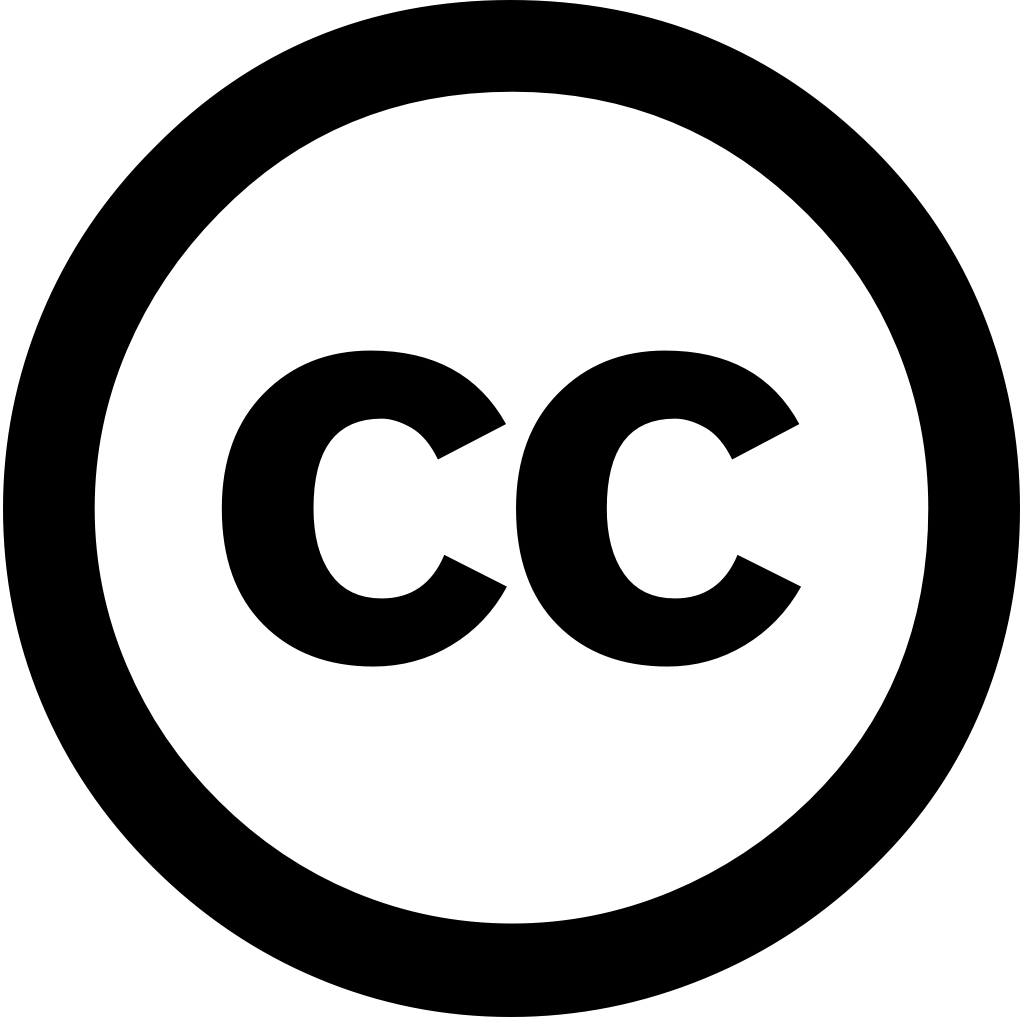
Frontiers in Environmental Science, Journal Year: 2024, Volume and Issue: 12
Published: Nov. 19, 2024
Global climate change is an extensive phenomenon characterized by alterations in weather patterns, temperature trends, and precipitation levels. These variations substantially impact agrifood systems, encompassing the interconnected components of farming, food production, distribution. This article analyzes 8,100 data points with 27 input features that quantify diverse aspects system’s contribution to predicted Greenhouse Gas Emissions (GHGE). The study uses two machine learning algorithms, Long-Short Term Memory (LSTM) Random Forest (RF), as well a hybrid approach (LSTM-RF). LSTM-RF model integrates strengths LSTM RF. LSTMs are adept at capturing long-term dependencies sequential through memory cells, addressing vanishing gradient problem. Meanwhile, its ensemble approach, RF improves overall performance generalization combining multiple weak learners. Additionally, provides insights into importance features, helping understand significant contributors model’s predictions. results demonstrate algorithm outperforms other algorithms (for test subset, RMSE = 2.977 R 2 0.9990). findings highlight superior accuracy compared individual being less accurate comparison. As determined Pearson correlation analysis, key variables such on-farm energy use, pesticide manufacturing, land use factors significantly influence GHGE outputs. Furthermore, this heat map visually represent coefficient between GHGE, enhancing our understanding complex interactions within system. Understanding intricate connection systems crucial for developing practices security environmental challenges.
Language: Английский