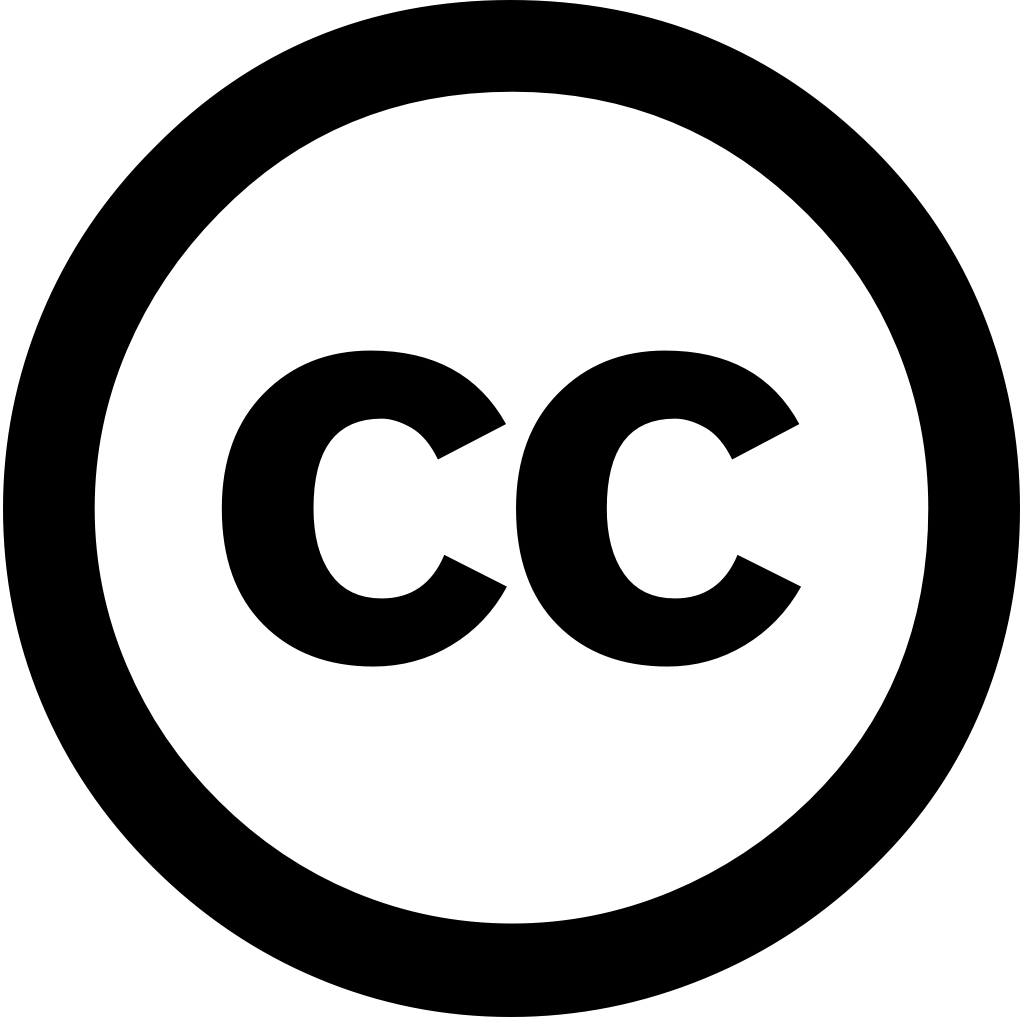
Sustainable Energy Technologies and Assessments, Journal Year: 2021, Volume and Issue: 44, P. 101032 - 101032
Published: Feb. 7, 2021
Language: Английский
Sustainable Energy Technologies and Assessments, Journal Year: 2021, Volume and Issue: 44, P. 101032 - 101032
Published: Feb. 7, 2021
Language: Английский
ACM Computing Surveys, Journal Year: 2023, Volume and Issue: 56(1), P. 1 - 41
Published: June 9, 2023
Today, cloud computation offloading may not be an appropriate solution for delay-sensitive applications due to the long distance between end-devices and remote datacenters. In addition, a can consume bandwidth dramatically increase costs. However, such as sensors, cameras, smartphones have limited computing storage capacity. Processing tasks on battery-powered energy-constrained devices becomes even more complex. To address these challenges, new paradigm called Edge Computing (EC) emerged nearly decade ago bring resources closer end-devices. Here, edge servers located end-device perform user tasks. Recently, several paradigms Mobile (MEC) Fog (FC) complement Cloud (CC) EC. Although are heterogeneous, they further reduce energy consumption task response time, especially applications. Computation is multi-objective, NP-hard optimization problem. A significant part of previous research in this field devoted Machine Learning (ML) methods. One essential types ML Reinforcement (RL), which agent learns how make best decision using experiences gained from environment. This article provides systematic review widely used RL approaches offloading. It covers complementary mobile computing, fog Internet Things. We explain reasons various methods technical point view. analysis includes both binary partial techniques. For each method, elements characteristics environment discussed regarding most important criteria. Research challenges Future trends also mentioned.
