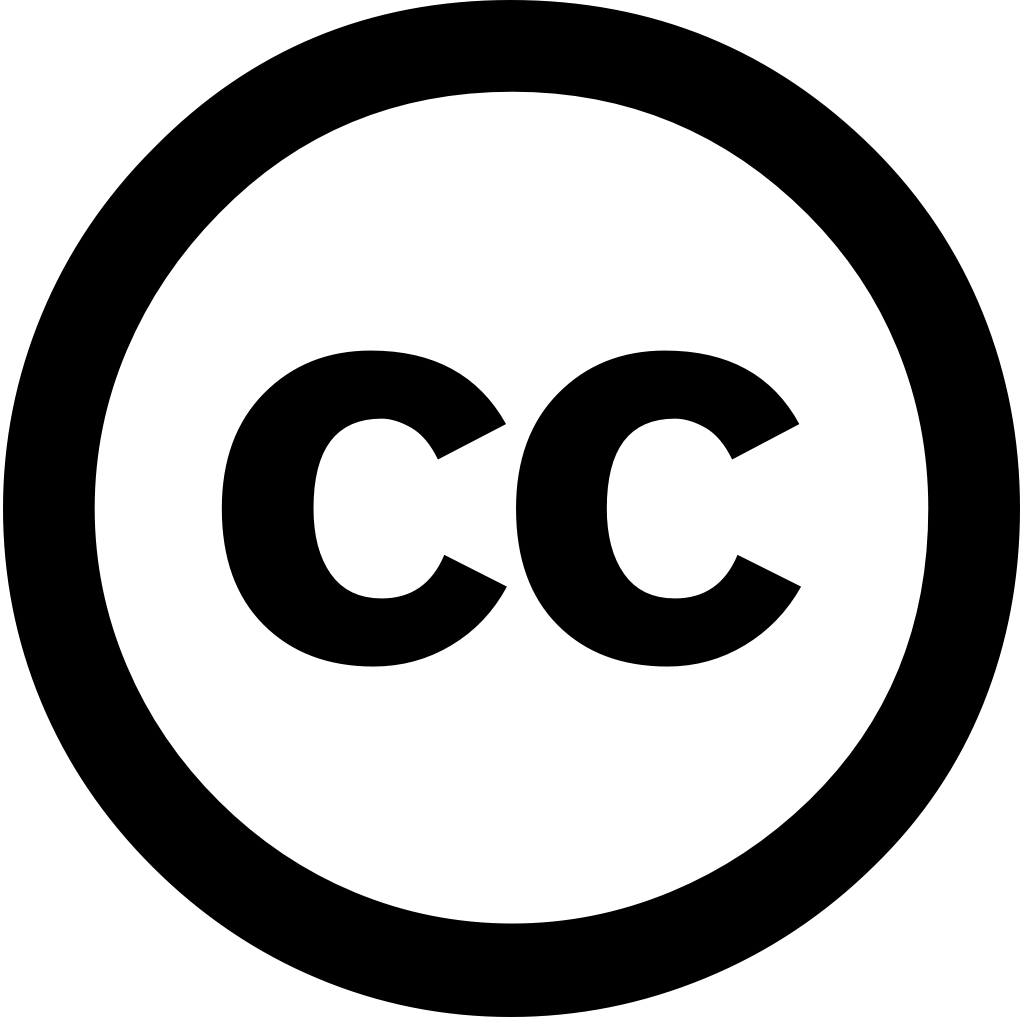
Results in Engineering, Journal Year: 2025, Volume and Issue: unknown, P. 104267 - 104267
Published: Feb. 1, 2025
Language: Английский
Results in Engineering, Journal Year: 2025, Volume and Issue: unknown, P. 104267 - 104267
Published: Feb. 1, 2025
Language: Английский
Energy, Journal Year: 2023, Volume and Issue: 275, P. 127430 - 127430
Published: April 8, 2023
Predicting electricity demand data is considered an essential task in decisions taking, and establishing new infrastructure the power generation network. To deliver a high-quality prediction, this paper proposes hybrid combination technique, based on deep learning model of Convolutional Neural Networks Echo State Networks, named as CESN. Daily from four sites (Roderick, Rocklea, Hemmant Carpendale), located Southeast Queensland, Australia, have been used to develop proposed prediction model. The study also analyzes five other machine learning-based models (support vector regression, multilayer perceptron, extreme gradient boosting, neural network, Light Gradient Boosting) compare evaluate outcomes approach. results obtained experimental showed that able obtain highest performance compared existing developed for daily forecasting. Based statistical approaches utilized study, approach presents accuracy among models. algorithm excellent accurate forecasting method, which outperformed state art algorithms are currently problem.
Language: Английский
Citations
43Energy Strategy Reviews, Journal Year: 2024, Volume and Issue: 54, P. 101446 - 101446
Published: June 4, 2024
In the innovative domain of sustainable and renewable energy, artificial intelligence incorporation has appeared as a critical stimulant for improving productivity, cutting costs, addressing complex difficulties. However, all reported advancement over recent years, their experimental implementations, challenges associated have not been covered by single source. Hence, this review aims to give data source get recent, advanced detailed outlook on applications in energy technologies systems along with examples implementation. More than 150 research reports were retrieved from different bases keywords selection criteria maintain relevance. This specifically explored diverse approaches wide range sources innovations spanning solar power, photovoltaics, microgrid integration, storage power management, wind, geothermal comprehensively. The current technological advances, outcomes, case studies implications are discussed, potential possible solutions. expected advancements trends near future also discussed which can gateway researchers, investigators engineers look resolve already associated.
