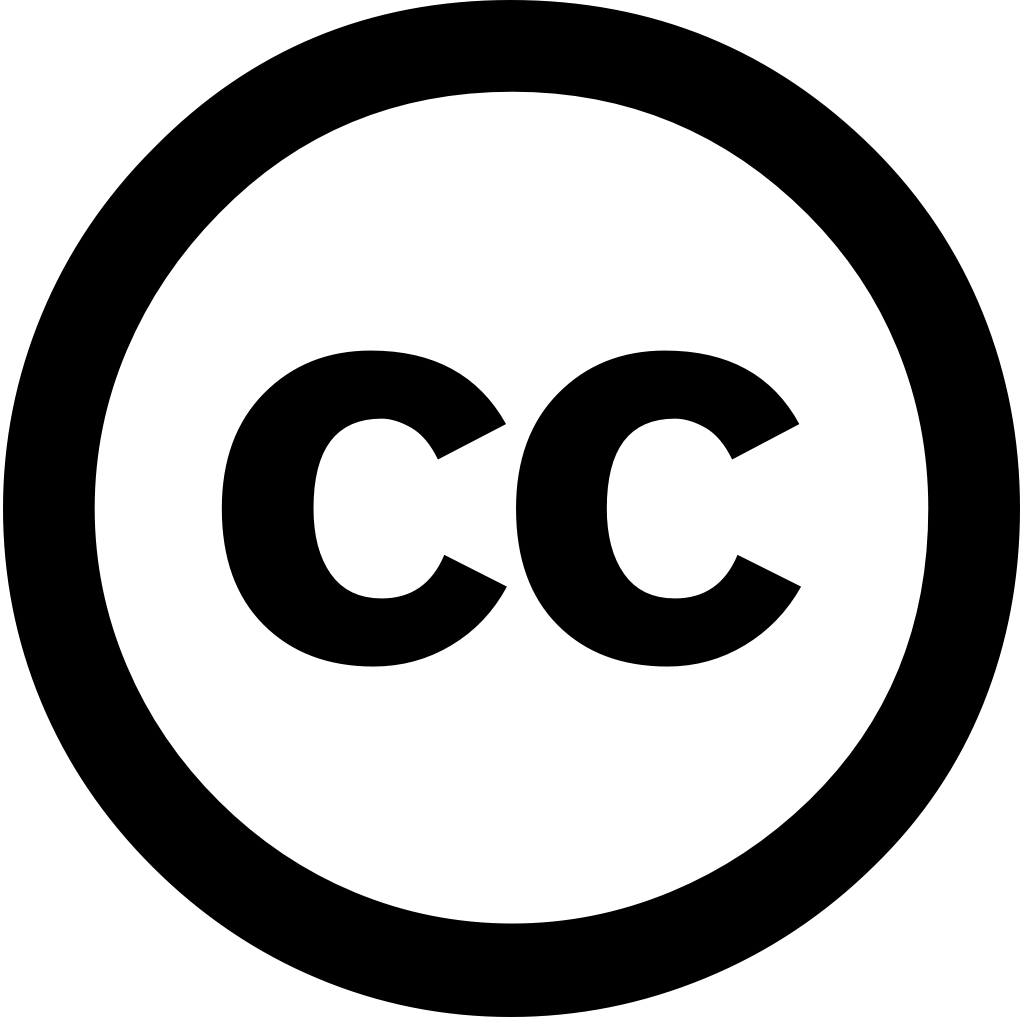
Research Square (Research Square), Journal Year: 2023, Volume and Issue: unknown
Published: Sept. 18, 2023
Language: Английский
Research Square (Research Square), Journal Year: 2023, Volume and Issue: unknown
Published: Sept. 18, 2023
Language: Английский
Indian Journal of Microbiology, Journal Year: 2024, Volume and Issue: unknown
Published: April 10, 2024
Language: Английский
Citations
5International Journal of Computational Intelligence Systems, Journal Year: 2024, Volume and Issue: 17(1)
Published: May 16, 2024
Abstract Autism spectrum disorder (ASD) is a complex developmental issue that affects the behavior and communication abilities of children. It extremely needed to perceive it at an early age. The research article focuses on attentiveness by considering eye positioning as key feature its implementation completed in two phases. In first phase, various transfer learning algorithms are implemented evaluated predict ASD traits available open-source image datasets Kaggle Zenodo. To reinforce result, fivefold cross-validation used dataset. Progressive pre-trained named VGG 16, 19, InceptionV3, ResNet152V2, DenseNet201, ConNextBase, EfficientNetB1, NasNetMobile, InceptionResNEtV2 establish correctness result. result being compiled analyzed ConvNextBase model has best diagnosing ability both datasets. This achieved prediction accuracy 80.4% with batch size rate 0.00002, 10 epochs 6 units, 80.71% Zenodo dataset 4, 4 units. found challenging nature compared existing model. Attentiveness parameter will accurately diagnose visual participant which helps automatic autistic traits. second phase proposed model, engrossed identifying uses dlib library HOG Linear SVM-based face detectors identify particular facial called EAR measure participants' based gaze analysis. If value less than 0.20 for more 100 consecutive frames, concludes un-attentive. generated special graph time period continuously plotting attention level. average depict participant.
