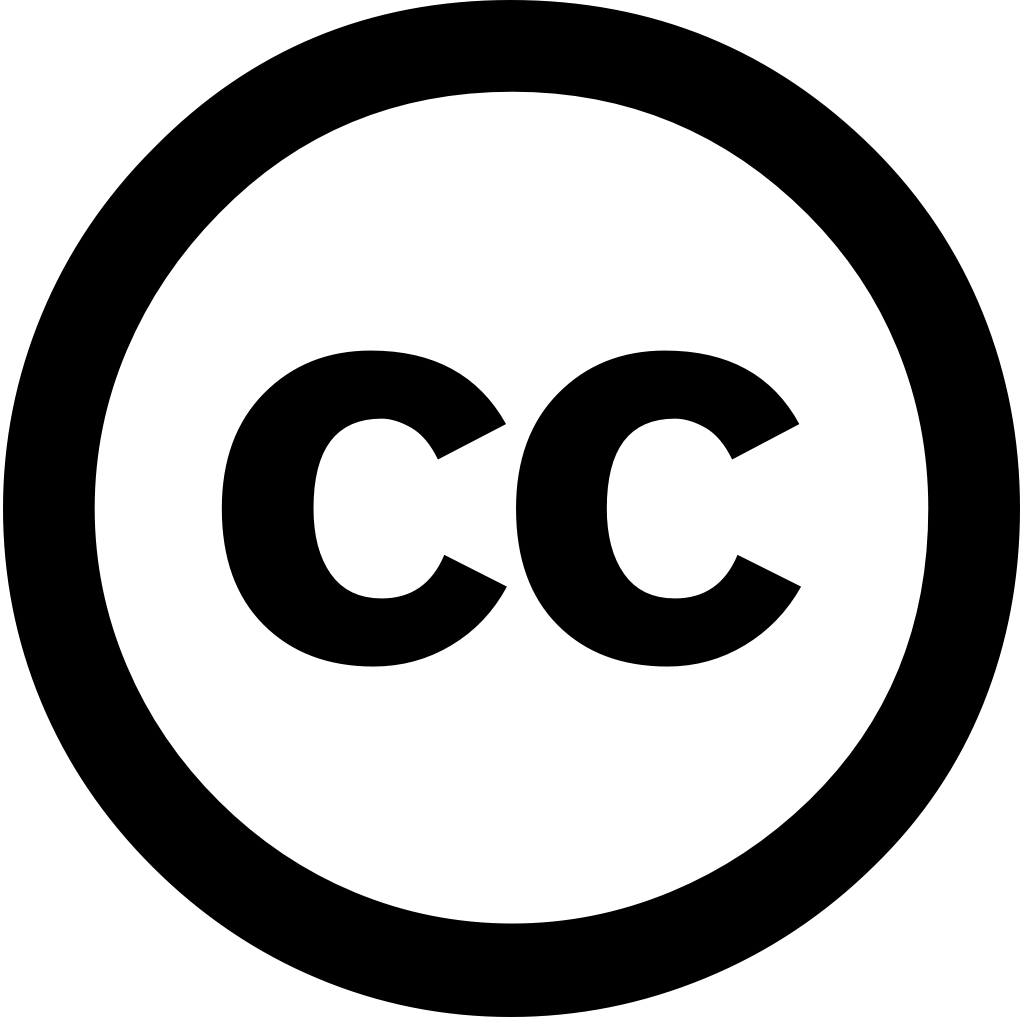
EJNMMI Research, Journal Year: 2024, Volume and Issue: 14(1)
Published: Nov. 13, 2024
A considerable portion of patients with fever unknown origin (FUO) present concomitant lymphadenopathy. Diseases within the spectrum FUO accompanied by lymphadenopathy include lymphoma, infections, and rheumatic diseases. Particularly, lymphoma has emerged as most prevalent etiology associated Distinguishing between benign malignant lymph node lesions is a major challenge for physicians an urgent clinical concern patients. However, conventional imaging techniques, including PET/CT, often have difficulty accurately distinguishing lesions. This study utilizes PET/CT radiomics to differentiate in FUO, aiming improve diagnostic accuracy. Data were collected from 204 who underwent 18F-FDG examinations 114 90 Patients randomly divided into training testing groups at ratio 7:3. total 15 effective features obtained least absolute shrinkage selection operator (LASSO) algorithm. Machine learning models constructed using logistic regression (LR), support vector machine (SVM), random forest (RF), k-nearest neighbors (KNN) algorithms. In group, area under curve (AUC) values predicting cases LR, SVM, RF, KNN 0.936, 0.930, 0.998, 0.938, respectively. There statistically significant differences AUC RF other (all P < 0.001). four 0.860, 0.866, 0.915, 0.891, respectively, no observed among them > 0.05). The decision analysis (DCA) curves model outperformed those three both groups. demonstrated promising performance discriminating showing best performance.
Language: Английский