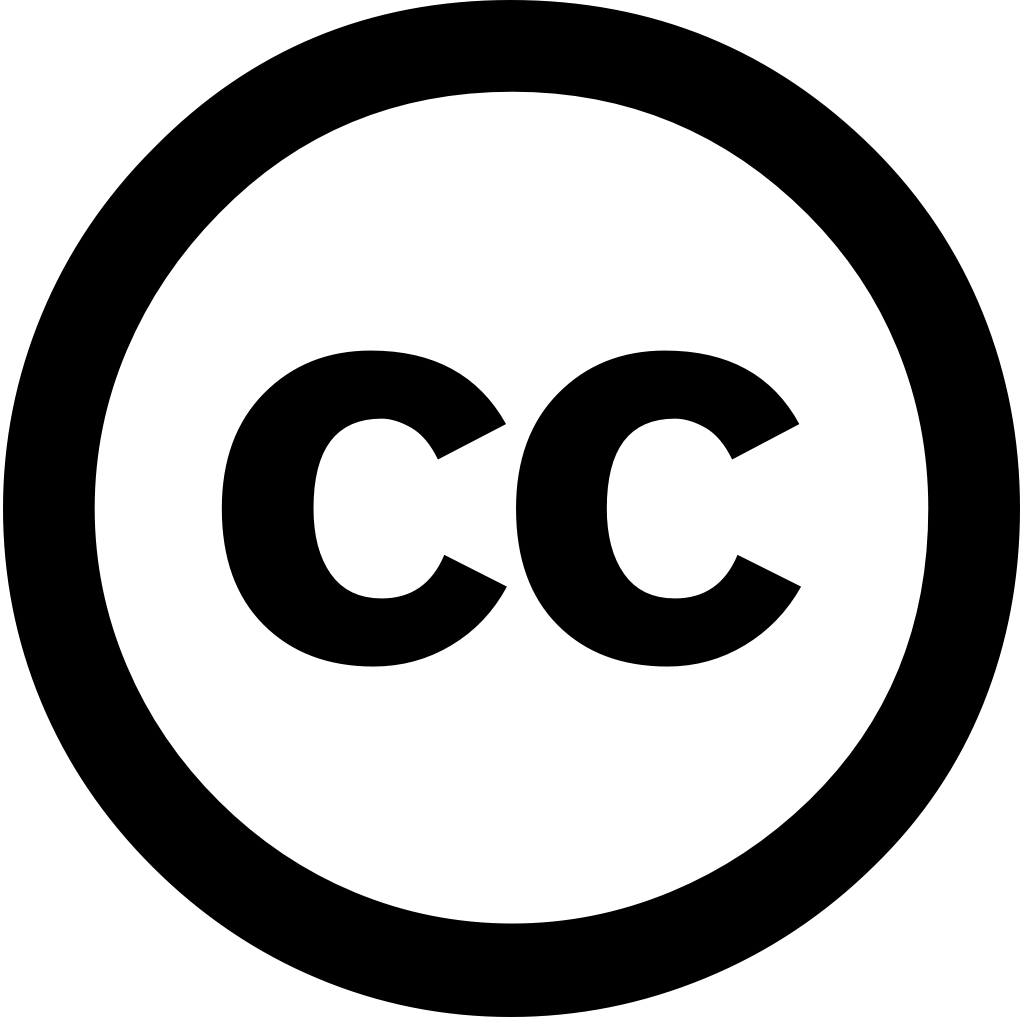
Research Square (Research Square), Journal Year: 2024, Volume and Issue: unknown
Published: March 18, 2024
Language: Английский
Research Square (Research Square), Journal Year: 2024, Volume and Issue: unknown
Published: March 18, 2024
Language: Английский
Current Oncology, Journal Year: 2024, Volume and Issue: 31(1), P. 403 - 424
Published: Jan. 10, 2024
The aim of this informative review was to investigate the application radiomics in cancer imaging and summarize results recent studies support oncological with particular attention breast cancer, rectal primitive secondary liver cancer. This also aims provide main findings, challenges limitations current methodologies. Clinical published last four years (2019–2022) were included review. Among 19 analyzed, none assessed differences between scanners vendor-dependent characteristics, collected images individuals at additional points time, performed calibration statistics, represented a prospective study registered database, conducted cost-effectiveness analysis, reported on clinical application, or multivariable analysis non-radiomics features. Seven reached high radiomic quality score (RQS), seventeen earned by using validation steps considering two datasets from distinct institutes open science data domains (radiomics features calculated set representative ROIs are source). potential is increasingly establishing itself, even if there still several aspects be evaluated before passage into routine practice. There challenges, including need for standardization across all stages workflow cross-site real-world heterogeneous datasets. Moreover, multiple centers more samples that add inter-scanner characteristics will needed future, as well collecting time points, reporting statistics performing database.
