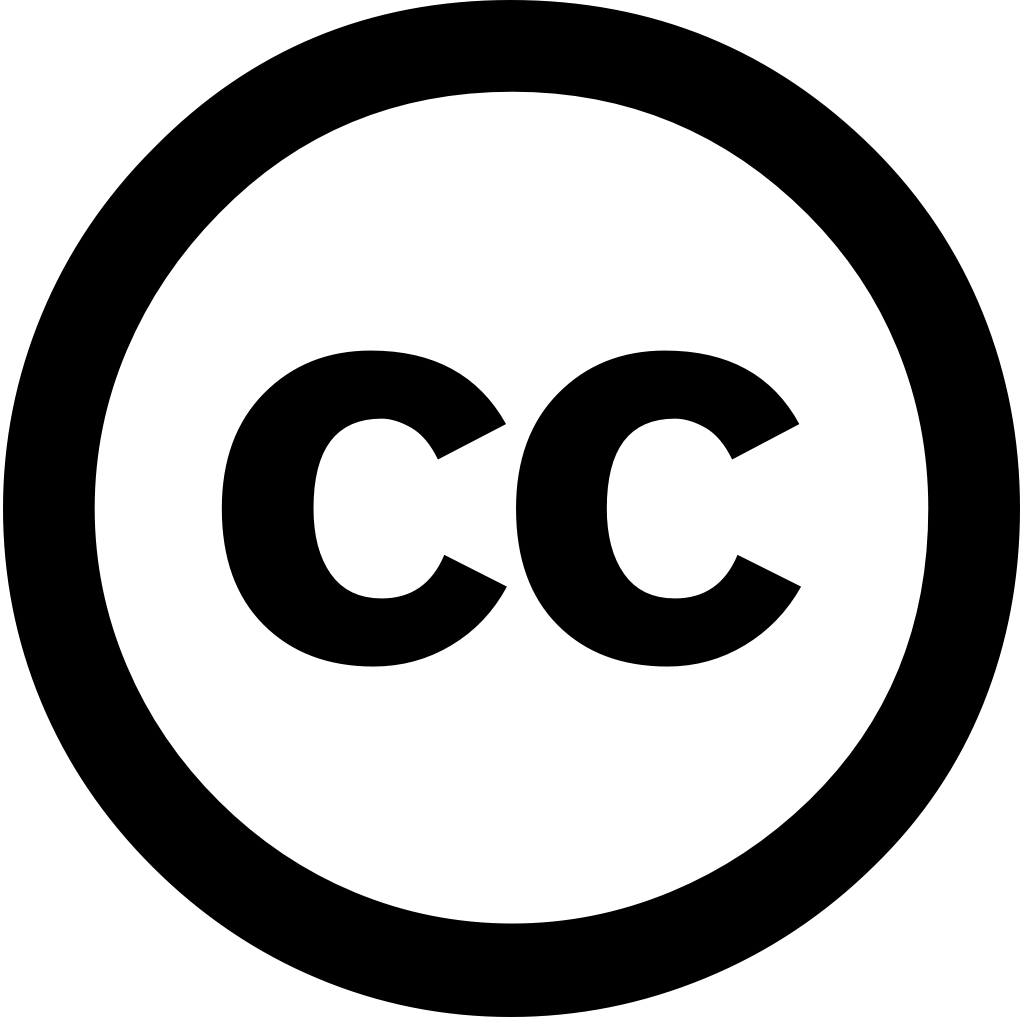
Entropy, Journal Year: 2024, Volume and Issue: 27(1), P. 14 - 14
Published: Dec. 27, 2024
Brain-computer interfaces (BCI) are an effective tool for recognizing motor imagery and have been widely applied in the control assistive operation domains. However, traditional intention-recognition methods face several challenges, such as prolonged training times limited cross-subject adaptability, which restrict their practical application. This paper proposes innovative method that combines a lightweight convolutional neural network (CNN) with domain adaptation. A feature extraction module is designed to extract key features from both source target domains, effectively reducing model's parameters improving real-time performance computational efficiency. To address differences sample distributions, adaptation strategy introduced optimize alignment. Furthermore, adversarial employed promote learning of domain-invariant features, significantly enhancing generalization ability. The proposed was evaluated on fNIRS dataset, achieving average accuracy 87.76% three-class classification task. Additionally, experiments were conducted two perspectives: model structure optimization data selection. results demonstrated potential advantages this applications recognition systems.
Language: Английский