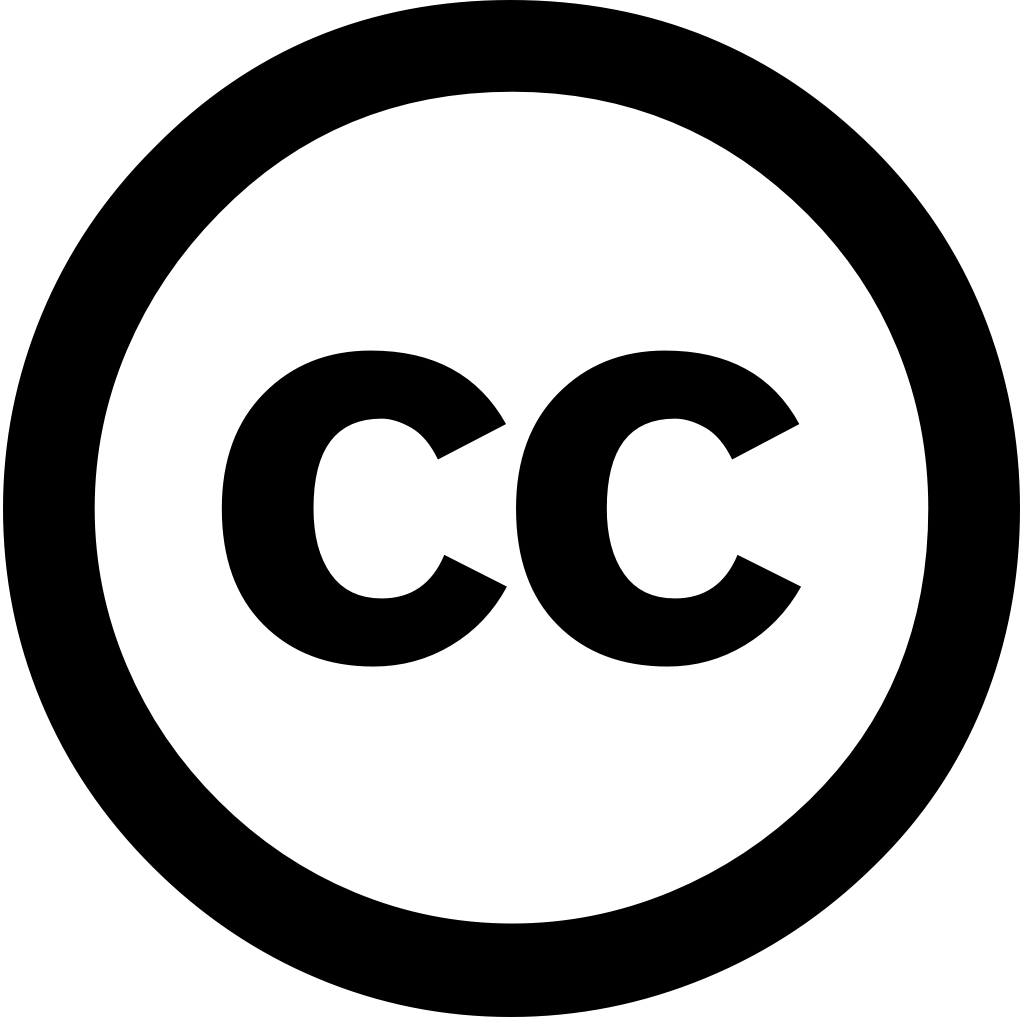
Land, Journal Year: 2025, Volume and Issue: 14(5), P. 931 - 931
Published: April 25, 2025
Unmanned aerial vehicles (UAVs) are rapidly becoming a popular tool for digital soil mapping at large-scale. However, their applicability in areas with homogeneous vegetation (i.e., not bare soil) has been fully investigated. In this study, we aimed to predict organic carbon, texture several depths, as well the thickness of AB horizon and penetration resistance using machine learning algorithm combination UAV images. We used an area Eurasian steppe zone (Republic Bashkortostan, Russia) covered Stipa type test plot, collected 192 samples from it. estimated models cross-validation approach spatial prediction uncertainties. To improve performance, also tested inclusion oblique geographic coordinates (OGCs) covariates that reflect position. The following results were achieved: (i) predictive demonstrated poor performance only images predictors; (ii) incorporation OGCs slightly improved predictions, whereas uncertainties remained high. conclude inability accurately properties these predictor variables (UAV OGC) is likely due limited access spectral signatures high variability within what appears be site, particularly relation soil-forming factors. Our limitations UAVs’ application modeling on site vegetation, including autocorrelation information can benefit should ignored further studies.
Language: Английский