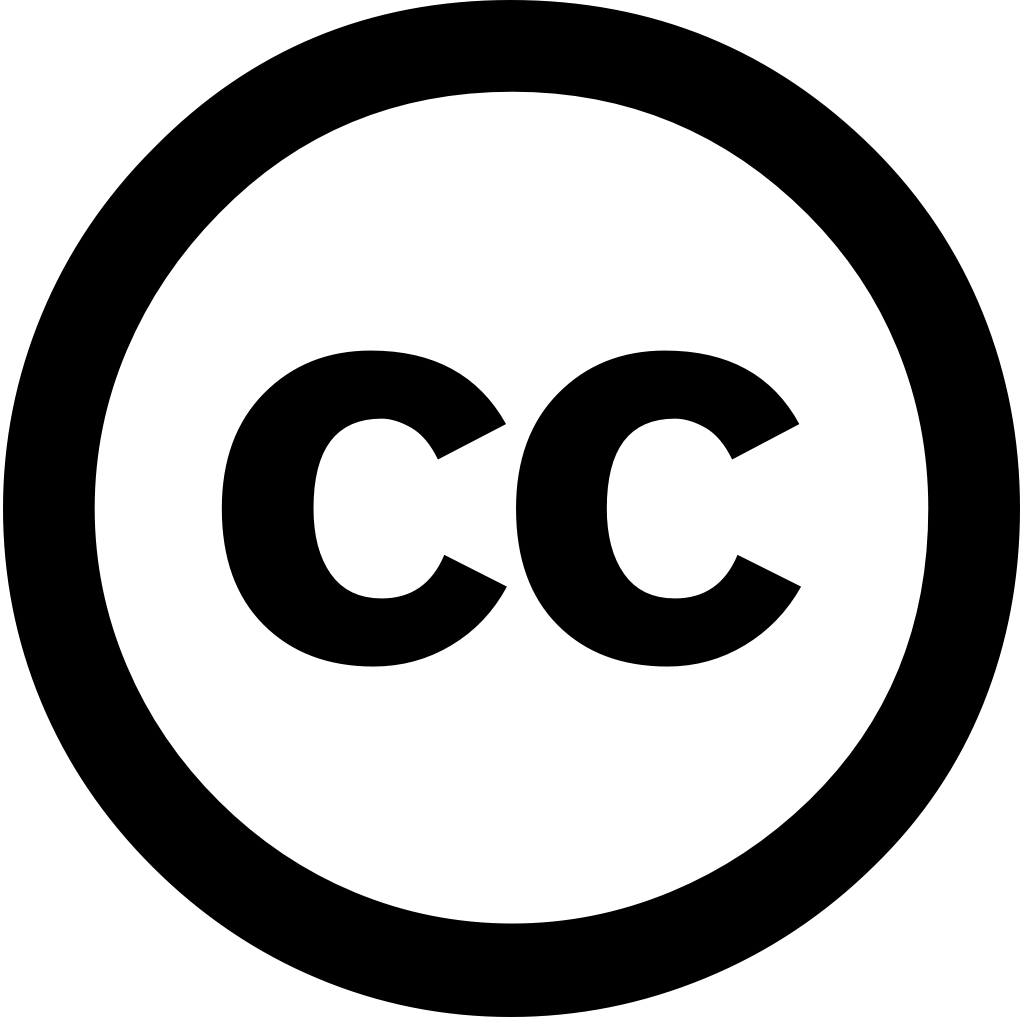
Discover Water, Journal Year: 2024, Volume and Issue: 4(1)
Published: Dec. 31, 2024
Language: Английский
Discover Water, Journal Year: 2024, Volume and Issue: 4(1)
Published: Dec. 31, 2024
Language: Английский
International Journal of Applied Earth Observation and Geoinformation, Journal Year: 2025, Volume and Issue: 136, P. 104357 - 104357
Published: Jan. 14, 2025
Language: Английский
Citations
3Geomatics Natural Hazards and Risk, Journal Year: 2024, Volume and Issue: 15(1)
Published: May 28, 2024
Frequent floods caused by monsoons and rainstorms have significantly affected the resilience of human natural ecosystems in Nam Ngum River Basin, Lao PDR. A cost-efficient framework integrating advanced remote sensing machine learning techniques is proposed to address this issue enhancing flood susceptibility understanding informed decision-making. This study utilizes geo-datasets algorithms (Random Forest, Support Vector Machine, Artificial Neural Networks, Long Short-Term Memory) generate comprehensive maps. The results highlight Random Forest's superior performance, achieving highest train test Area Under Curve Receiver Operating Characteristic (AUROC) (1.00 0.993), accuracy (0.957), F1-score (0.962), kappa value (0.914), with lowest mean squared error (0.207) Root Mean Squared Error (0.043). Vulnerability particularly pronounced low-elevation low-slope southern downstream areas (Central part PDR). reveal that 36%–53% basin's total area highly susceptible flooding, emphasizing dire need for coordinated floodplain management strategies. research uses freely accessible data, addresses data scarcity studies, provides valuable insights disaster risk sustainable planning
Language: Английский
Citations
9Earth Systems and Environment, Journal Year: 2024, Volume and Issue: unknown
Published: Sept. 2, 2024
Language: Английский
Citations
9Natural Hazards, Journal Year: 2025, Volume and Issue: unknown
Published: Jan. 11, 2025
Abstract Floods are natural disasters with significant economic and infrastructural impacts. Assessing flood susceptibility in mountainous urban regions is particularly challenging due to the complicated interaction which structures terrain affect behavior. This study employs two ensemble machine learning algorithms, Extreme Gradient Boosting (XGBoost) Random Forest (RF), develop maps for Hunza-Nagar region, has been experiencing frequent flooding past three decades. An unsteady flow simulation carried out HEC-RAS utilizing a 100-year return period hydrograph as an input boundary condition, output of provided spatial inundation extents necessary developing inventory. Ten explanatory factors, including