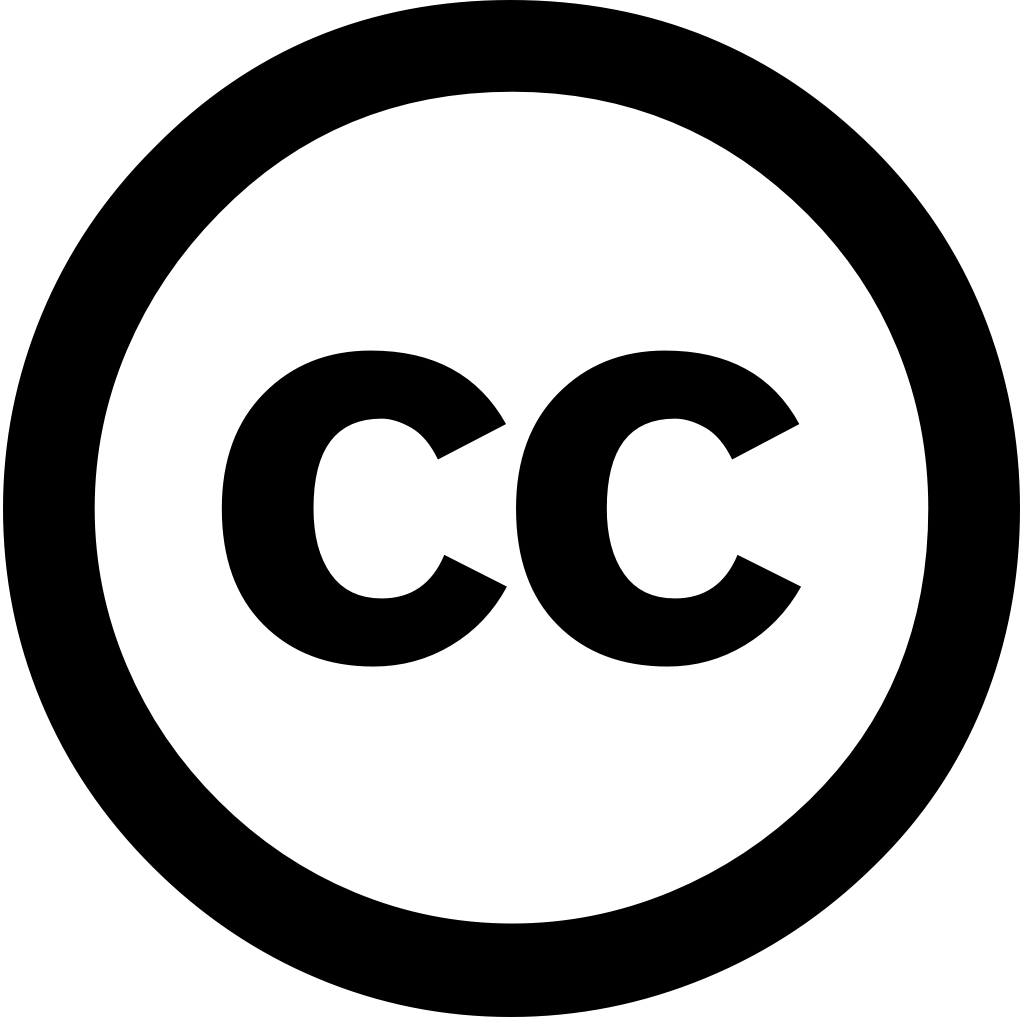
Frontiers in Immunology, Journal Year: 2025, Volume and Issue: 16
Published: March 18, 2025
Breast cancer, a heterogeneous malignancy, comprises multiple subtypes and poses substantial threat to women's health globally. Neoadjuvant therapy (NAT), administered prior surgery, is integral breast cancer treatment strategies. It aims downsize tumors, optimize surgical outcomes, evaluate tumor responsiveness treatment. However, accurately predicting NAT efficacy remains challenging due the disease's complexity diverse responses across different molecular subtypes. In this study, we harnessed multimodal data, including proteomic, genomic, MRI imaging, clinical information, sourced from cohorts such as I-SPY2, TCGA-BRCA, GSE161529, METABRIC. Post data preprocessing, Lasso regression was utilized for feature extraction selection. Five machine learning algorithms were employed construct diagnostic models, with pathological complete response (pCR) predictive endpoint. Our results revealed that multi-omics Ridge model achieved optimal performance in pCR, an AUC of 0.917. Through unsupervised clustering using R package MOVICS nine algorithms, identified four distinct associated NAT. These exhibited significant differences proteomic profiles, hallmark gene sets, pathway activities, immune microenvironments, transcription factor characteristics. For instance, CS1 subtype, predominantly ER-positive, had low pCR rate poor chemotherapy drugs, while CS4 characterized by high infiltration, showed better immunotherapy. At single-cell level, detected heterogeneity microenvironment among Malignant cells displayed copy number variations, differentiation levels, evolutionary trajectories. Cell-cell communication analysis further highlighted differential interaction patterns subtypes, implications progression response. subtype provide novel insights into cancer. findings hold promise guiding personalized Future research should focus on experimental validation, in-depth exploration underlying mechanisms, extension these methods other cancers modalities.
Language: Английский