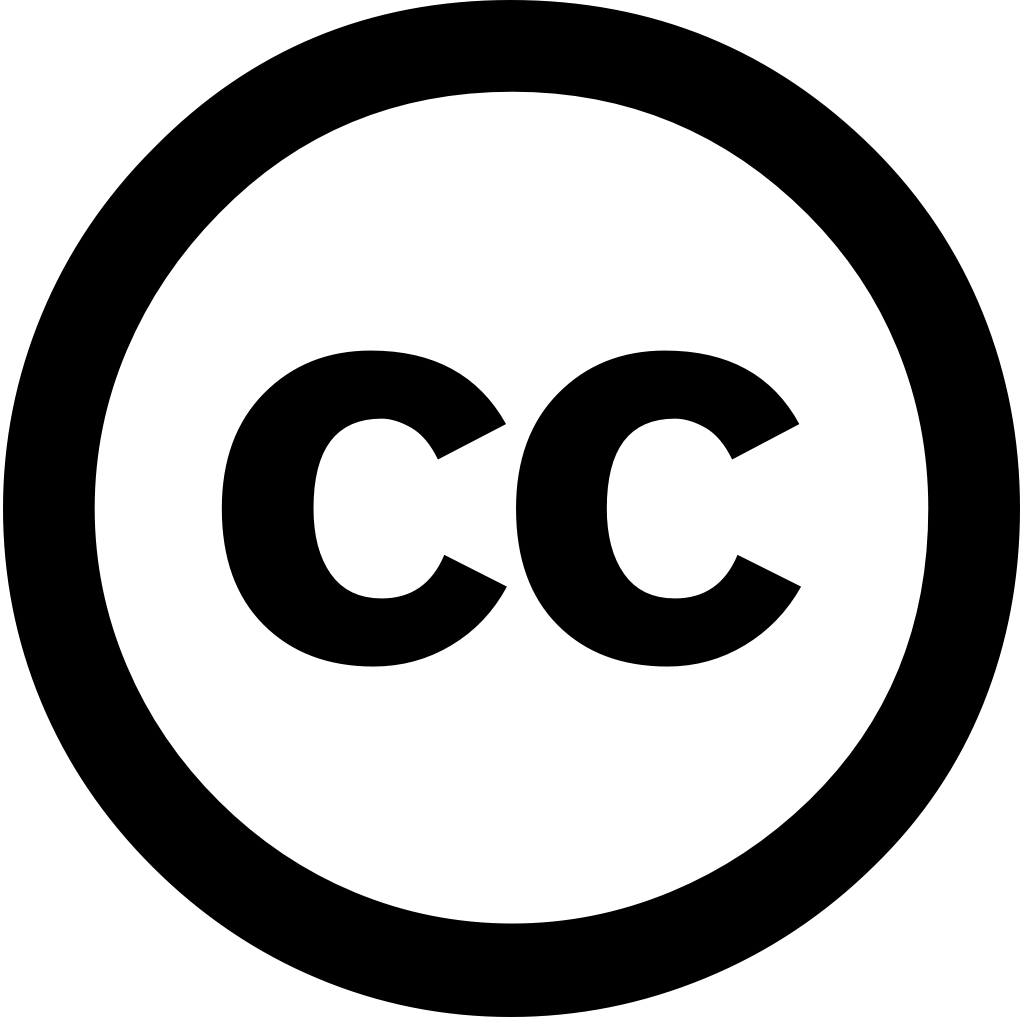
Expert Systems with Applications, Journal Year: 2025, Volume and Issue: unknown, P. 127939 - 127939
Published: May 1, 2025
Language: Английский
Expert Systems with Applications, Journal Year: 2025, Volume and Issue: unknown, P. 127939 - 127939
Published: May 1, 2025
Language: Английский
Mathematics, Journal Year: 2025, Volume and Issue: 13(5), P. 887 - 887
Published: March 6, 2025
DeepFake detection models play a crucial role in ambient intelligence and smart environments, where systems rely on authentic information for accurate decisions. These integrating interconnected IoT devices AI-driven systems, face significant threats from DeepFakes, potentially leading to compromised trust, erroneous decisions, security breaches. To mitigate these risks, neural-network-based have been developed. However, their substantial computational requirements long training times hinder deployment resource-constrained edge devices. This paper investigates compression transfer learning techniques reduce the demands of deploying models, while preserving performance. Pruning, knowledge distillation, quantization, adapter modules are explored enable efficient real-time detection. An evaluation was conducted four benchmark datasets: “SynthBuster”, “140k Real Fake Faces”, “DeepFake Images”, “ForenSynths”. It compared compressed with uncompressed baselines using widely recognized metrics such as accuracy, precision, recall, F1-score, model size, time. The results showed that at 10% original size retained only 56% baseline but fine-tuning similar scenarios increased this nearly 98%. In some cases, accuracy even surpassed original’s performance by up 12%. findings highlight feasibility computing scenarios.
Language: Английский
Citations
0Published: March 25, 2025
AInsectID Version 1.1 is a Graphical User Interface (GUI)‐operable open‐source insect species identification, color processing, and image analysis software. The software has current database of 150 insects integrates artificial intelligence approaches to streamline the process with focus on addressing prediction challenges posed by mimics. This paper presents methods algorithmic development, coupled rigorous machine training used enable high levels validation accuracy. Our work transfer learning prominent convolutional neural network (CNN) architectures, including VGG16, GoogLeNet, InceptionV3, MobileNetV2, ResNet50, ResNet101. Here, we employ both fine tuning hyperparameter optimization improve performance. After extensive computational experimentation, ResNet101 evidenced as being most effective CNN model, achieving accuracy 99.65%. dataset utilized for sourced from National Museum Scotland, Natural History London, open source datasets Zenodo (CERN's Data Center), ensuring diverse comprehensive collection species.
Language: Английский
Citations
0Automation in Construction, Journal Year: 2025, Volume and Issue: 175, P. 106238 - 106238
Published: May 1, 2025
Language: Английский
Citations
0Expert Systems with Applications, Journal Year: 2025, Volume and Issue: unknown, P. 127939 - 127939
Published: May 1, 2025
Language: Английский
Citations
0