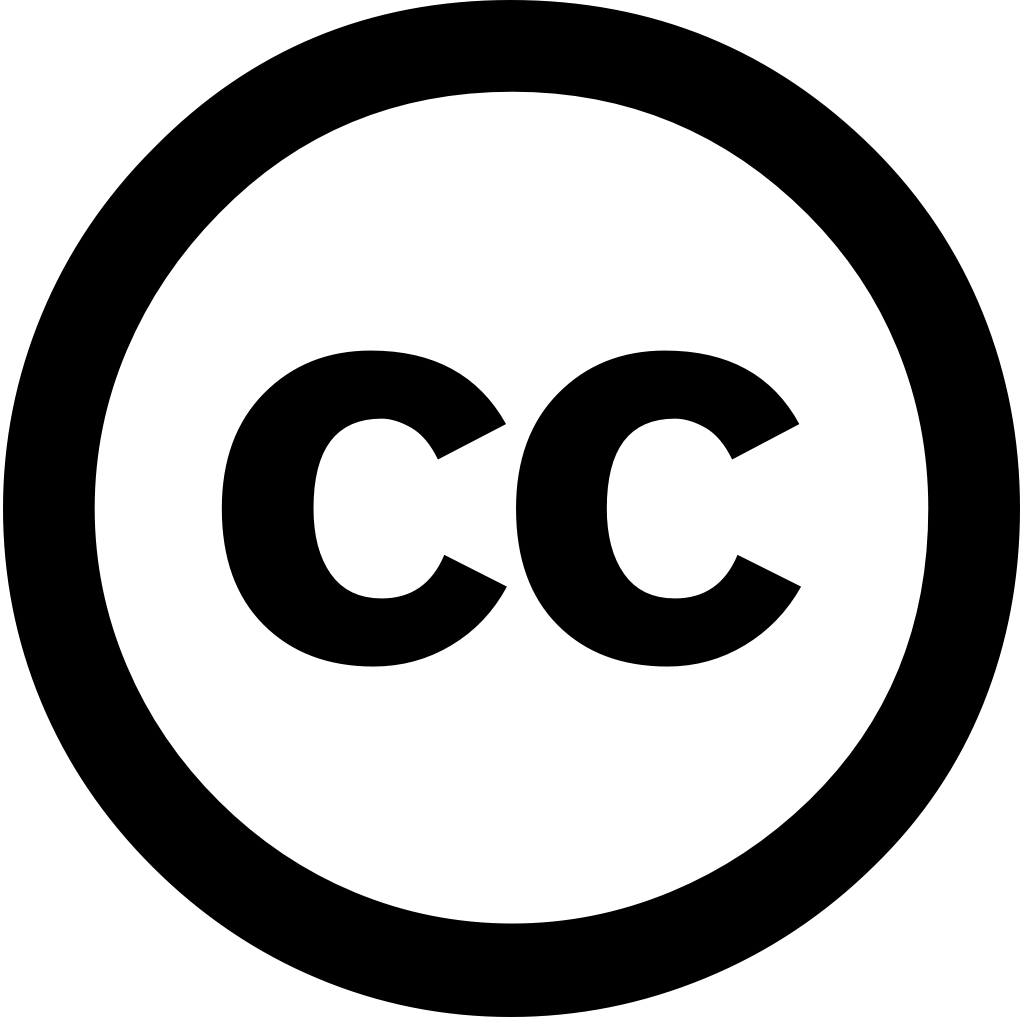
International Journal of Optics, Journal Year: 2025, Volume and Issue: 2025(1)
Published: Jan. 1, 2025
Free‐space optical (FSO) communication is vital for modern wireless systems due to its high data rates, energy efficiency, secure transmission, and cost‐effectiveness. However, weather‐induced attenuation, turbulence, pointing errors affect performance. Recent advancements leverage machine learning (ML) predict enhance system reliability under adverse conditions, improving channel awareness quality of service (QoS). This study has applied modulation schemes, including on‐off keying with nonreturn‐to‐zero (OOK–NRZ), quadrature phase shift (QPSK), polarization division multiplexed QPSK (PDM–QPSK), (PolSK) an ultra‐dense wavelength (WDM) FSO‐fiber‐to‐the‐x modulate the across clear, fog, rain, hazy conditions. Furthermore, multiparameter performance predictions diverse weather conditions have been assessed using ML algorithms such as extreme (ELM), support vector (SVM), gradient boosting (GB). Mean squared error (MSE) coefficient determination ( R 2 ) statistical measures employed measure robustness ML. Modulation atmospheric input powers, FSO link length are fed features. At same time, primary modeling targets signal‐to‐noise ratio (OSNR), factor (QF), bit rate (BER), rate, received power. The simulation results demonstrated that GB achieved model’s best reduced MSE values OSNR, QF, BER, power 2.0203e − 03, 9.3905e 04, 3.5214e 12, 1.2527e respectively. Moreover, exceptional ‐squared 0.9997, 0.9998, 0.9987, 1,
Language: Английский