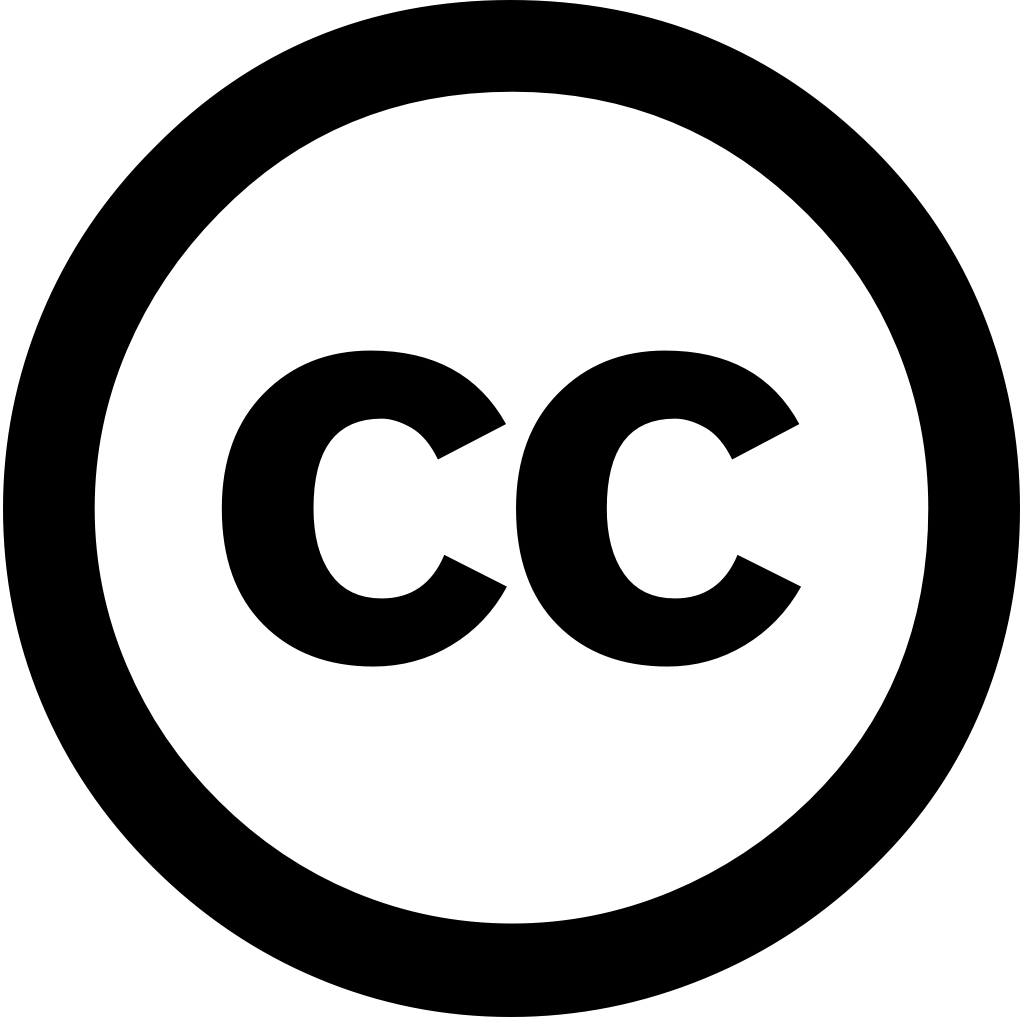
Nano-Micro Letters, Journal Year: 2025, Volume and Issue: 17(1)
Published: April 10, 2025
Language: Английский
Nano-Micro Letters, Journal Year: 2025, Volume and Issue: 17(1)
Published: April 10, 2025
Language: Английский
Polymers, Journal Year: 2025, Volume and Issue: 17(5), P. 694 - 694
Published: March 5, 2025
The growing demand for high-performance and cost-effective composite materials necessitates advanced computational approaches optimizing their composition properties. This study aimed at the application of machine learning prediction optimization functional properties composites based on a thermoplastic matrix with various fillers (two types fibrous, four dispersed, two nano-dispersed fillers). experimental methods involved material production through powder metallurgy, further microstructural analysis, mechanical tribological testing. analysis revealed distinct structural modifications interfacial interactions influencing key findings indicate that optimal filler selection can significantly enhance wear resistance while maintaining adequate strength. Carbon fibers 20 wt. % improved (by 17–25 times) reducing tensile strength elongation. Basalt 10 provided an effective balance between reinforcement 11–16 times). Kaolin 2 greatly enhanced 45–57 moderate reduction. Coke maximized 9−15 acceptable Graphite ensured wear, as higher concentrations drastically decreased Sodium chloride 5 offered improvement 3–4 minimal impact Titanium dioxide 3 11–12.5 slightly Ultra-dispersed PTFE 1 optimized both work analyzed in detail effect content learning-driven prediction. Regression models demonstrated high R-squared values (0.74 density, 0.67 strength, 0.80 relative elongation, 0.79 intensity), explaining up to 80% variability Despite its efficiency, limitations include potential multicollinearity, lack consideration external factors, need validation under real-world conditions. Thus, approach reduces extensive testing, minimizing waste costs, contributing SDG 9. highlights use polymer design, offering data-driven framework rational choice fillers, thereby sustainable industrial practices.
Language: Английский
Citations
0Nano-Micro Letters, Journal Year: 2025, Volume and Issue: 17(1)
Published: April 10, 2025
Language: Английский
Citations
0