climatic, geological, geomorphological features namely elevation, slope, curvature, topographic wetness index (TWI), normalized difference vegetation (NDVI), land use cover (LULC), rainfall, lithology, distance roads rivers considered mapping. For inventory, random sampling technique adopted create repository non-flood points, incorporating ten geo-environmental conditioning factors. The models’ accuracy assessed using area under curve (AUC) receiver operating characteristics (ROC). prediction rate AUC values 0.912 RF 0.893 XGBoost, also demonstrating superior performance accuracy, precision, recall, F1-score, kappa evaluation metrics. Consequently, model selected represent map area. resulting will assist national disaster management infrastructure development authorities identifying high susceptible zones carrying early mitigation actions future floods.
Language: Английский
Citations
1Economics of Disasters and Climate Change, Journal Year: 2025, Volume and Issue: unknown
Published: Jan. 30, 2025
Language: Английский
Citations
0Frontiers in Water, Journal Year: 2025, Volume and Issue: 7
Published: March 18, 2025
Floods are the most common natural hazard, causing major economic losses and severely affecting people’s lives. Therefore, accurately identifying vulnerable areas is crucial for saving lives resources, particularly in regions with restricted access insufficient data. The aim of this study was to automate identification flood-prone within a data-scarce, mountainous watershed using remote sensing (RS) machine learning (ML) models. In study, we integrate Normalized Difference Flood Index (NDFI), Google Earth Engine generate flood inventory, which considered step susceptibility mapping. Seventeen determining factors, namely, elevation, slope, aspect, curvature, Stream Power (SPI), Topographic Wetness (TWI), Ruggedness (TRI), Position (TPI), distance from roads, rivers, stream density, rainfall, lithology, Vegetation (NDVI), land use, length slope (LS) factor, Convergence were used map vulnerability. This aimed assess predictive performance gradient boosting, AdaBoost, random forest. model evaluated area under curve (AUC). assessment results showed that forest (RF) achieved highest accuracy (1), followed by boosting ensemble (RF-GB) (0.96), (GB) (0.95), AdaBoost (AdaB) (0.83). Additionally, research employed Shapely Additive Explanations (SHAP) method, explain predictions determine contributing factor each model. introduces novel approach providing significant insights into mapping, offering potential pathways future practical applications. Overall, emphasizes need urban planning emergency preparedness build safer more resilient communities.
