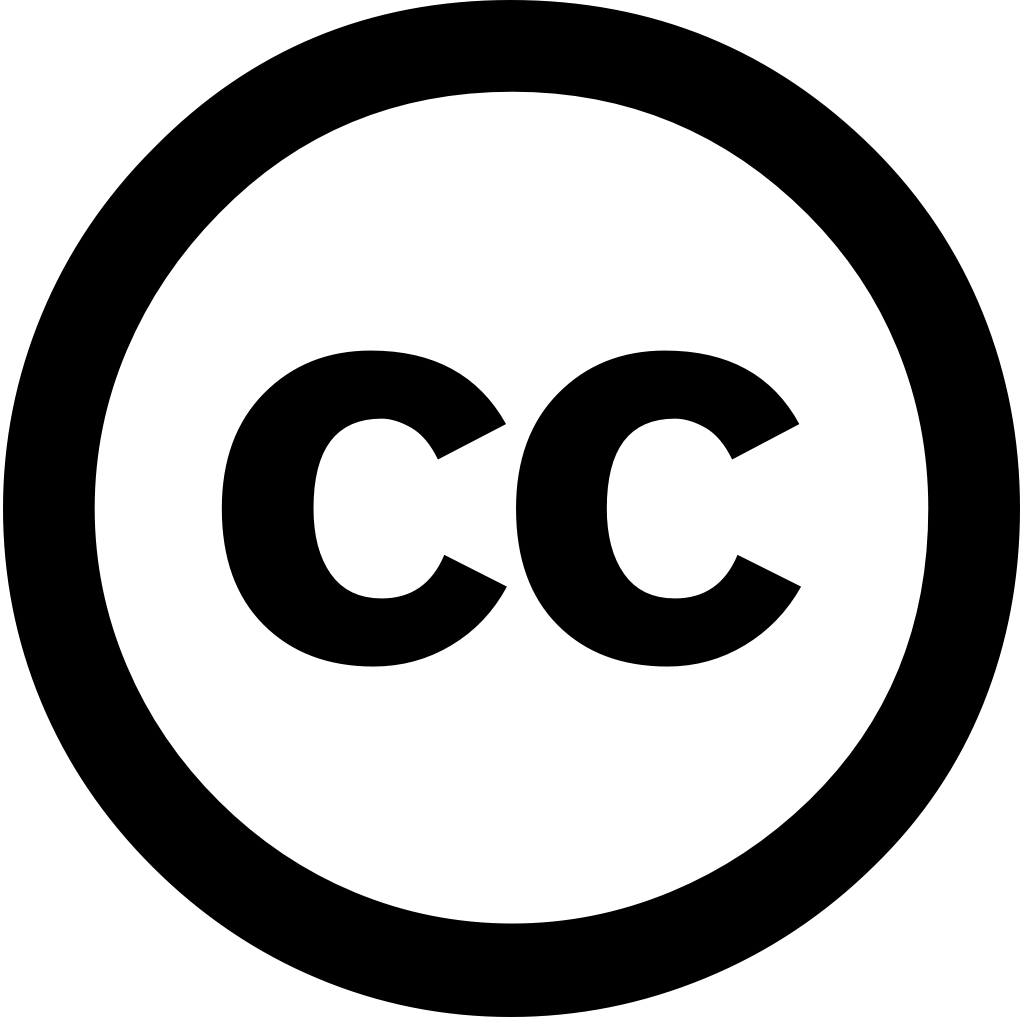
Journal of the Indian Society of Remote Sensing, Journal Year: 2024, Volume and Issue: 52(4), P. 703 - 709
Published: April 1, 2024
Language: Английский
Journal of the Indian Society of Remote Sensing, Journal Year: 2024, Volume and Issue: 52(4), P. 703 - 709
Published: April 1, 2024
Language: Английский
Journal of the Indian Society of Remote Sensing, Journal Year: 2025, Volume and Issue: unknown
Published: Jan. 17, 2025
Language: Английский
Citations
0Research Square (Research Square), Journal Year: 2025, Volume and Issue: unknown
Published: March 17, 2025
Language: Английский
Citations
0Remote Sensing, Journal Year: 2025, Volume and Issue: 17(6), P. 1063 - 1063
Published: March 18, 2025
Aboveground biomass (AGB) is crucial in forest ecosystems and intricately linked to the carbon cycle global climate change dynamics. This study investigates efficacy of synthetic aperture radar (SAR) data from X, C, L bands, combined with Sentinel-2 optical imagery, vegetation indices, gray-level co-occurrence matrix (GLCM) texture metrics, topographical variables estimating AGB Küre Mountains National Park, Türkiye. Four machine-learning regression models were employed: partial least squares (PLS), absolute shrinkage selection operator (LASSO), multivariate linear, ridge regression. Among these, PLS (PLSR) model demonstrated highest accuracy estimation, achieving an R2 0.74, a mean error (MAE) 28.22 t/ha, root square (RMSE) 30.77 t/ha. An analysis across twelve revealed that integrating ALOS-2 PALSAR-2 SAOCOM L-band satellite data, particularly HV HH polarizations significantly enhances precision reliability estimations.
Language: Английский
Citations
0Journal of the Indian Society of Remote Sensing, Journal Year: 2024, Volume and Issue: 52(4), P. 703 - 709
Published: April 1, 2024
Language: Английский
Citations
0