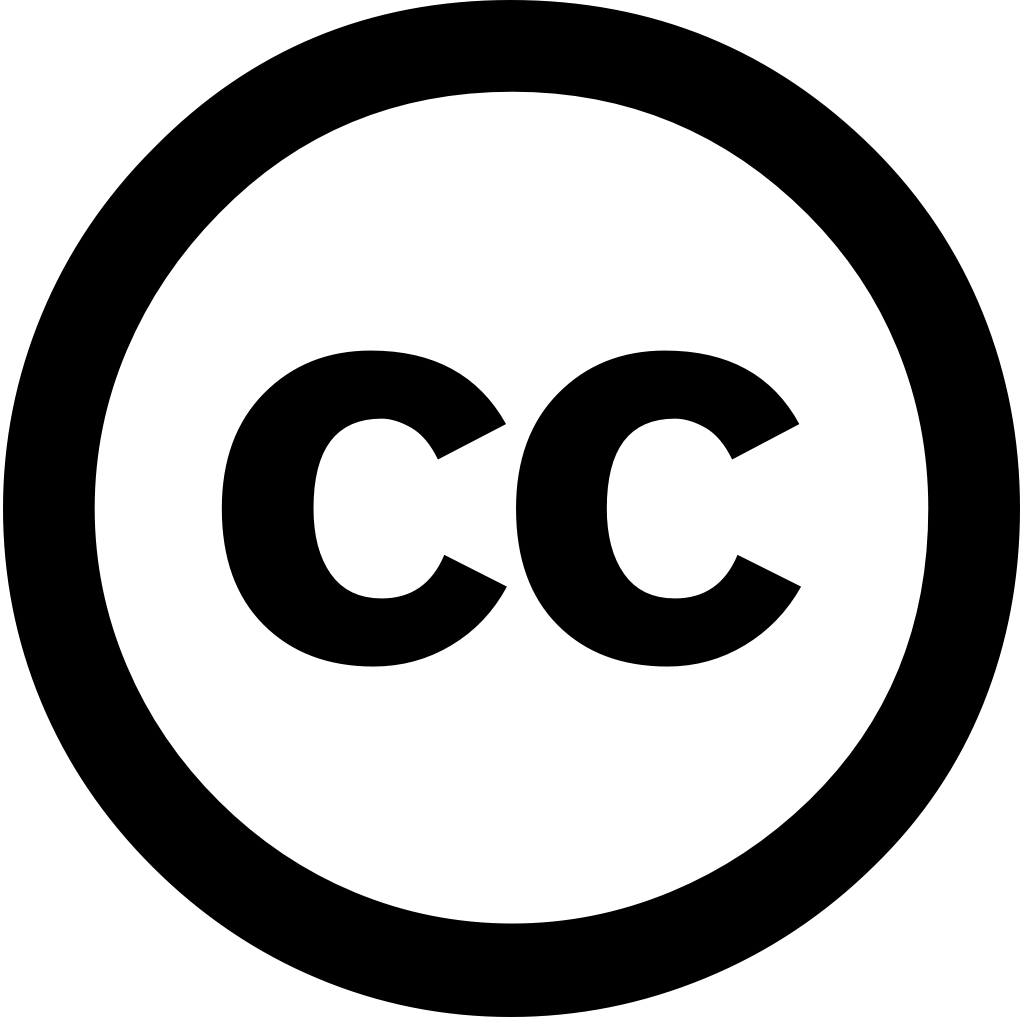
Geomechanics and Geophysics for Geo-Energy and Geo-Resources, Journal Year: 2024, Volume and Issue: 10(1)
Published: Dec. 1, 2024
Language: Английский
Geomechanics and Geophysics for Geo-Energy and Geo-Resources, Journal Year: 2024, Volume and Issue: 10(1)
Published: Dec. 1, 2024
Language: Английский
Physics and Chemistry of the Earth Parts A/B/C, Journal Year: 2025, Volume and Issue: unknown, P. 103885 - 103885
Published: Feb. 1, 2025
Language: Английский
Citations
0ABUAD Journal of Engineering Research and Development (AJERD), Journal Year: 2025, Volume and Issue: 8(1), P. 292 - 306
Published: April 24, 2025
Sand production is one of the major challenges in oil and gas industry, impacting operational integrity economic efficiency extraction activities. This study focuses on predicting Reservoir Flow Capacity (RFC) sandstone formations by analyzing geological petrophysical properties critical to reservoir performance mechanical stability. It also identified key factors that impact stability during production. Given a large number input variables enclose environmental factors, set correlation these conditions provide profound analysis reveal patterns within data. With following supervised machine learning algorithms: Random Forest, Artificial Neural Network (ANN) Support Vector Regression (SVR); modeled RFC. The algorithms were selected for their ability model complex relationships characterization, with Forest excelling high-dimensional data handling, ANN pattern learning, SVR regression-based predictions. Model evaluation using R-Squared metrics showed possesses good level accuracy 0.9573 RFC, compared which had values 0.9390 0.7294 respectively. variations from actual hence was not very useful our Further developed models revealed formation thickness, permeability are most parameters influencing flow capacity overall rock
Language: Английский
Citations
0Geoenergy Science and Engineering, Journal Year: 2025, Volume and Issue: unknown, P. 213954 - 213954
Published: May 1, 2025
Language: Английский
Citations
0Journal of Rock Mechanics and Geotechnical Engineering, Journal Year: 2025, Volume and Issue: unknown
Published: May 1, 2025
Language: Английский
Citations
0Geomechanics and Geophysics for Geo-Energy and Geo-Resources, Journal Year: 2024, Volume and Issue: 10(1)
Published: Dec. 1, 2024
Language: Английский
Citations
1