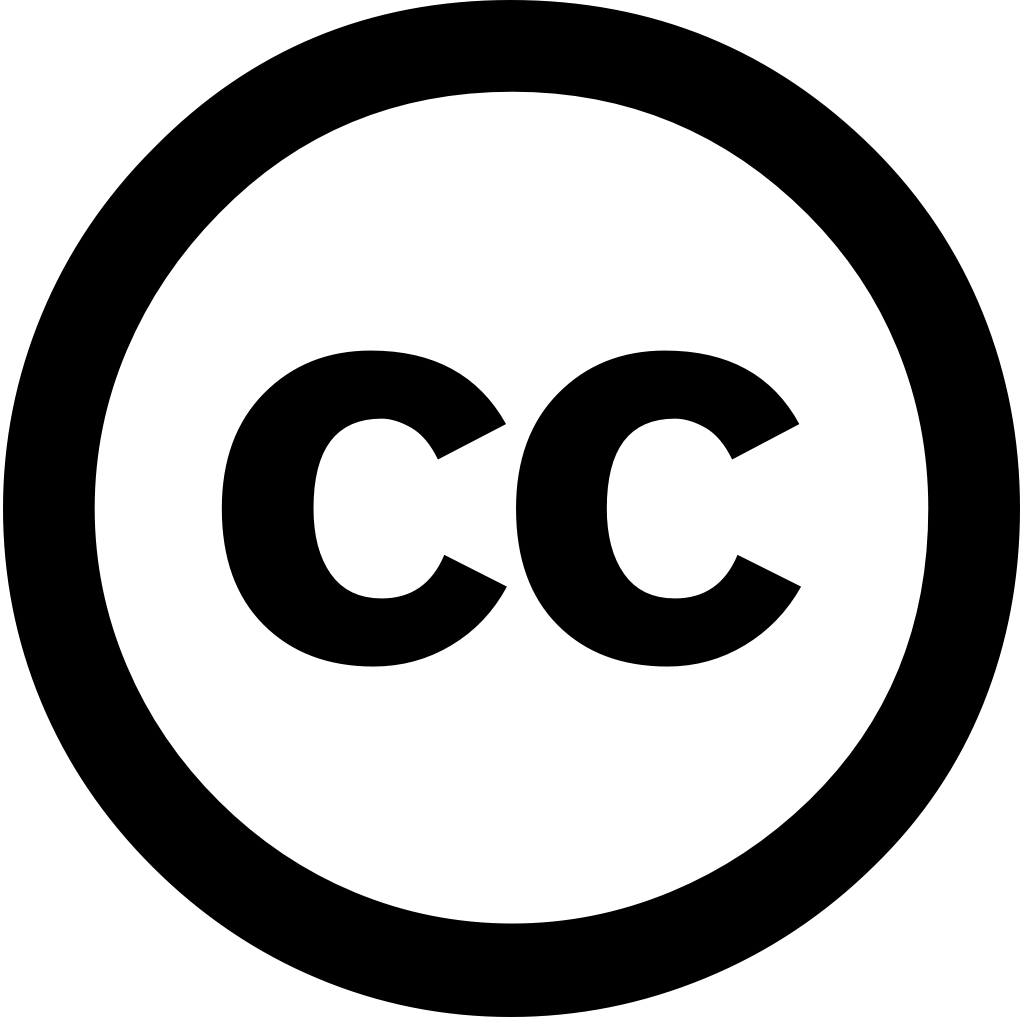
Research Square (Research Square), Journal Year: 2024, Volume and Issue: unknown
Published: May 30, 2024
Language: Английский
Research Square (Research Square), Journal Year: 2024, Volume and Issue: unknown
Published: May 30, 2024
Language: Английский
Earth Systems and Environment, Journal Year: 2025, Volume and Issue: unknown
Published: Jan. 2, 2025
Language: Английский
Citations
0Urban Science, Journal Year: 2025, Volume and Issue: 9(3), P. 78 - 78
Published: March 11, 2025
The rapid expansion of built-up areas (BUAs) requires effective spatial and temporal monitoring, being a crucial practice for urban land use planning, resource allocation, environmental studies, spectral indices (SIs) can provide efficiency reliability in automating the process BUAs extraction. This paper explores nine sixteen thresholding methods automatic mapping using Landsat 8 imagery from semi-arid climate Morocco during spring summer. These are Normalized Difference Built-Up Index (NDBI), Vis-red-NIR (VrNIR-BI), Perpendicular Impervious Surface (PISI), Combinational Biophysical Composition (CBCI), Built-up Area (NBAI), (BUI), Enhanced Surfaces (ENDISI) Land Features Extraction (BLFEI). Results show that BLFEI, SWIRED, BUI maintain high separability between each other cover types across both seasons, as evaluated via Spectral Discrimination (SDI). lowest SDI values all three were observed bare soil against BUAs, with BLFEI recording 1.21 wet season 1.05 dry season, SWIRED yielding 1.22 1.08, showing demonstrating their robustness distinguishing covers under varying phenological moisture conditions. reached overall accuracies 93.97%, 93.39% 92.81%, respectively, conditions, 91.57%, 89.17% 89.67%, assessment reveals Minimum method resulted highest these where bimodal medium peaked histograms observed, whereas Li, Huang, Shanbhag, Otsu, K-means, or IsoData was found to be most more observed.
Language: Английский
Citations
0Scientific Reports, Journal Year: 2025, Volume and Issue: 15(1)
Published: March 18, 2025
Abstract Plastic products have resulted in enormous pollution many ecosystem types and regions worldwide. The problem is particularly prominent within aquatic environments, where multiple anthropogenic sources (i.e., agriculture, urbanisation, industries, illegal dumping) are common, exacerbated by interconnectedness between terrestrial environments management challenges. Regional disparities also common