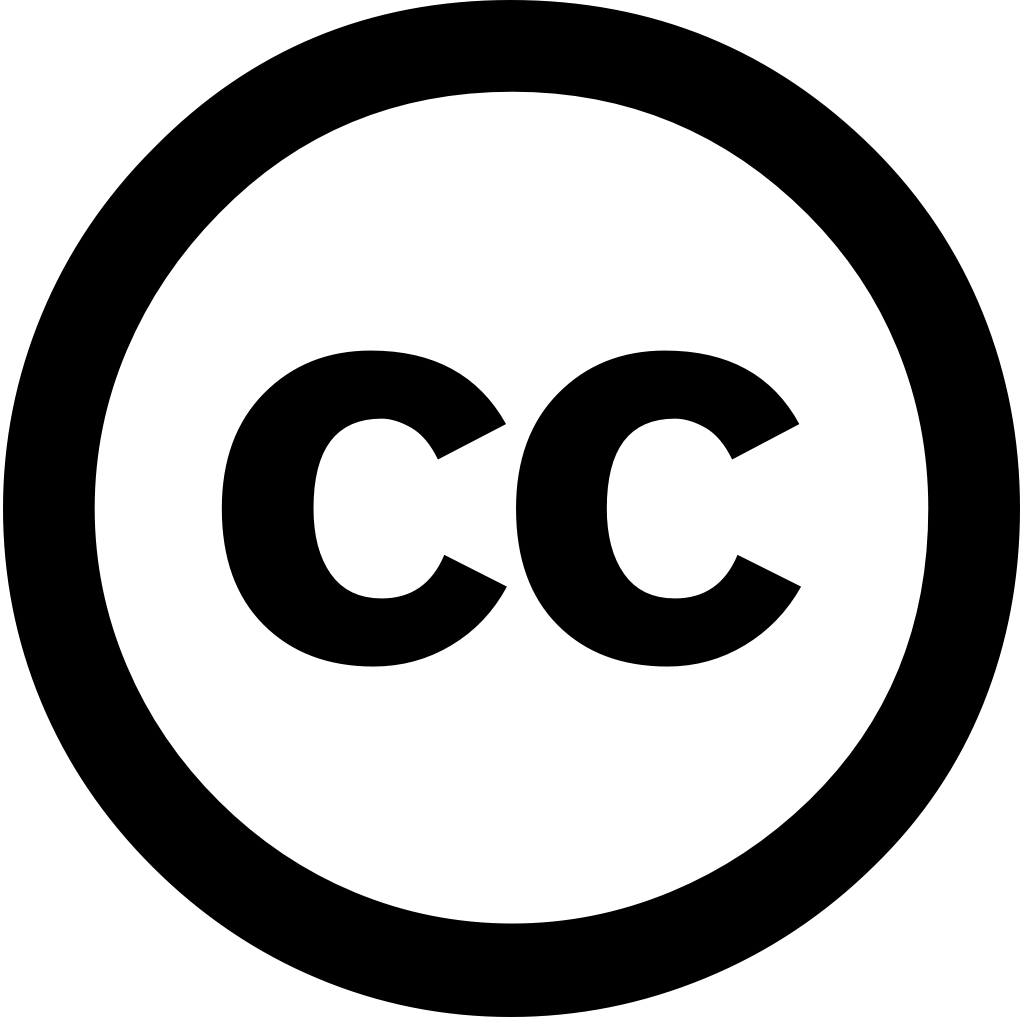
Deleted Journal, Journal Year: 2025, Volume and Issue: 28(1)
Published: April 24, 2025
Abstract In healthcare institutions, an automated system plays a critical role by enhancing patients’ satisfaction with service delivery. This paper focused on the development of model that assists patients in finding appropriate medical specialists Afaan Oromo. To do this, text preprocessing tasks were applied to data remove unnecessary texts, punctuation, and numbers, as they would be suitable for training model. A feature extraction task is obtain standard Oromo health dataset using TF-IDF. We used supervised learning algorithms such logistic regression, random forest, multi-layer perceptron, decision trees, Bi-LSTM, K-NN experimental purposes. Evaluation measures comparing performance seven specialist classes labeled dataset. comparative analysis, result reveals Bi-LSTM performed well, achieving equal value accuracy F1 score, which 0.9708. Based results, user interface was developed proposed method, highest-outperformed detect symptoms predict specialists.
Language: Английский