: Английский
Ain Shams Engineering Journal, Journal Year: 2024, Volume and Issue: unknown, P. 103127 - 103127
Published: Oct. 1, 2024
Language: Английский
Water Research, Journal Year: 2023, Volume and Issue: 233, P. 119745 - 119745
Published: Feb. 16, 2023
Language: Английский
Citations
150Journal of Hydrology Regional Studies, Journal Year: 2023, Volume and Issue: 46, P. 101331 - 101331
Published: Feb. 7, 2023
Bisham Qilla and Doyian stations, Indus River Basin of Pakistan Water pollution is an international concern that impedes human health, ecological sustainability, agricultural output. This study focuses on the distinguishing characteristics evolutionary ensemble machine learning (ML) based modeling to provide in-depth insight escalating water quality problems. The 360 temporal readings electric conductivity (EC) total dissolved solids (TDS) with several input variables are used establish multi-expression programing (MEP) model random forest (RF) regression for assessment at River. developed models were evaluated using statistical metrics. findings reveal determination coefficient (R2) in testing phase (subject unseen data) all more than 0.95, indicating accurateness models. Furthermore, error measurements much lesser root mean square logarithmic (RMSLE) nearly equals zero each model. absolute percent (MAPE) MEP RF falls below 10% 5%, respectively, three phases (training, validation testing). According sensitivity generated about relevance inputs predicted EC TDS, shows bi-carbonates chlorine content have significant influence a sensitiveness score 0.90, whereas impact sodium less pronounced. All (RF MEP) lower uncertainty prediction interval coverage probability (PICP) calculated quartile (QR) approach. PICP% greater 85% stages. Thus, indicate developing intelligent parameter cost effective feasible monitoring analyzing quality.
Language: Английский
Citations
34Water, Journal Year: 2023, Volume and Issue: 15(9), P. 1750 - 1750
Published: May 2, 2023
Developing precise soft computing methods for groundwater management, which includes quality and quantity, is crucial improving water resources planning management. In the past 20 years, significant progress has been made in management using hybrid machine learning (ML) models as artificial intelligence (AI). Although various review articles have reported advances this field, existing literature must cover ML. This article aims to understand current state-of-the-art ML used achievements domain. It most cited employed from 2009 2022. summarises reviewed papers, highlighting their strengths weaknesses, performance criteria employed, highly identified. worth noting that accuracy was significantly enhanced, resulting a substantial improvement demonstrating robust outcome. Additionally, outlines recommendations future research directions enhance of including prediction related knowledge.
Language: Английский
Citations
33Journal of Molecular Liquids, Journal Year: 2024, Volume and Issue: 411, P. 125745 - 125745
Published: Aug. 11, 2024
Language: Английский
Citations
13Process Safety and Environmental Protection, Journal Year: 2025, Volume and Issue: unknown, P. 106816 - 106816
Published: Jan. 1, 2025
Language: Английский
Citations
1Discover Water, Journal Year: 2025, Volume and Issue: 5(1)
Published: Feb. 27, 2025
Language: Английский
Citations
1Water Research, Journal Year: 2023, Volume and Issue: 235, P. 119885 - 119885
Published: March 20, 2023
The issue of freshwater salinization in coastal areas has grown importance with the increase demand groundwater supply and more frequent droughts. However, spatial patterns salinity contamination are not easy to be understood, as well their numerical modeling is subject various kinds uncertainty. This paper offers a robust, flexible, reliable geostatistical methodology provide stochastic assessment distribution alluvial areas. applied aquifer Campania (Italy), where 83 monitoring wells provided depth-averaged data. A Monte Carlo (MC) framework was implemented simulate fields. Both MC fields mean across simulations enabled delineation which high salinity. Then, probabilistic approach developed setting up thresholds for agricultural use delineate unsuitable irrigation purposes. Furthermore, steady saline wedge lengths were unveiled through uncertainty estimates seawater ingression at Volturno River mouth. results compared versus calibrated model remarkable fit (R2=0.96) an analytical solution, obtaining similar lengths. pointed out that salinities found inland (more than 2 km from coastline) could ascribed trapped paleo-seawater rather actual intrusion. In fact, correspondence thick peaty layers, can store waters because porosity low permeability. these consistent recognition depositional environments position ancient lagoon sediments, located same highest (simulated) robust understand present salinization, disentangle intrusion, general zones
Language: Английский
Citations
16Environmental Modelling & Software, Journal Year: 2023, Volume and Issue: 168, P. 105788 - 105788
Published: Aug. 2, 2023
Language: Английский
Citations
15Frontiers in Earth Science, Journal Year: 2023, Volume and Issue: 11
Published: Sept. 7, 2023
Agriculture is considered one of the primary elements for socioeconomic stability in most parts Sudan. Consequently, irrigation water should be properly managed to achieve sustainable crop yield and soil fertility. This research aims predict indices sodium adsorption ratio (SAR), percentage (Na%), permeability index (PI), potential salinity (PS) using innovative machine learning (ML) techniques, including K-nearest neighbor (KNN), random forest (RF), support vector regression (SVR), Gaussian process (GPR). Thirty-seven groundwater samples are collected analyzed twelve physiochemical parameters (TDS, pH, EC, TH, Ca +2 , Mg Na + HCO 3 − Cl, SO 4 −2 NO ) assess hydrochemical characteristics its suitability purposes. The investigation indicated that dominated by Ca-Mg-HCO Na-HCO types resulted from recharge ion exchange reactions. observed SAR, Na%, PI, PS showed average values 7, 42.5%, 64.7%, 0.5, respectively. ML modeling based on ion’s concentration as input output. data divided into two sets training (70%) validation (30%), models validated a 10-fold cross-validation technique. tested with three statistical criteria, mean square error (MSE), root means (RMSE), correlation coefficient ( R 2 ). SVR algorithm best performance predicting indices, lowest RMSE value 1.45 SAR. other PS, were 6.70, 7.10, 0.55, applied digital predictive Nile River area Khartoum state, uncertainty maps was estimated running 10 times iteratively. standard deviation generated model’s sensitivity data, model can used identify areas where denser sampling needed improve accuracy estimates.
Language: Английский
Citations
12Journal of the Saudi Society of Agricultural Sciences, Journal Year: 2023, Volume and Issue: 23(1), P. 17 - 33
Published: Sept. 3, 2023
Collecting and analyzing data on surface water across extensive areas is a challenging, time-consuming expensive. Developing predictive models that offer high accuracy, reliability require minimal parameters can potentially reduce the time expense associated with quality monitoring management. While most existing studies have focused estimating point prediction of without approximating interval (PI) estimation, this study aimed to develop tool estimate PI indexes (WQIs) in lower Mun river basin. This was achieved by employing principal component analysis (PCA), artificial neural networks (ANN), bootstrap methods enhance robustness, minimum number parameters. PCA initially used select 4 for WQI. Subsequently, ANN regression employed new WQI evaluation efficiency. The testing results proposed model revealed its excellent performance compared other terms accuracy (root mean square error (RMSE) = 0.86, correlation coefficient (R) 0.993, scatter index (SI) 0.019, absolute (MAE) 0.709, bias (MBE) -0.003). Additionally, incorporated method quantify uncertainty create PI, resulting coverage rate exceeding 95%. By integrating statistical techniques intelligence quantifying uncertainty, it possible effectively evaluate quality, provide more accurate reliable indexes. be an effective decision makers planners seeking precise resource management strategies.
Language: Английский
Citations
11