Language: Английский
Citations
5Lecture notes in networks and systems, Journal Year: 2025, Volume and Issue: unknown, P. 577 - 586
Published: Jan. 1, 2025
Language: Английский
Citations
0AIP conference proceedings, Journal Year: 2025, Volume and Issue: 3308, P. 030001 - 030001
Published: Jan. 1, 2025
Language: Английский
Citations
0Franklin Open, Journal Year: 2025, Volume and Issue: unknown, P. 100275 - 100275
Published: May 1, 2025
Language: Английский
Citations
0Bioengineering & Translational Medicine, Journal Year: 2024, Volume and Issue: 9(6)
Published: June 28, 2024
Abstract Engineered stone silicosis (ESS), primarily caused by inhaling respirable crystalline silica, poses a significant occupational health risk globally. ESS has no effective treatment and presents rapid progression from simple (SS) to progressive massive fibrosis (PMF), with respiratory failure death. Despite the use of diagnostic methods like chest x‐rays high‐resolution computed tomography, early detection remains challenging. Since routine blood tests have shown promise in detecting inflammatory markers associated disease, this study aims assess whether biomarkers, coupled machine learning techniques, can effectively differentiate between healthy individuals, subjects SS, PMF. To end, 107 men diagnosed silicosis, ex‐workers engineered (ES) sector, 22 male volunteers as controls not exposed ES dust were recruited. Twenty‐one primary biochemical derived peripheral extraction obtained retrospectively clinical hospital records. Relief‐ F features selection technique was applied, resulting subset 11 biomarkers used build five models, demonstrating high performance sensitivities specificities best case greater than 82% 89%, respectively. The percentage lymphocytes, angiotensin‐converting enzyme, lactate dehydrogenase indexes revealed, among others, cumulative importance for models. Our reveals that these could detect chronic status potentially serve supportive tool diagnosis, monitoring, silicosis.
Language: Английский
Citations
2Health Information Science and Systems, Journal Year: 2024, Volume and Issue: 12(1)
Published: July 16, 2024
Abstract Diagnosing autism spectrum disorder (ASD) in children poses significant challenges due to its complex nature and impact on social communication development. While numerous data analytics techniques have been proposed for ASD evaluation, the process remains time-consuming lacks clarity. Eye tracking (ET) has emerged as a valuable resource risk assessment, yet existing literature predominantly focuses predictive methods rather than descriptive that offer human-friendly insights. Interpretation of ET Bayley scales, widely used assessment tool, is challenging children. It should be understood clearly perform better analytic tasks screening. Therefore, this study addresses gap by employing linguistic summarization generate easily understandable summaries from raw scales. By integrating scores, aims improve identification with typically developing (TD). Notably, research represents one pioneering efforts linguistically summarize alongside presenting comparative results between TD. Through summarization, facilitates creation simple, natural language statements, offering first unique approach enhance screening contribute our understanding neurodevelopmental disorders.
Language: Английский
Citations
2World Wide Web, Journal Year: 2023, Volume and Issue: 26(6), P. 3759 - 3794
Published: Sept. 11, 2023
Abstract
Given
a
user
dataset
$$\varvec{U}$$
Language: Английский
Citations
2ICST Transactions on Scalable Information Systems, Journal Year: 2024, Volume and Issue: 11
Published: Nov. 20, 2024
The development of big data analysis technology has brought new opportunities to the production and management various industries. Through mining in operation process enterprises by technology, internal associated even entire industry can be obtained. As a common method for large-scale statistical analysis, clustering effectively mine relationship within massive heterogeneous multidimensional data, complete unlabeled classification, provide support model data. Common density methods are time-consuming easy cause errors allocation, which affects accuracy clustering. Therefore we propose novel large peak based on sparse auto-encoder space meshing via evidence probability distribution. Firstly, deep learning is used achieve feature extraction dimensionality reduction input high-dimensional matrix through training. Secondly, meshed reduce calculation distance between sample points. When calculating local density, not only value grid itself, but also nearest neighbors considered, reduces influence subjective selection truncation results improves accuracy. threshold set ensure stability results. Using K-nearest neighbor information points, transfer distribution strategy proposed optimize remaining so as avoid joint error experimental show that algorithm higher better performance than other advanced algorithms artificial real sets.
Language: Английский
Citations
0Research Square (Research Square), Journal Year: 2023, Volume and Issue: unknown
Published: Feb. 16, 2023
Language: Английский
Citations
0