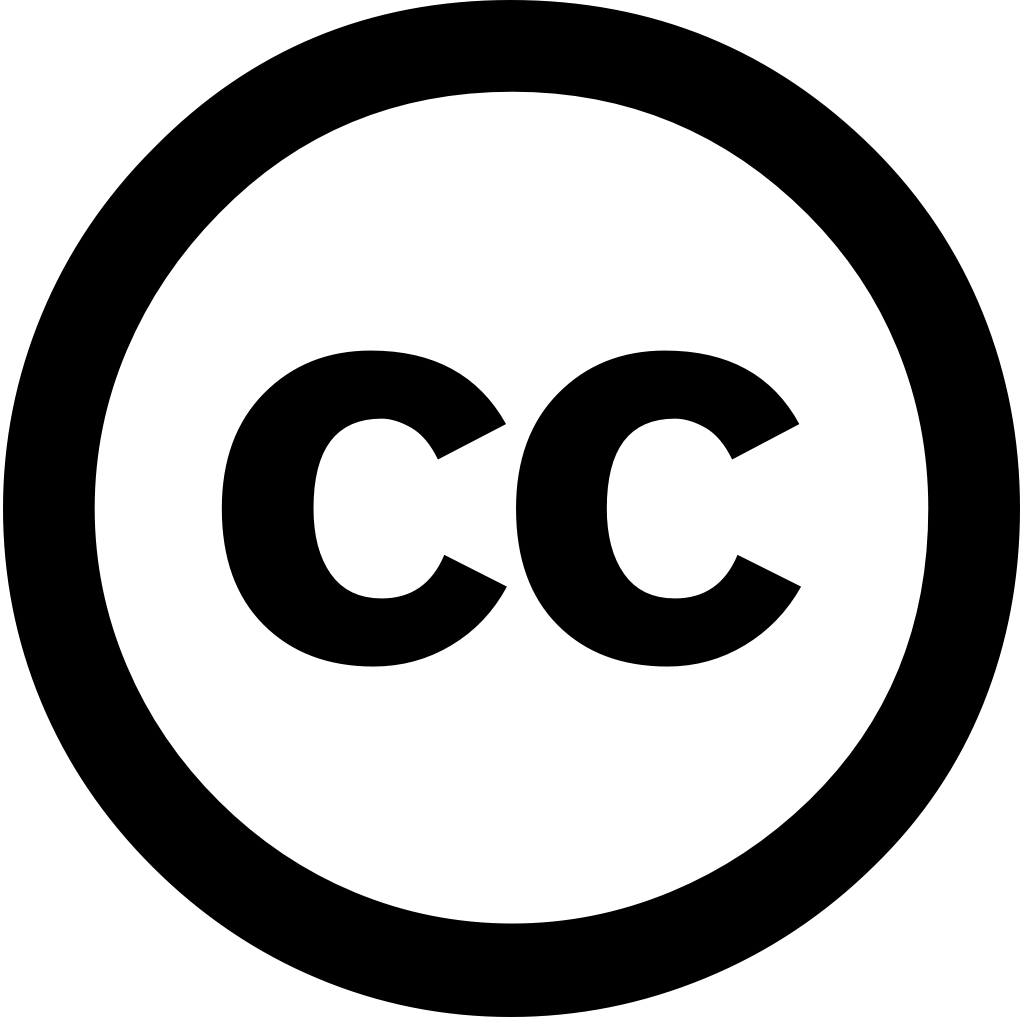
Machines, Journal Year: 2024, Volume and Issue: 12(10), P. 716 - 716
Published: Oct. 10, 2024
Unmanned aerial vehicles (UAVs) are equipped with various sensors to facilitate control and navigation. However, UAV highly susceptible damage under complex flight environments, leading severe accidents economic losses. Although fault diagnosis methods based on deep neural networks have been widely applied in the mechanical field, these often fail integrate multi-source information overlook importance of system prior knowledge. As a result, this study employs spatial-temporal difference graph convolutional network (STDGCN) for sensors, where structure naturally organizes diverse sensors. Specifically, layer enhances feature extraction capability nodes, modules designed extract dependencies from sensor data. Moreover, ensure accuracy association graph, research introduces UAV’s dynamic model as knowledge constructing graph. Finally, diagnostic accuracies 94.93%, 98.71%, 92.97% were achieved three self-constructed datasets. In addition, compared commonly used data-driven approaches, proposed method demonstrates superior capabilities achieves highest accuracy.
Language: Английский