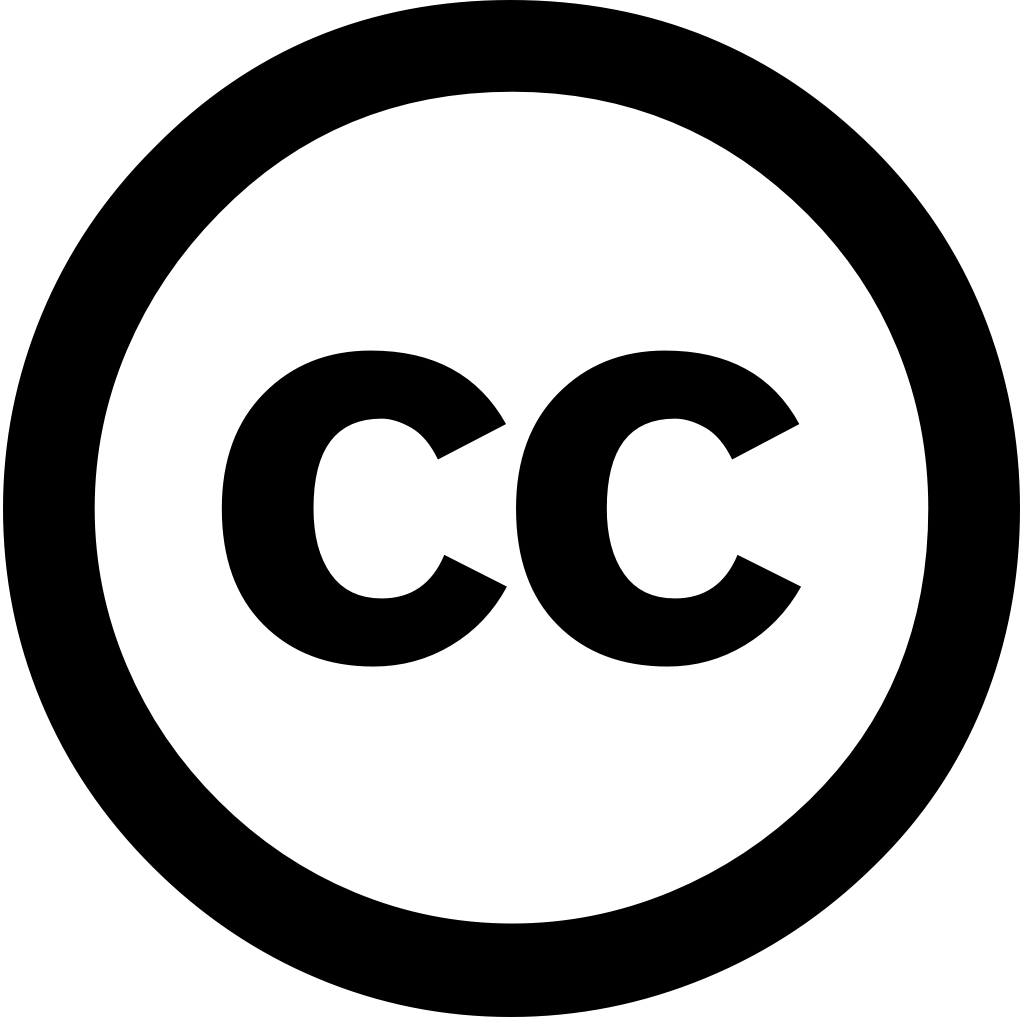
Applied Sciences, Journal Year: 2024, Volume and Issue: 14(24), P. 11636 - 11636
Published: Dec. 12, 2024
Airwave interference presents a major source of noise in seismic exploration, posing significant challenges to the quality control raw data. With increasing data volume 3D manual identification methods fall short meeting demands high-density surveys. This study employs YOLOv5 model, widely used tool object detection, achieve rapid airwave profiles. Initially, model was pre-trained on COCO dataset—a large-scale dataset designed for detection—and subsequently fine-tuned using training set specifically labeled The achieved an accuracy and recall rate approximately 85% test dataset, successfully identifying not only presence but also its location, confidence levels, range. To evaluate model’s effectiveness, we applied trained 2D records from two regions: Ningqiang, Shanxi, Xiushui, Sichuan. overall prediction both regions exceeded 90%, with rates surpassing 83% respectively. evaluation time single-shot (over 8000 traces) less than 2 s, highlighting exceptional transferability, generalization ability, efficiency. These results demonstrate that is highly effective detecting across different regions, marking first successful attempt at computer recognition airwaves exploration.
Language: Английский