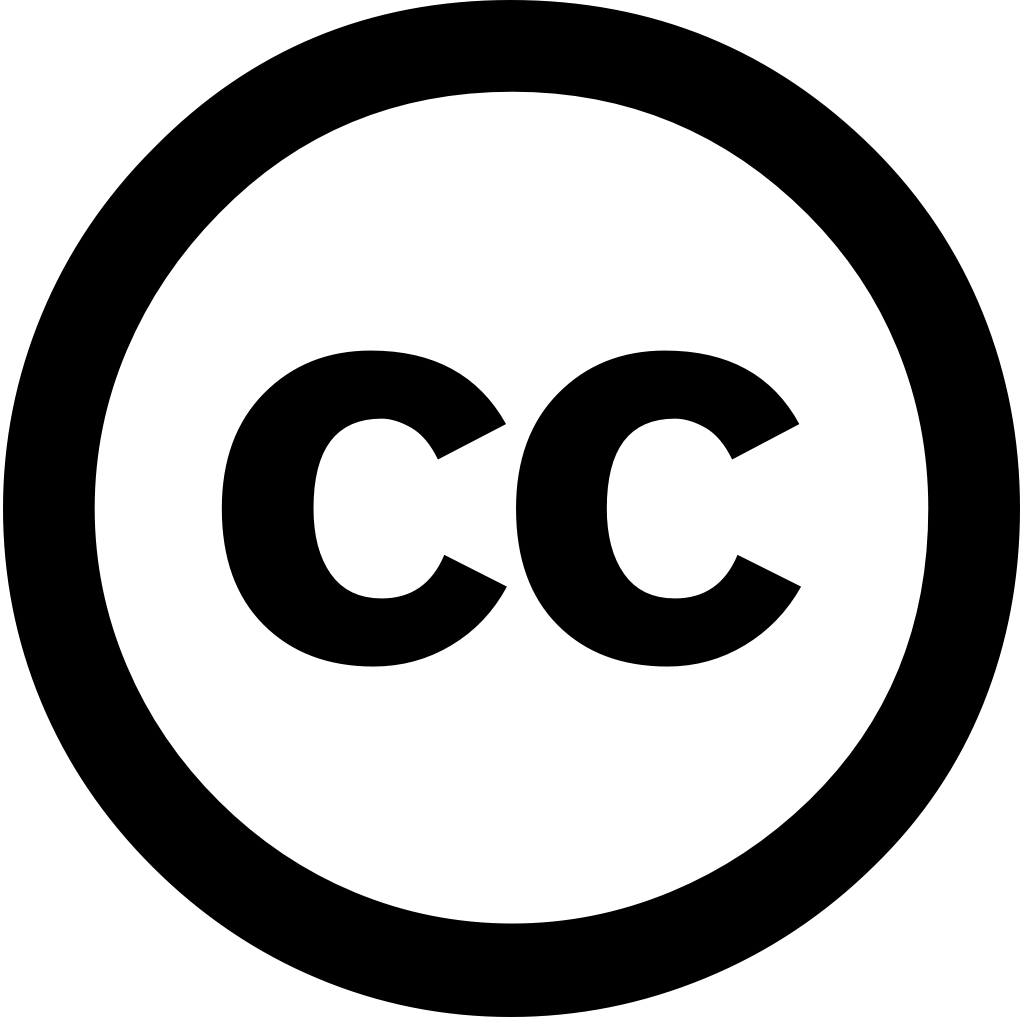
Results in Engineering, Journal Year: 2025, Volume and Issue: unknown, P. 104989 - 104989
Published: April 1, 2025
Language: Английский
Results in Engineering, Journal Year: 2025, Volume and Issue: unknown, P. 104989 - 104989
Published: April 1, 2025
Language: Английский
Environmental Science and Pollution Research, Journal Year: 2025, Volume and Issue: unknown
Published: March 1, 2025
Abstract Monitoring changes in groundwater quality over time helps identify time-dependent factors influencing water safety and supports the development of effective management strategies. This study investigates spatiotemporal evolution chemistry Debrecen area, Hungary, from 2019 to 2024, using indexing, machine learning, multivariate statistical techniques. These techniques include self-organizing maps (SOM), hierarchical cluster analysis (HCA), principal component (PCA), indexing (GWQI). The hydrochemical revealed that Ca-Mg-HCO₃ is dominant type, with a temporal shift toward Na-HCO₃, reflecting increased salinity driven by ongoing rock-water interactions. SOM showed transition heterogeneous more uniform time, suggesting greater stability aquifer system. Elevated zones shifted spatially due recharge flow patterns, while hardness intensified expanded, indicating continued carbonate dissolution. HCA highlighted shifts composition, six clusters identified five gradual homogenization quality. PCA further confirmed this trend, linking it underlying processes, such as water–rock interactions, limited contributions anthropogenic influences. GWQI indicated general improvement most regions meeting drinking standards. However, specific areas exhibited signs localized contamination, requiring targeted management. findings underscore importance continuous monitoring detect emerging trends guide resource highlights need for sustainable practices safeguard resources ensure long-term security area.
Language: Английский
Citations
3Results in Engineering, Journal Year: 2025, Volume and Issue: unknown, P. 104989 - 104989
Published: April 1, 2025
Language: Английский
Citations
0