macroplastic research, with a scarcity of knowledge African freshwaters. Here, considering seven riparian sites across four seasons, we determined the abundance distribution litter along South Crocodile River system its associated tributaries. Macroplastics were sorted classified into various polymer groups, functional origins, physical forms for each site season. We hypothesised that abundances would be substantial, differences among related to human activities river shores, during summer months when recreational was high. observed significant variation high autumn (338), while lower total numbers macroplastics unexpectedly collected spring (243–263). High proportional plastic bags film all seasons observed, as well polypropylene polymers. Our study serves baseline understanding seasonal variations their driving factors subtropical systems, which may help inform policies. further contributes resolving gaps underrepresented regions, providing novel insights sources, accumulation, impacts linked unique socio-environmental contexts. Thus, it bridges critical data gaps, informing targeted interventions global comparative analyses waste management.
Language: Английский
Citations
0DELETED, Journal Year: 2024, Volume and Issue: unknown
Published: Aug. 25, 2024
Fly ash pollutants, which are created in various power plants and industries as a result of the mineral matters coal, being transformed during combustion, among most harmful pollutants today. It is usually disposed ponds or artificial pits. combines with rainfall monsoon season spreads to nearby areas. Consequently, it has negative effect on ecosystem. Monitoring soil contamination using physiochemical processes time-consuming arduous procedure that can be reduced by analyzing satellite data, takes less time produces systematic rapidly. The accuracy land use cover categorization depends imagery appropriateness suitable classifier selection. Hence, this study attempts examine changes due fly Nagpur district, Maharashtra, India, temporal Landsat-8 images over period 2013–2023 Support Vector Machine (SVM) classifier. SVM reported an average value overall equal 87.64% kappa coefficient 0.83. From results obtained, was seen area increased from 23.4 hectares 2013 134.1 2023. If proper care not taken, increase ultimately leaches into tributary Kanhan River, passes nearby, makes water undrinkable. In addition leaching, toxins travel through environment erosion, runoff, air fine dust.
