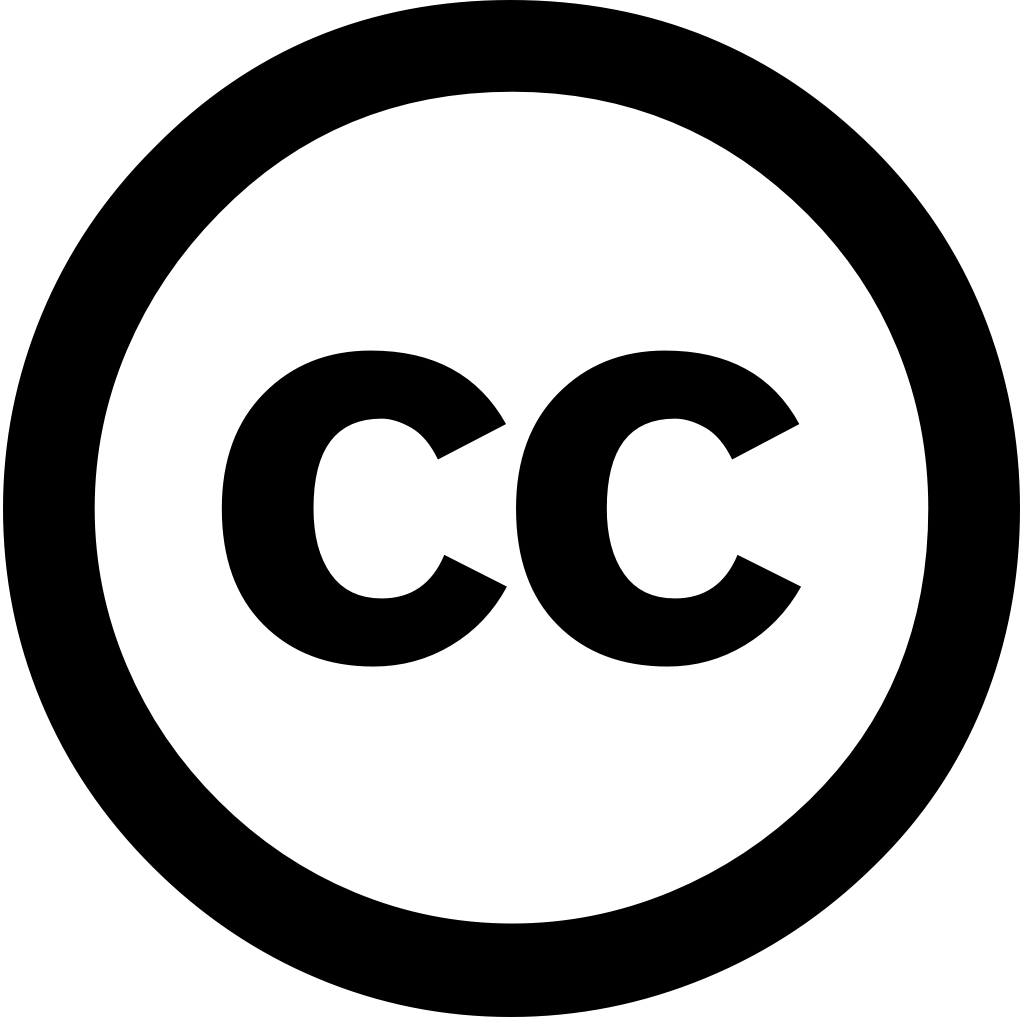
Heritage Science, Journal Year: 2024, Volume and Issue: 12(1)
Published: Sept. 6, 2024
Language: Английский
Heritage Science, Journal Year: 2024, Volume and Issue: 12(1)
Published: Sept. 6, 2024
Language: Английский
Molecular Informatics, Journal Year: 2025, Volume and Issue: 44(1)
Published: Jan. 1, 2025
Abstract In recent times, graph representation learning has been becoming a hot research topic which attracted lot of attention from researchers. Graph embeddings have diverse applications across fields such as information and social network analysis, bioinformatics cheminformatics, natural language processing (NLP), recommendation systems. Among the advanced deep (DL) based architectures used in learning, neural networks (GNNs) emerged dominant highly effective framework. The GNN‐based methods demonstrated state‐of‐the‐art performance on complex supervised unsupervised tasks at both node levels. years, to enhance multi‐view structured representations, contrastive learning‐based techniques developed, introducing models known (GCL) models. These GCL approaches leverage capture representations by comparing embeddings, yielding significant improvements graph‐level task‐specific applications, molecular embedding classification. However, most are primarily designed focus explicit structure through encoders, they often overlook critical topological insights that could be provided data analysis (TDA). Given promising indicating features can greatly benefit various tasks, we propose novel topology‐enhanced, model called TMGCL. Our TMGCL is utilize comprehensive multi‐scale global structural graphs. This enhanced capability positions directly support range classification, with improved accuracy robustness. Extensive experiments within two real‐world datasets proved effectiveness outperformance our proposed GNN/GCL‐based baselines.
Language: Английский
Citations
1Molecular Informatics, Journal Year: 2025, Volume and Issue: 44(3)
Published: March 1, 2025
Abstract Within a recent decade, graph neural network (GNN) has emerged as powerful architecture for various graph‐structured data modelling and task‐driven representation learning problems. Recent studies have highlighted the remarkable capabilities of GNNs in handling complex tasks, achieving state‐of‐the‐art results node/graph classification, regression, generation. However, most traditional GNN‐based architectures like GCN GraphSAGE still faced several challenges related to capability preserving multi‐scaled topological structures. These models primarily focus on capturing local neighborhood information, often failing retain global structural features essential graph‐level classification tasks. Furthermore, their expressiveness is limited when structures molecular datasets. To overcome these limitations, this paper, we proposed novel which an integration between neuro‐ f uzzy t o p ological g raph approach, naming as: FTPG. Specifically, within our FTPG model, introduce approach property prediction by integrating with advanced components. The employs separate modules effectively capture both graph‐based well features. Moreover, further address feature uncertainty global‐view representation, multi‐layered neuro‐fuzzy incorporated model enhance robustness learned embeddings. This combinatorial can assist leverage strengths multi‐view multi‐modal learning, enabling deliver superior performance Extensive experiments real‐world/benchmark datasets demonstrate effectiveness model. It consistently outperforms baselines categorized different approaches, including canonical proximity message passing based, transformer‐based, topology‐driven approaches.
Language: Английский
Citations
0Heritage Science, Journal Year: 2024, Volume and Issue: 12(1)
Published: Sept. 6, 2024
Language: Английский
Citations
3