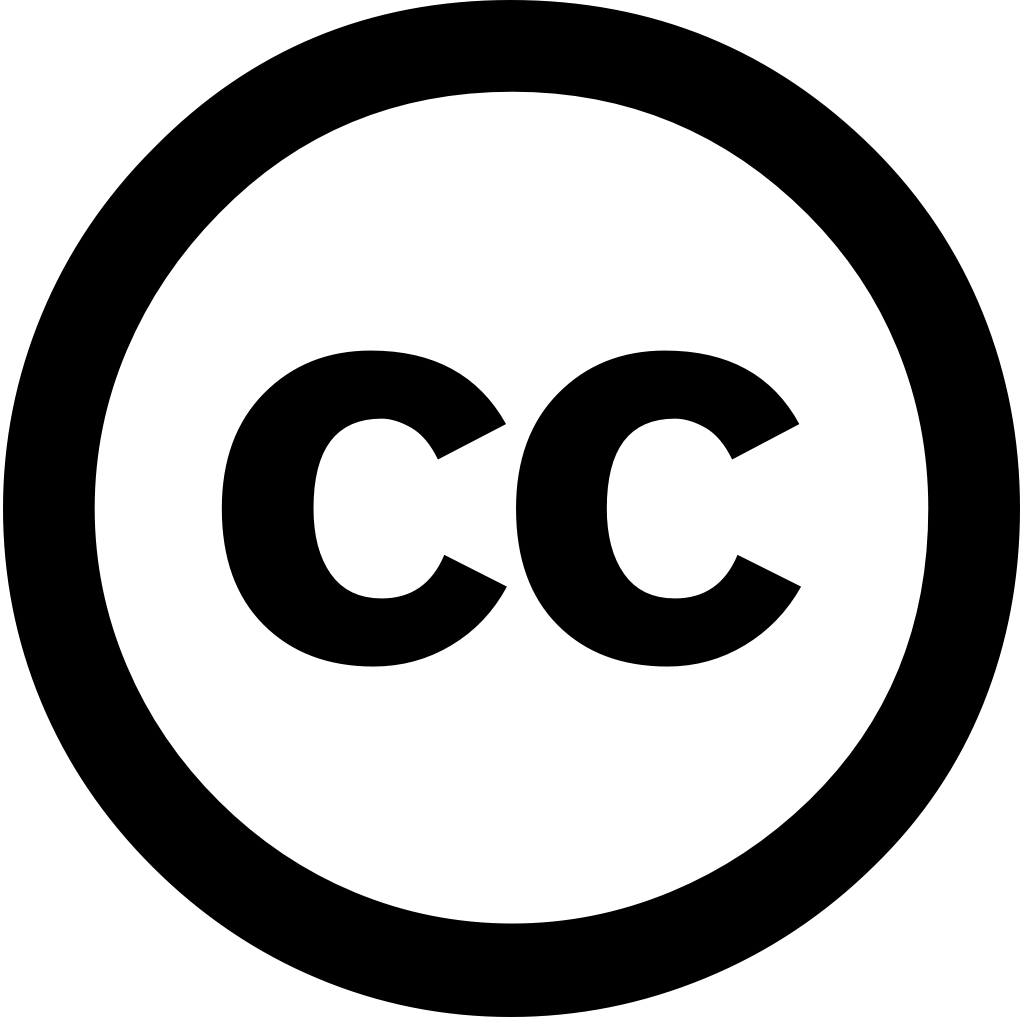
Sensors, Journal Year: 2025, Volume and Issue: 25(8), P. 2487 - 2487
Published: April 15, 2025
With the rapid emergence of Internet Things (IoT) devices, there were new vectors for attacking cyber, so was a need approachable intrusion detection systems (IDSs) with more innovative custom tactics. The traditional IDS models tend to find difficulties in generalization continuously changing and heterogeneous IoT environments. This paper contributes an adaptive framework using Model-Agnostic Meta-Learning (MAML) few-shot learning paradigms quickly adapt tasks little data. goal this research is improve security by developing strong that will perform well across assorted datasets attack Finally, we apply our proposed two benchmark datasets, UNSW-NB15 NSL-KDD99, which provide different scenarios network behaviors. methodology trains base model MAML allow fast adaptation on specific during fine-tuning. Our approach leads experimental results 99.98% accuracy, 99.5% precision, 99.0% recall, 99.4% F1 score dataset. achieved 99.1% 97.3% 98.2% 98.5% NSL-KDD99 That shows can detect many cyber threats Based study, it concluded meta-learning-based could help build resilient systems. Future works move educated meta-learning federated setting deploy real time response threats.
Language: Английский