Language: Английский
Citations
52IEEE Access, Journal Year: 2024, Volume and Issue: 12, P. 14334 - 14349
Published: Jan. 1, 2024
With
the
voluminous
information
being
produced
by
Internet
of
Things
(IoT)
smart
gadgets,
consumers
with
their
countless
service
requests
are
also
growing
rapidly.
As
there
is
a
huge
distance
between
IoT
devices
and
Cloud
datacenter,
some
latency
incurred
in
communication
datacenter.
This
can
be
reduced
introducing
Fog
layer
therefore,
it
paramount
to
offload
those
tremendous
data
leverage
overloaded
storage
computation
Cloud-based
systems
Fog-assisted
nodes.
Moreover,
these
heavy
computations
consume
significant
energy
from
distributed
servers
as
well
datacenters.
Therefore,
this
work
addresses
task
migration
problem
Fog-Cloud
system
load
balancing
reduce
rate,
utilized
time
while
increasing
resource
utilization
for
latency-sensitive
systems.
paper
uses
Fuzzy
logic
algorithm
determining
target
layers
offloading
considering
heterogeneity
requirements
(
Language: Английский
Citations
17Journal of Ambient Intelligence and Humanized Computing, Journal Year: 2020, Volume and Issue: 12(8), P. 8265 - 8284
Published: Sept. 25, 2020
Language: Английский
Citations
82Peer-to-Peer Networking and Applications, Journal Year: 2021, Volume and Issue: 14(3), P. 1334 - 1347
Published: March 3, 2021
Language: Английский
Citations
64IEEE Transactions on Consumer Electronics, Journal Year: 2024, Volume and Issue: 70(1), P. 3848 - 3855
Published: Feb. 1, 2024
This paper introduces an innovative framework at the convergence of Artificial Intelligence (AI), Multi-objective Optimization (MOO), and Internet Things (IoT), specifically tailored for applications in consumer electronics within smart cities. The seeks to revolutionize urban living by offering intelligent, responsive, interconnected solutions. In advancing evolution cities towards enhanced sharing interconnectedness, this scrutinizes city data technology grounded (IoT) cloud computing (CC) approaches. Employing machine learning methodologies, particularly Random Forest (RF) algorithm, facilitates autonomous communication between machines devoid human intervention. To solve multi-criteria problem, a hybrid algorithm is proposed, emulating behavioral traits Spotted Hyena (SHO) Emperor Penguin (EPO) algorithms. Experimental results underscore superior efficiency proposed optimization comparison with currently employed techniques.
Language: Английский
Citations
9Cluster Computing, Journal Year: 2022, Volume and Issue: 25(2), P. 1393 - 1420
Published: Jan. 18, 2022
Language: Английский
Citations
32Soft Computing, Journal Year: 2022, Volume and Issue: 26(18), P. 9287 - 9322
Published: June 30, 2022
Language: Английский
Citations
29Cluster Computing, Journal Year: 2023, Volume and Issue: 26(5), P. 3113 - 3144
Published: July 21, 2023
Language: Английский
Citations
17Cluster Computing, Journal Year: 2024, Volume and Issue: 27(8), P. 10299 - 10324
Published: May 17, 2024
Language: Английский
Citations
8Soft Computing, Journal Year: 2022, Volume and Issue: 27(7), P. 3817 - 3828
Published: Oct. 18, 2022
Language: Английский
Citations
23