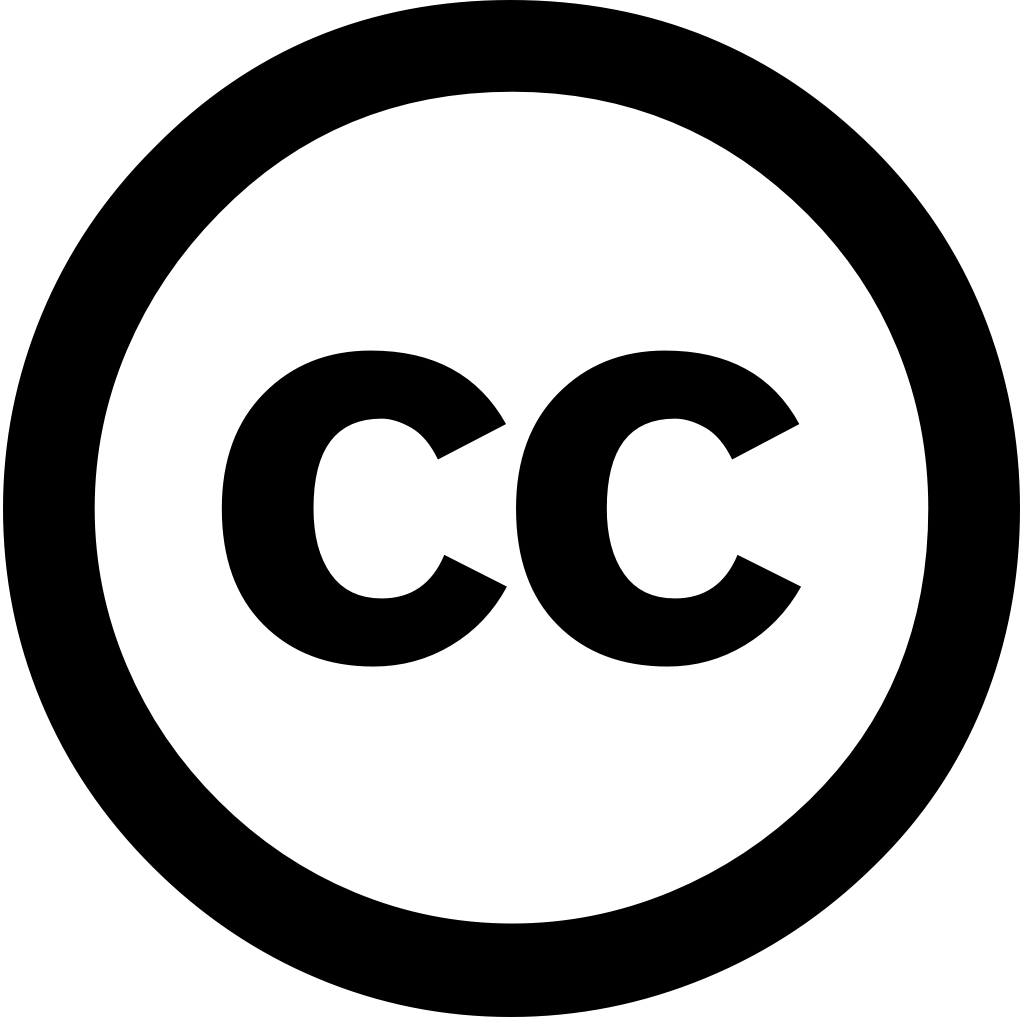
Journal of Translational Medicine, Journal Year: 2025, Volume and Issue: 23(1)
Published: April 5, 2025
Identification of seizures is essential for the treatment epilepsy. Current machine-learning and deep-learning models often perform well on public datasets when classifying generalized with prominent features. However, their performance was less effective in detecting brief, localized seizures. These seizure-like patterns can be masked by fixed brain rhythms. Our study proposes a supervised multilayer hybrid model called GEM-CRAP (gradient-enhanced modulation CNN-RES, attention-like, pre-policy networks), three parallel feature extraction channels: CNN-RES module, an amplitude-aware channel attention-like mechanisms, LSTM-based layer integrated into recurrent neural network. The trained Xuanwu Hospital HUP iEEG dataset, including intracranial, cortical, stereotactic EEG data from 83 patients, covering over 8500 labeled electrode channels classification (wakefulness sleep). A post-SVM network used secondary training accuracy below 80%. We introduced average deviation rate metric to assess seizure detection accuracy. For datasets, achieved 97% intracranial cortical sequences 95% mixed sequences, deviations 5%. In it maintained 94% wakefulness around 90% during sleep. SVM improved 10%. Additionally, strong positive correlation found between distribution temporal states. enhances focal epilepsy through adaptive adjustments attention achieving higher precision robustness complex signal environments. Beyond improving interval detection, excels identifying analyzing specific epileptic waveforms, such as high-frequency oscillations. This advancement may pave way more precise diagnostics provide suitable artificial intelligence algorithm closed-loop neurostimulation.
Language: Английский