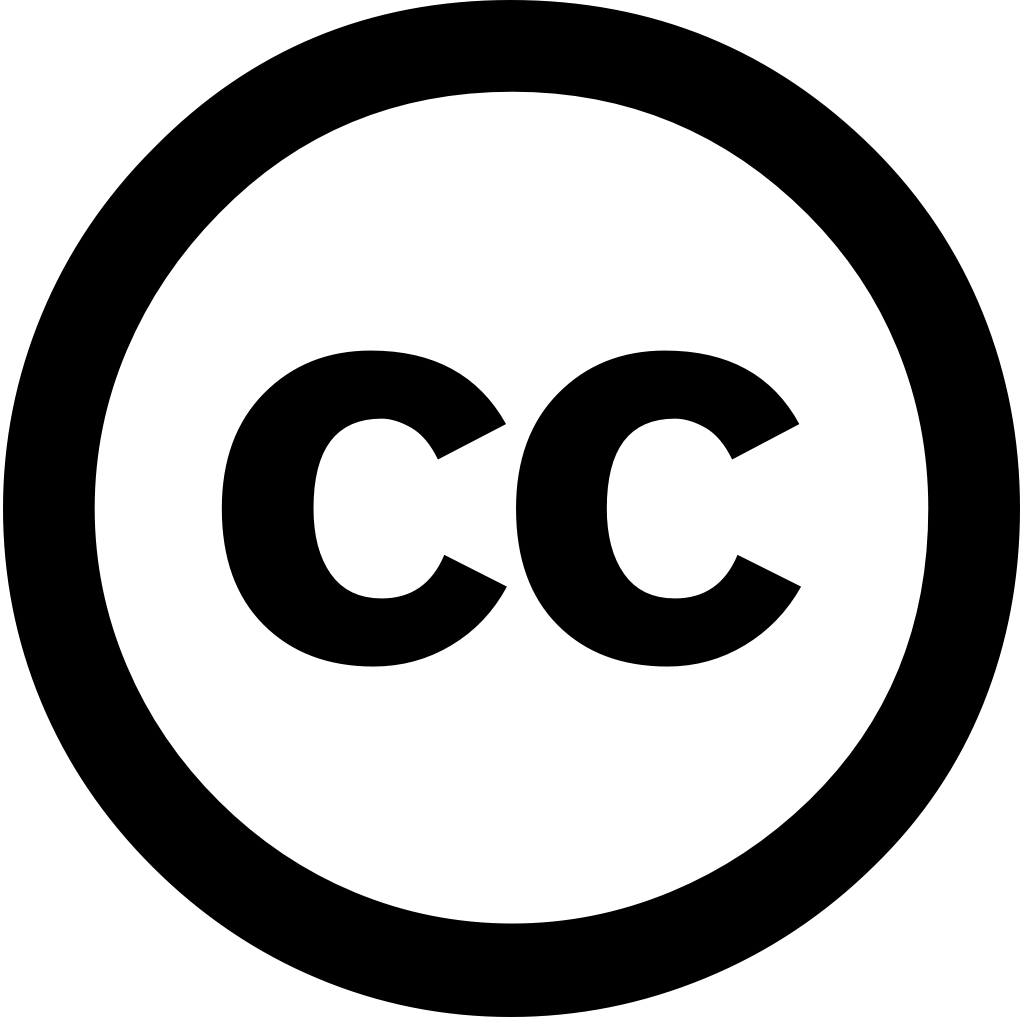
Journal of Cleaner Production, Journal Year: 2024, Volume and Issue: unknown, P. 144300 - 144300
Published: Nov. 1, 2024
Language: Английский
Journal of Cleaner Production, Journal Year: 2024, Volume and Issue: unknown, P. 144300 - 144300
Published: Nov. 1, 2024
Language: Английский
Water, Journal Year: 2025, Volume and Issue: 17(7), P. 1076 - 1076
Published: April 4, 2025
This study proposes an innovative framework integrating geographic information systems (GISs), water quality index (WQI) analysis, and advanced machine learning (ML) models to evaluate the prevalence impact of organic inorganic pollutants across urban–industrial confluence zones (UICZ) surrounding National Capital Territory (NCT) India. Surface samples (n = 118) were systematically collected from Gautam Buddha Nagar, Ghaziabad, Faridabad, Sonipat, Gurugram, Jhajjar, Baghpat districts assess physical, chemical, microbiological parameters. The application spatial interpolation techniques, such as kriging inverse distance weighting (IDW), enhances WQI estimation in unmonitored areas, improving regional assessments remediation planning. GIS mapping highlighted stark disparities, with industrial hubs, like Faridabad exhibiting values exceeding 600 due untreated discharges wastewater, while rural regions, Jhajjar Baghpat, recorded below 200, reflecting minimal anthropogenic pressures. employed four ML models—linear regression (LR), random forest (RF), Gaussian process (GPR_PUK), support vector machines (SVM_Poly)—to predict high precision. SVM_Poly emerged most effective model, achieving testing CC, RMSE, MAE 0.9997, 11.4158, 5.6085, respectively, outperforming RF (0.9925, 29.8107, 21.7398) GPR_PUK (0.9811, 68.4466, 54.0376). By leveraging models, this prediction beyond conventional computation, enabling extrapolation early contamination detection data-scarce regions. Sensitivity analysis identified total suspended solids critical predictor influencing WQI, underscoring its relevance monitoring programs. research uniquely integrates algorithms analytics, providing a novel methodological contribution assessment. findings emphasize urgency mitigating fate transport protect Delhi’s hydrological ecosystems, presenting robust decision-support system for policymakers environmental managers.
Language: Английский
Citations
0Environmental Earth Sciences, Journal Year: 2025, Volume and Issue: 84(8)
Published: April 1, 2025
Language: Английский
Citations
0Journal of Hydrology, Journal Year: 2024, Volume and Issue: unknown, P. 132421 - 132421
Published: Nov. 1, 2024
Language: Английский
Citations
0Journal of Cleaner Production, Journal Year: 2024, Volume and Issue: unknown, P. 144300 - 144300
Published: Nov. 1, 2024
Language: Английский
Citations
0