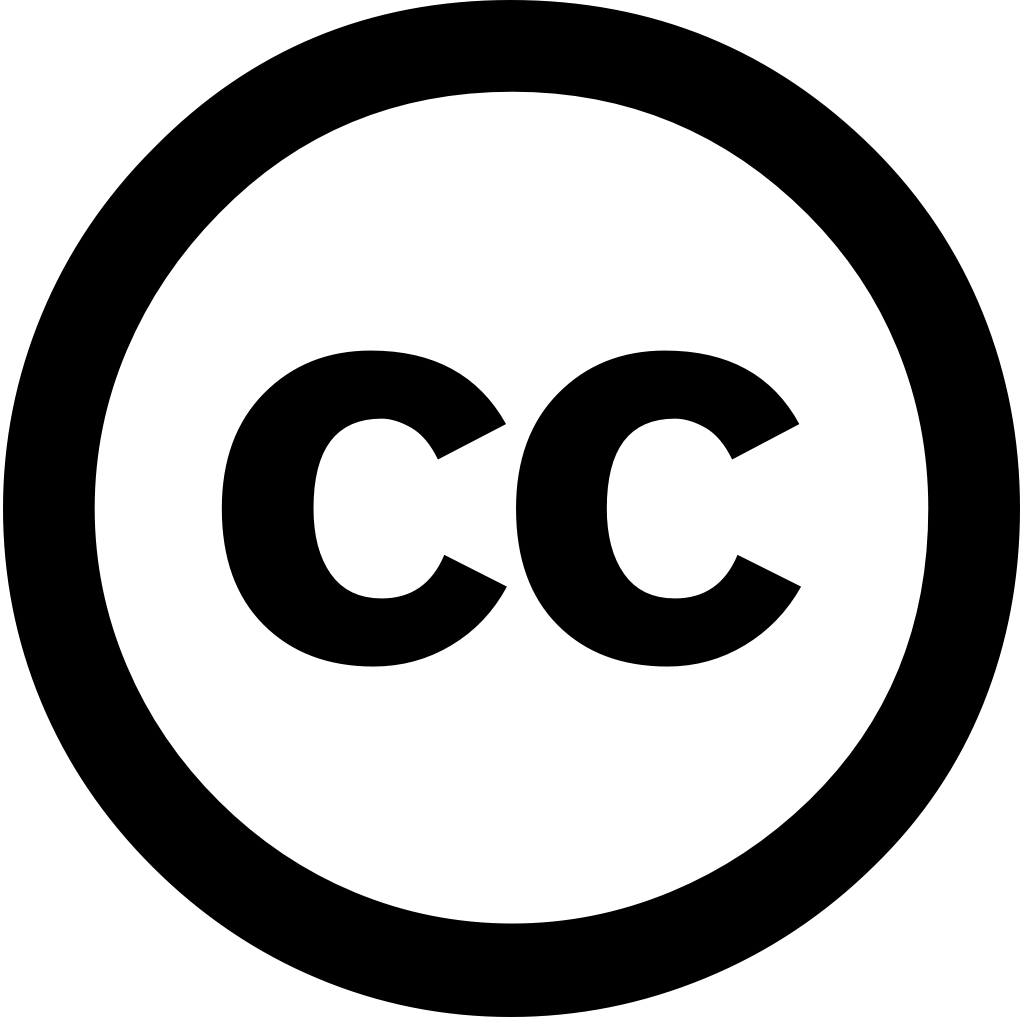
Sensors, Journal Year: 2025, Volume and Issue: 25(2), P. 547 - 547
Published: Jan. 18, 2025
Tea bud localization detection not only ensures tea quality, improves picking efficiency, and advances intelligent harvesting, but also fosters industry upgrades enhances economic benefits. To solve the problem of high computational complexity deep learning models, we developed Bud DSCF-YOLOv8n (TBF-YOLOv8n)lightweight model. Improvement Cross Stage Partial Bottleneck Module with Two Convolutions(C2f) module via efficient Distributed Shift Convolution (DSConv) yields C2f DSConv(DSCf)module, which reduces model’s size. Additionally, coordinate attention (CA) mechanism is incorporated to mitigate interference from irrelevant factors, thereby improving mean accuracy. Furthermore, SIOU_Loss (SCYLLA-IOU_Loss) function Dynamic Sample(DySample)up-sampling operator are implemented accelerate convergence enhance both average precision The experimental results show that compared YOLOv8n model, TBF-YOLOv8n model has a 3.7% increase in accuracy, 1.1% 44.4% reduction gigabit floating point operations (GFLOPs), 13.4% total number parameters included In comparison experiments variety lightweight still performs well terms accuracy while remaining more lightweight. conclusion, achieves commendable balance between efficiency precision, offering valuable insights for advancing harvesting technologies.
Language: Английский