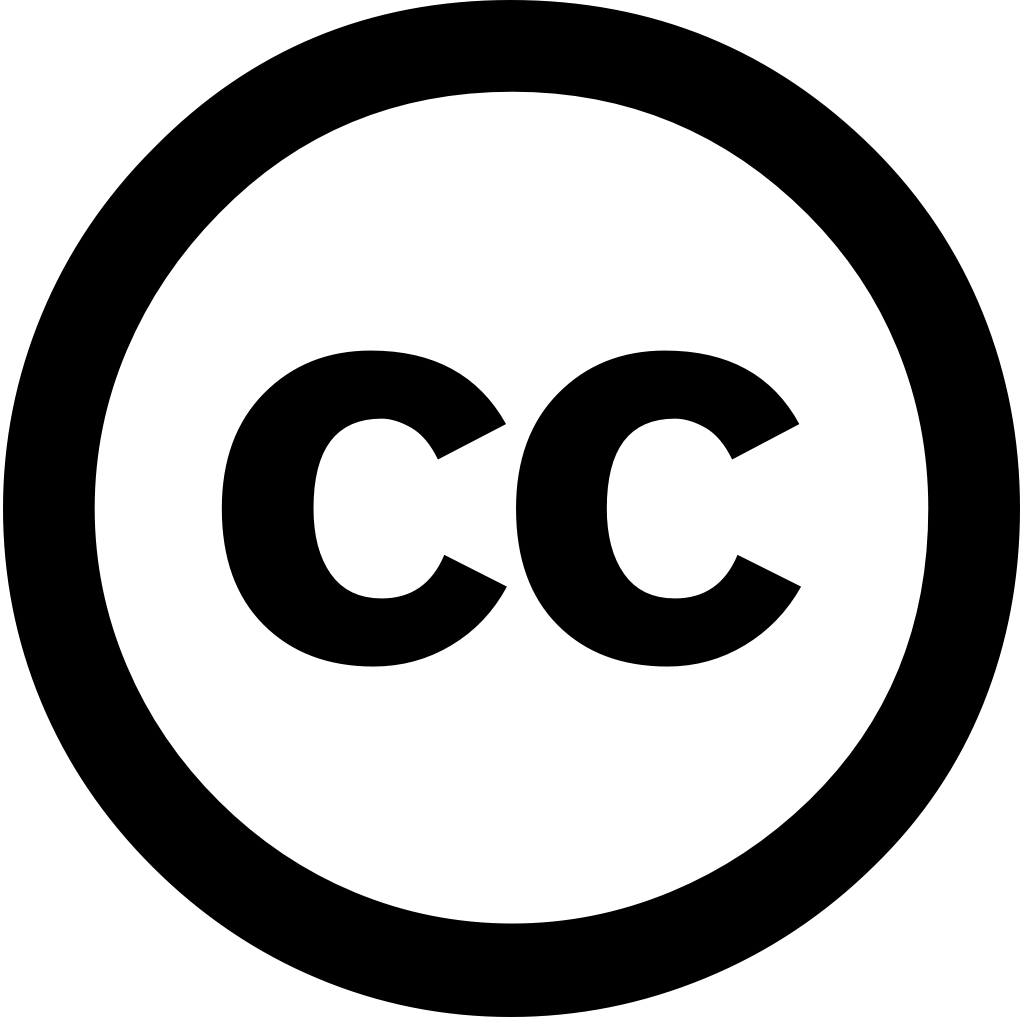
Metals, Journal Year: 2025, Volume and Issue: 15(4), P. 408 - 408
Published: April 4, 2025
In steel structural engineering, artificial intelligence (AI) and machine learning (ML) are improving accuracy, efficiency, automation. This review explores AI-driven approaches, emphasizing how AI models improve predictive capabilities, optimize performance, reduce computational costs compared to traditional methods. Inverse Machine Learning (IML) is a major focus since it helps engineers minimize reliance on iterative trial-and-error by allowing them identify ideal material properties geometric configurations depending predefined performance targets. Unlike conventional ML that mostly forward predictions, IML data-driven design generation, enabling more adaptive engineering solutions. Furthermore, underlined Explainable Artificial Intelligence (XAI), which enhances model transparency, interpretability, trust of AI. The paper categorizes applications in construction based their impact automation, health monitoring, failure prediction evaluation throughout research from 1990 2025. challenges such as data limitations, generalization, reliability, the need for physics-informed while examining AI’s role bridging real-world applications. By integrating into this work supports adoption ML, IML, XAI analysis design, paving way reliable interpretable practices.
Language: Английский