Language: Английский
Citations
11European Journal of Radiology, Journal Year: 2025, Volume and Issue: 185, P. 112013 - 112013
Published: Feb. 28, 2025
Language: Английский
Citations
1International Journal of Medical Informatics, Journal Year: 2025, Volume and Issue: 196, P. 105793 - 105793
Published: Jan. 21, 2025
Language: Английский
Citations
0Research Square (Research Square), Journal Year: 2025, Volume and Issue: unknown
Published: Feb. 3, 2025
Language: Английский
Citations
0Academic Radiology, Journal Year: 2025, Volume and Issue: unknown
Published: Feb. 1, 2025
Language: Английский
Citations
0Research Square (Research Square), Journal Year: 2025, Volume and Issue: unknown
Published: May 15, 2025
Language: Английский
Citations
0Current Breast Cancer Reports, Journal Year: 2024, Volume and Issue: 16(2), P. 134 - 141
Published: March 18, 2024
Abstract Purpose of Review Since its approval by the Unites States Food and Drug Administration (FDA) in 2015, cone-beam breast computed tomography (CBBCT) has gained acceptance among radiologists for cancer imaging. This review aims to highlight advancements benefits CBBCT diagnostic workup disease. It showcases how CBBCT, including both non-contrast (NC-CBBCT) contrast-enhanced (CE-CBBCT) protocols, complements often surpasses performance more traditional imaging modalities such as mammography magnetic resonance (MRI). Recent Findings Studies clinical settings have shown CBBCT’s efficacy detecting characterizing lesions differing morphologies, non-mass enhancement calcifications—tasks that previously required use multiple modalities. In addition, significantly enhances patient comfort efficiency, offering quick acquisition times without discomfort compression. The technology can be utilized guiding biopsies, planning surgical interventions, assessing density tumor characteristics, evidence supporting integration into practice. Summary holds potential shift paradigm care, indicating a promising future modality terms enhancing accuracy, improving experience, influencing treatment outcomes.
Language: Английский
Citations
3International Journal of Surgery, Journal Year: 2024, Volume and Issue: 110(9), P. 5363 - 5373
Published: June 7, 2024
Background: The accuracy of traditional clinical methods for assessing the metastatic status axillary lymph nodes (ALNs) is unsatisfactory. In this study, authors propose use radiomic technology and three-dimensional (3D) visualization to develop an unsupervised learning model predicting node metastasis in patients with breast cancer (BC), aiming provide a new method assessment disease. Methods: we retrospectively analyzed data 350 invasive BC who underwent lung-enhanced computed tomography (CT) dissection surgery at Department Breast Surgery Second Xiangya Hospital Central South University. used 3D create atlas ALNs identified region interest nodes. Radiomic features were subsequently extracted selected, prediction was constructed using K-means algorithm. To validate model, prospectively collected from 128 clinically evaluated as negative our center. Results: Using technology, selected total 36 CT radiomics features. categorized 1737 unlabeled into two groups, analysis between these groups indicated potential differences status. Further validation 1397 labeled demonstrated that had good predictive ability status, area under curve 0.847 (0.825–0.869). Additionally, model’s excellent performance confirmed cohort (cN0) positive (cN+) cohort, which correct classification rates 86.72 87.43%, respectively, significantly greater than those methods. Conclusions: created accurately predicts ALNs. This approach offers novel solution precise BC.
Language: Английский
Citations
3Deleted Journal, Journal Year: 2024, Volume and Issue: unknown
Published: Feb. 23, 2024
The goal of this study was to evaluate the performance a convolutional neural network (CNN) with preoperative MRI and clinical factors in predicting treatment response unresectable hepatocellular carcinoma (HCC) patients receiving hepatic arterial infusion chemotherapy (HAIC). A total 191 HCC who underwent HAIC our hospital between May 2019 March 2022 were retrospectively recruited. We selected InceptionV4 from three representative CNN models, AlexNet, ResNet, InceptionV4, according cross-entropy loss (CEL). subsequently developed fuse information qualified pretreatment data patient factors. Radiomic evaluated based on several constant sequences, including enhanced T1-weighted sequences (with arterial, portal, delayed phases), T2 FSE dual-echo sequences. cross-validated training cohort (n = 127) internally validated an independent 64), comparisons against single important radiologists terms receiver operating characteristic (ROC) curves. Class activation mapping used visualize model. model achieved AUC 0.871 (95% confidence interval [CI] 0.761–0.981) cross-validation 0.826 CI 0.682–0.970) internal validation cohort; these two models performed better than did other methods (AUC ranges 0.783–0.873 0.708–0.806 for cross- validations, respectively; P < 0.01). present model, which integrates radiomic factors, helps predict treatment.
Language: Английский
Citations
1Medicine, Journal Year: 2024, Volume and Issue: 103(24), P. e38513 - e38513
Published: June 14, 2024
To explore the value of machine learning (ML) models based on contrast-enhanced cone-beam breast computed tomography (CE-CBBCT) radiomics features for preoperative prediction human epidermal growth factor receptor 2 (HER2)-low expression cancer (BC). Fifty-six patients with HER2-negative invasive BC who underwent CE-CBBCT were prospectively analyzed. Patients randomly divided into training and validation cohorts at approximately 7:3. A total 1046 quantitative radiomic extracted from images normalized using z-scores. The Pearson correlation coefficient recursive feature elimination used to identify optimal features. Six ML constructed selected features: linear discriminant analysis (LDA), random forest (RF), support vector (SVM), logistic regression (LR), AdaBoost (AB), decision tree (DT). evaluate performance these models, receiver operating characteristic curves area under curve (AUC) used. Seven as constructing models. In cohort, AUC values SVM, LDA, RF, LR, AB, DT 0.984, 0.981, 1.000, 0.970, respectively. 0.859, 0.880, 0.781, 0.750, 0.713, Among all LDA LR demonstrated best performance. DeLong test showed that there no significant differences among in cohort (P > .05); however, between AUCs statistically = .037, .003, .046). .023, .005, .030). Nevertheless, observed when compared other achieved excellent HER2-low could potentially serve an effective tool assist precise personalized targeted therapy.
Language: Английский
Citations
1