Language: Английский
Citations
0Deleted Journal, Journal Year: 2025, Volume and Issue: 7(4)
Published: March 31, 2025
Language: Английский
Citations
0ISPRS International Journal of Geo-Information, Journal Year: 2025, Volume and Issue: 14(4), P. 161 - 161
Published: April 8, 2025
Rapid urbanization and climate change are increasing the risks associated with natural hazards, especially in cities where socio-economic disparities significant. Current hazard risk assessment frameworks fail to consider factors, which limits their ability effectively address vulnerabilities at community level. This study introduces a machine learning framework designed assess flood susceptibility vulnerability, particularly urban areas limited data. Using Kigali, Rwanda, as case study, we quantified vulnerability through composite index that includes indicators of sensitivity adaptive capacity. We utilized variety data sources, such demographic, environmental, remotely sensing datasets, applying algorithms like Multilayer Perceptron (MLP), Random Forest, Support Vector Machine (SVM), XGBoost. Among these, MLP achieved best predictive performance, an AUC score 0.902 F1-score 0.86. The findings indicate spatial differences central southern Kigali showing greater due mix challenges high risk. maps created were validated against historical records, research, expert insights, confirming accuracy relevance for assessment. Additionally, tested framework’s scalability adaptability Kampala, Uganda, Dar es Salaam, Tanzania, making context-specific adjustments model improves its transferability. offers solid, data-driven approach combining assessments filling important gaps resilience planning. results support advancement risk-informed decision-making, access detailed information.
Language: Английский
Citations
0Remote Sensing, Journal Year: 2025, Volume and Issue: 17(9), P. 1540 - 1540
Published: April 26, 2025
Flooding is one of the most devastating natural disasters worldwide, with increasing frequency due to climate change. Traditional hydrological models require extensive data and computational resources, while machine learning (ML) struggle capture spatial dependencies. To address this, we propose a modified U-Net architecture that integrates prior knowledge permanent water bodies improve flood susceptibility mapping in Northumberland County, UK. By embedding domain-specific insights, our model achieves higher area under curve (AUC) (0.97) compared standard (0.93), also reducing training time by converging three times faster. Additionally, integrate Grad-CAM module provide visualisations explaining areas attention from model, enabling interpretation its decision-making, thus barriers practical implementation.
Language: Английский
Citations
0Remote Sensing, Journal Year: 2025, Volume and Issue: 17(9), P. 1605 - 1605
Published: April 30, 2025
On 14 December 2005, there was a catastrophic flood after failure in the upper reservoir at Taum Sauk Plant southern Missouri. While has been extensive research on cause of dam’s and flood’s immediate impact, limited investigation how vegetation around resulting scour changed since this event. This study fills gap through time-series analysis using imagery sourced from GloVis Planet Explorer to quantify levels prior (2005) 2024. Vegetation level calculated Normalized Difference Index (NDVI), which measures greenness via light reflected by vegetation. inside were compared two 120 m buffer areas surrounding scour, immediately adjacent (0–120 m) 120–240 scour’s edge. Within NDVI showed dramatic loss flood, followed varying for several years, before steady increase proportion with starting 2014. The area edge similar pattern, but lower magnitudes change, likely reflects ragged created flood. farther consistent pattern high vegetation, broader landscape. ground truthing confirmed these patterns between 2006 2011, 2012, revealed much recovery small local within that not apparent though analysis. These recolonization nearby glades (i.e., natural habitats exposed bedrock) glade flora eastern collared lizard (Crotaphytus collaris collaris), an apex predator adapted living rocky, open bioindicator recovery. occurred steadily indicated original oak/hickory forest now minor component recovery, species dominated former forested area.
Language: Английский
Citations
0