Language: Английский
Citations
2Forests, Journal Year: 2023, Volume and Issue: 14(9), P. 1881 - 1881
Published: Sept. 15, 2023
Convolutional neural networks (CNNs) and recurrent (RNNs) have gained improved results in remote sensing image data classification. Multispectral classification can benefit from the rich spectral information extracted by these models for land cover This paper proposes a model called hierarchical convolutional network (HCRNN) to combine CNN RNN modules pixel-level of multispectral images. In HCRNN model, original 13-band Sentinel-2 is transformed into 1D sequence using fully connected layer. It then reshaped 3D feature matrix. The 2D-CNN features are used as inputs corresponding RNN. at each level adapted same convolution size. structure leverages advantages CNNs RNNs extract temporal spatial data, leading high-precision experimental demonstrate that overall accuracy on dataset reaches 97.62%, which improves performance 1.78% compared model. Furthermore, this study focused changes forest area Laibin City, Guangxi Zhuang Autonomous Region, was 7997.1016 km2, 8990.4149 8103.0020 km2 2017, 2019, 2021, respectively, with an trend small increase covered.
Language: Английский
Citations
6DELETED, Journal Year: 2024, Volume and Issue: 90(3), P. 706 - 721
Published: Feb. 23, 2024
Red mud & fly ash are industrial waste generated during the extraction of alumina from bauxite. is composed various oxides including iron oxide in bulk which gives it red colour. On other hand, Fly grey its colour with a very fine size and can be easily carried away by wind. Both Ash contain high concentrations elements radioactive elements. Its composition depends upon type raw material used. It disposed into man made ponds, landfills etc. Due to hazardous nature if not stored/disposed properly pose serious environmental hazard. Hence regular monitoring needed. simple use satellite data freely available systematic results generated. This study attempts examine spectral reflectance signature pollutants-Red feature also analyse contamination surrounding area Aluminium Extraction Company nearby water reservoir using multi-spectral Landsat-8 Satellite image employing three supervised machine learning classifiers i.e., Maximum Likelihood (ML) classifier, Support Vector Machine (SVM) classifier Artificial Neural Network (ANN) over period 2015 2023. The result obtained for five years reveals that SVM yielded better overall accuracy kappa coefficients average value classification outputs were 89.11% 0.83 followed 83.19% 0.74, whereas lowest i.e. 82.68% 0.76 respectively. carefully examined comparing accuracies then visual analysis. From analysis was concluded contaminated found widely spread areas too. present indicated significant changes terms wide pollutants have occurred region, has degraded environment lot.
Language: Английский
Citations
1IOP Conference Series Earth and Environmental Science, Journal Year: 2023, Volume and Issue: 1266(1), P. 012085 - 012085
Published: Dec. 1, 2023
Abstract Land use change commonly has an impact on urban areas. The land and cover (LULC) model should be used to address problems. conversion is inextricably linked Samarinda, Indonesia, the administrative center of province East Kalimantan. Additionally, this city serves as new capital city’s Ibu Kota Nusantara-specific buffer zone. current study aims find assess Samarinda City’s LULC. A supervised maximum likelihood technique was extract data from spatiotemporal images. Both spatial non-spatial about changes in LULC are study. imagery for region includes Landsat 5, 7, 9 images 1994 2022, along with maps GPS measurement enabling on-the-ground checkpoints. Before classification analysis, radiometric atmospheric correction, cropping, layer stacking procedures were treat image data. In classification, method applied four classes uncultivated vegetation, cultivated waterbodies, buildings. research result indicates that number decreased by 6.38 percent percentage 5.52 percent, according study’s findings. On other hand, proportion water bodies increased 2.39 built-up 9.51 percent. overall kappa accuracy test’s average value 97.67%, higher than required minimum 75%.
Language: Английский
Citations
3Research Square (Research Square), Journal Year: 2024, Volume and Issue: unknown
Published: May 30, 2024
Language: Английский
Citations
0