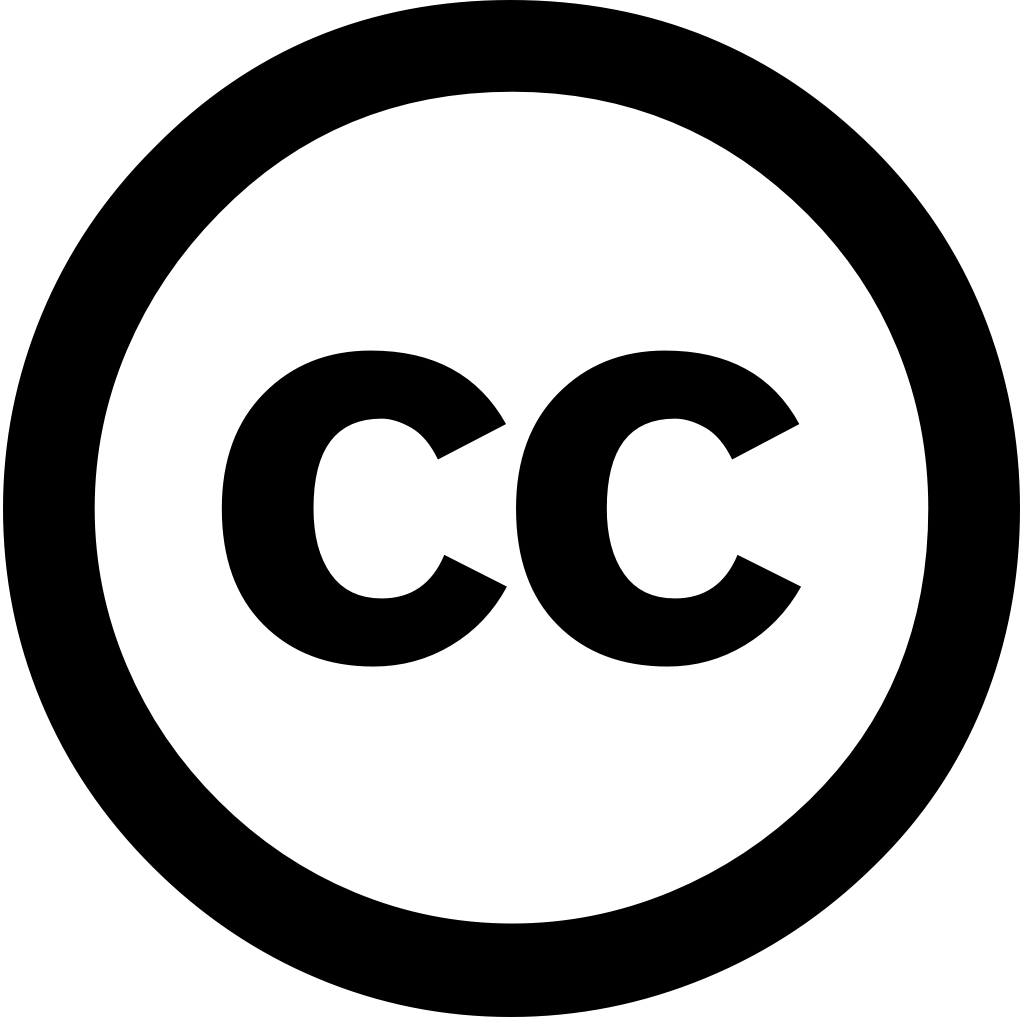
Sensors, Journal Year: 2025, Volume and Issue: 25(7), P. 2237 - 2237
Published: April 2, 2025
Traditional crop classification methods have three critical limitations: (1) dependency on labor-intensive field surveys with limited spatial coverage, (2) susceptibility to human subjectivity during manual data collection, and (3) the inability capture fine-grained spectral variations due lack of multispectral analysis. This research introduces an enhanced identification model based a residual ResNet network. leverages remote sensing images from unmanned aerial vehicles (UAVs) accurately classify complex planting structures. The focuses four typical crops: sunflower, corn, beet, pepper. By acquiring preprocessing image data, improved ResNet50 integrating ACmix self-attention module coordinate attention mechanism is developed enhance recognition accuracy these crops. Experimental results demonstrate that achieves 97.8% images, outperforming both RGB traditional methods. highlights potential combining UAV technology deep learning for precise classification, offering valuable technical support precision agriculture management.
Language: Английский