Language: Английский
Citations
18IEEE Access, Journal Year: 2024, Volume and Issue: 12, P. 90461 - 90485
Published: Jan. 1, 2024
Solar energy is largely dependent on weather conditions, resulting in unpredictable, fluctuating, and unstable photovoltaic (PV) power outputs. Thus, accurate PV forecasts are increasingly crucial for managing controlling integrated systems. Over the years, advanced artificial neural network (ANN) models have been proposed to increase accuracy of various geographical regions. Hence, this paper provides a state-of-the-art review five most popular ANN forecasting. These include multilayer perceptron (MLP), recurrent (RNN), long short-term memory (LSTM), gated unit (GRU), convolutional (CNN). First, internal structure operation these studied. It then followed by brief discussion main factors affecting their forecasting accuracy, including horizons, meteorological evaluation metrics. Next, an in-depth separate analysis standalone hybrid provided. has determined that bidirectional GRU LSTM offer greater whether used as model or configuration. Furthermore, upgraded metaheuristic algorithms demonstrated exceptional performance when applied models. Finally, study discusses limitations shortcomings may influence practical implementation
Language: Английский
Citations
16Applied Sciences, Journal Year: 2023, Volume and Issue: 13(14), P. 8332 - 8332
Published: July 19, 2023
The accuracy of solar energy forecasting is critical for power system planning, management, and operation in the global electric grid. Therefore, it crucial to ensure a constant sustainable supply consumers. However, existing statistical machine learning algorithms are not reliable due sporadic nature data. Several factors influence performance irradiance, such as horizon, weather classification, evaluation metrics. we provide review paper on deep learning-based irradiance models. These models include Long Short-Term Memory (LTSM), Gated Recurrent Unit (GRU), Neural Network (RNN), Convolutional (CNN), Generative Adversarial Networks (GAN), Attention Mechanism (AM), other hybrid Based our analysis, perform better than conventional applications, especially combination with some techniques that enhance extraction features. Furthermore, use data augmentation improve useful, networks. Thus, this expected baseline analysis future researchers select most appropriate approaches photovoltaic forecasting, wind electricity consumption medium term long term.
Language: Английский
Citations
31Applied Energy, Journal Year: 2023, Volume and Issue: 353, P. 122059 - 122059
Published: Oct. 18, 2023
Prediction of electricity price is crucial for national markets supporting sale prices, bidding strategies, dispatch, control and market volatility management. High volatility, non-stationarity multi-seasonality prices make it significantly challenging to estimate its future trend, especially over near real-time forecast horizons. An error compensation strategy that integrates Long Short-Term Memory (LSTM) network, Convolution Neural Network (CNN) the Variational Mode Decomposition (VMD) algorithm proposed predict half-hourly step prices. A prediction model incorporating VMD CLSTM first used obtain an initial prediction. To improve predictive accuracy, a novel framework, which built using Random Forest Regression (RF) algorithm, also used. The VMD-CLSTM-VMD-ERCRF evaluated from Queensland, Australia. results reveal highly accurate performance all datasets considered, including winter, autumn, spring, summer, yearly predictions. As compared with without (i.e., VMD-CLSTM model), outperforms benchmark models. For predictions, average Legates McCabe Index seen increase by 15.97%, 16.31%, 20.23%, 10.24%, 14.03%, respectively, relative According tests performed on independent datasets, can be practical stratagem useful short-term, forecasting. Therefore research outcomes demonstrate framework effective decision-support tool improving accuracy price. It could value energy companies, policymakers operators develop their insight analysis, distribution optimization strategies.
Language: Английский
Citations
25Water Resources Management, Journal Year: 2023, Volume and Issue: 37(9), P. 3631 - 3655
Published: May 8, 2023
Language: Английский
Citations
23Engineering Applications of Artificial Intelligence, Journal Year: 2024, Volume and Issue: 132, P. 107918 - 107918
Published: Feb. 3, 2024
Language: Английский
Citations
16Energy, Journal Year: 2024, Volume and Issue: 291, P. 129938 - 129938
Published: Jan. 3, 2024
Language: Английский
Citations
12The Journal of Supercomputing, Journal Year: 2025, Volume and Issue: 81(2)
Published: Jan. 14, 2025
Language: Английский
Citations
1Energy Conversion and Management, Journal Year: 2023, Volume and Issue: 297, P. 117707 - 117707
Published: Oct. 5, 2023
Predicting electricity demand (G) is crucial for grid operation and management. In order to make reliable predictions, model inputs must be analyzed predictive features before they can incorporated into a forecast model. this study, hybrid multi-algorithm framework developed by incorporating Artificial Neural Networks (ANN), Encoder-Decoder Based Long Short-Term Memory (EDLSTM) Improved Complete Ensemble Empirical Mode Decomposition with Adaptive Noise (ICMD). Following the partitioning of data, G time-series are decomposed multiple using ICEEMDAN algorithm, partial autocorrelation applied training sets determine lagged features. We combine where components highest frequency predicted an ANN model, while remaining EDLSTM To generate results, all IMF components' predictions merged ICMD-ANN-EDLSTM models. A comparison made between objective standalone models (ANN, RFR, LSTM), (CLSTM), three decomposition-based on Relative Mean Absolute Error at Duffield Road substation was ≈2.82%, ≈4.15%, ≈3.17%, ≈6.41%, ≈6.60%, ≈6.49%, ≈6.602%, compared ICMD-RFR-LSTM, ICMD-RFR-CLSTM, LSTM, CLSTM, ANN. According statistical score metrics, performed better than other benchmark Further, results show that not only detect seasonality in but also predict analyze market add valuable insight analysis.
Language: Английский
Citations
20