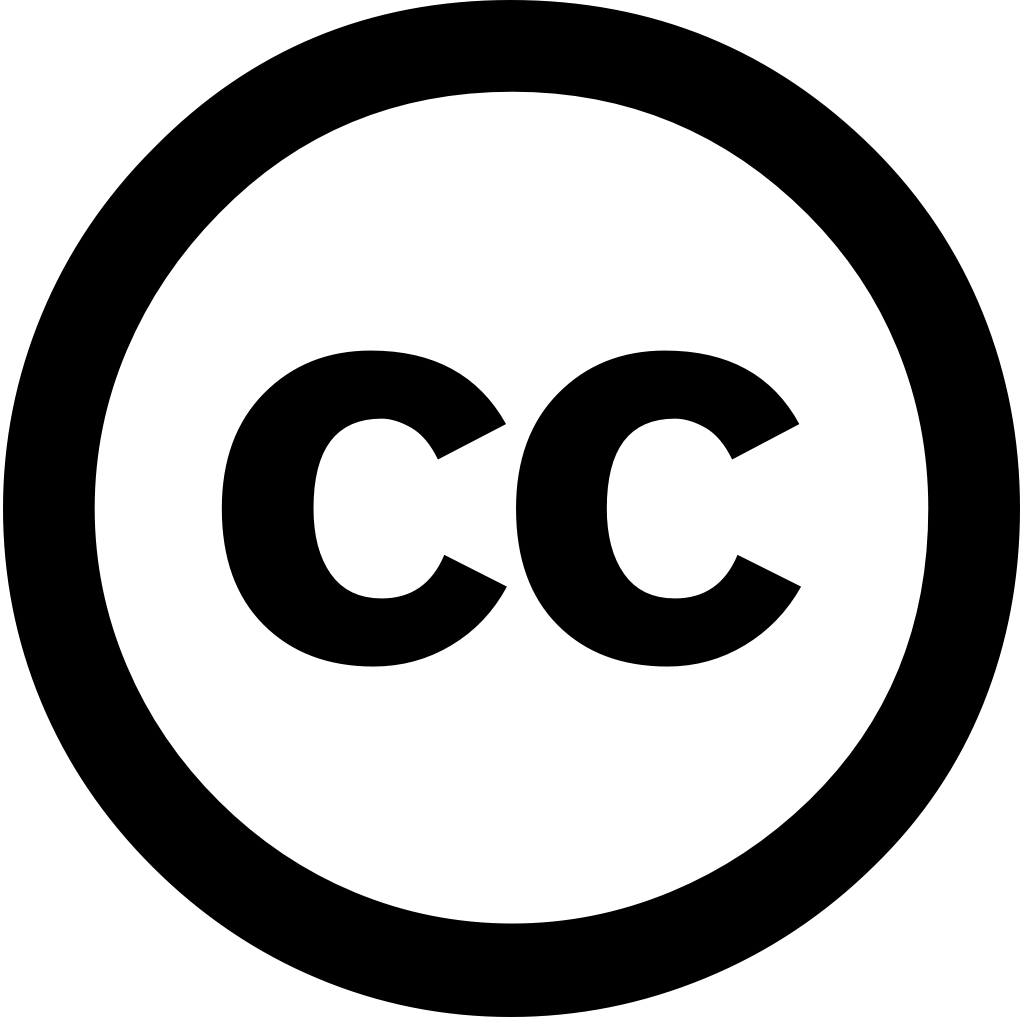
IoT, Journal Year: 2025, Volume and Issue: 6(2), P. 29 - 29
Published: May 8, 2025
The rise of wearable devices has enabled real-time processing sensor data for critical health monitoring applications, such as human activity recognition (HAR) and cardiac disorder classification (CDC). However, the limited computational memory resources wearables necessitate lightweight yet accurate models. While deep neural networks (DNNs), including convolutional (CNNs) long short-term networks, have shown high accuracy HAR CDC, their large parameter sizes hinder deployment on edge devices. On other hand, various DNN compression techniques been proposed, but exploiting combination with aim achieving efficient models CDC tasks remains under-investigated. This work studies impact CNN architecture parameters, focusing dense layers, to identify configurations that balance efficiency. We derive two versions each model—lean fat—based characteristics. Subsequently, we apply three complementary techniques: filter-based pruning, low-rank factorization, dynamic range quantization. Experiments across diverse DNNs demonstrate this multi-faceted approach can significantly reduce requirements while maintaining validation accuracy, leading suitable intelligent resource-constrained
Language: Английский