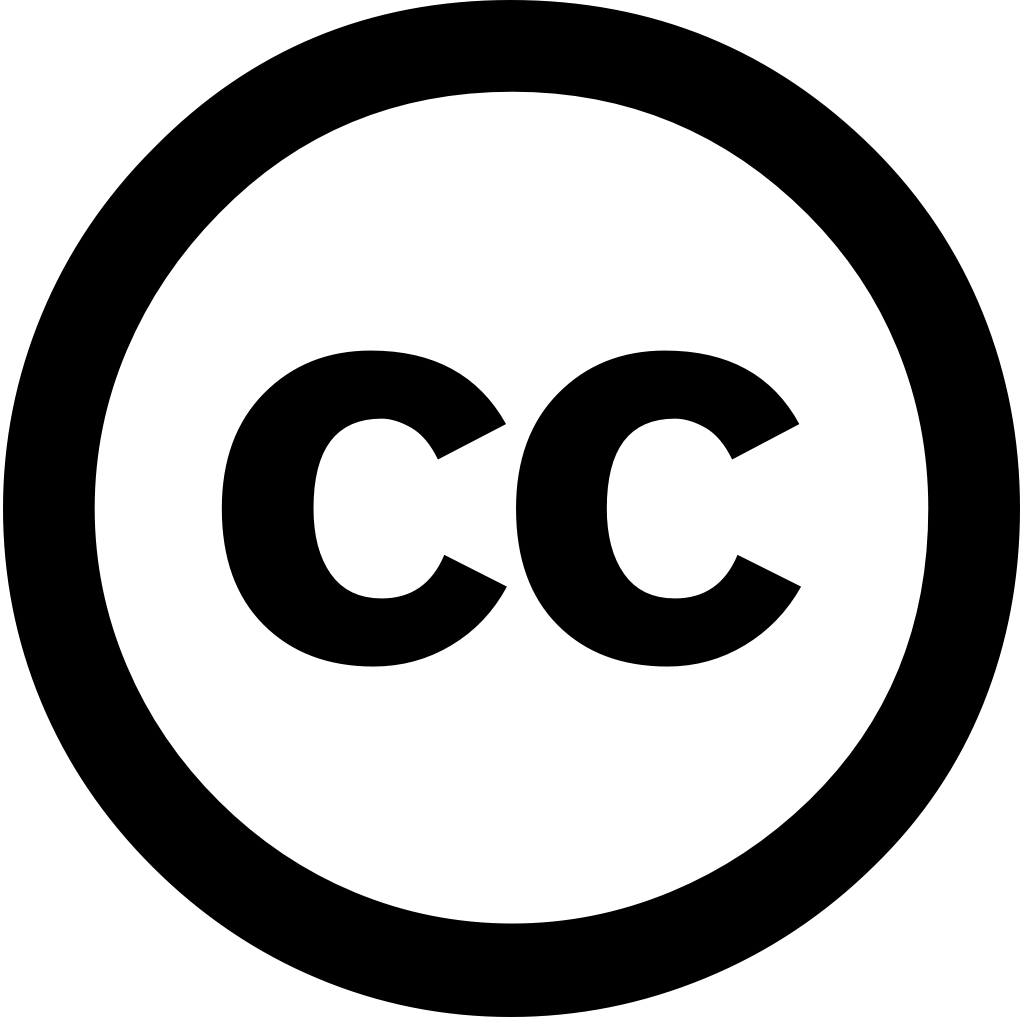
Scientific Reports, Journal Year: 2025, Volume and Issue: 15(1)
Published: May 13, 2025
Language: Английский
Scientific Reports, Journal Year: 2025, Volume and Issue: 15(1)
Published: May 13, 2025
Language: Английский
Scientific Reports, Journal Year: 2025, Volume and Issue: 15(1)
Published: March 17, 2025
Abstract The increasing prevalence of malware presents a critical challenge to cybersecurity, emphasizing the need for robust detection methods. This study uses binary tabular classification dataset evaluate impact feature selection, scaling, and machine learning (ML) models on detection. methodology involves experimenting with three scaling techniques (no normalization, min-max scaling), selection methods Linear Discriminant Analysis (LDA), Principal Component (PCA)), twelve ML models, including traditional algorithms ensemble A publicly available 11,598 samples 139 features is utilized, model performance assessed using metrics such as accuracy, precision, recall, F1-score, AUC-ROC. Results reveal that Light Gradient Boosting Machine (LGBM) achieves highest accuracy 97.16% when PCA either or normalization are applied. Additionally, consistently outperform demonstrating their effectiveness in enhancing These findings offer valuable insights into optimizing preprocessing strategies developing reliable efficient systems.
Language: Английский
Citations
1Scientific Reports, Journal Year: 2025, Volume and Issue: 15(1)
Published: March 3, 2025
Skin cancer is the most dominant and critical method of cancer, which arises all over world. Its damaging effects can range from disfigurement to major medical expenditures even death if not analyzed preserved timely. Conventional models skin recognition require a complete physical examination by specialist, time-wasting in few cases. Computer-aided medicinal analytical methods have gained massive popularity due their efficiency effectiveness. This model assist dermatologists initial significant for early diagnosis. An automatic classification utilizing deep learning (DL) help doctors perceive kind lesion improve patient's health. The one hot topics research field, along with development DL structure. manuscript designs develops Detection Cancer Using an Ensemble Deep Learning Model Gray Wolf Optimization (DSC-EDLMGWO) method. proposed DSC-EDLMGWO relies on biomedical imaging. presented initially involves image preprocessing stage at two levels: contract enhancement using CLAHE noise removal wiener filter (WF) model. Furthermore, utilizes SE-DenseNet method, fusion squeeze-and-excitation (SE) module DenseNet extract features. For process, ensemble models, namely long short-term memory (LSTM) technique, extreme machine (ELM) model, stacked sparse denoising autoencoder (SSDA) employed. Finally, gray wolf optimization (GWO) optimally adjusts models' hyperparameter values, resulting more excellent performance. effectiveness approach evaluated benchmark database, outcomes measured across various performance metrics. experimental validation portrayed superior accuracy value 98.38% 98.17% under HAM10000 ISIC datasets other techniques.
Language: Английский
Citations
0International Journal of Advanced Research in Science Communication and Technology, Journal Year: 2025, Volume and Issue: unknown, P. 370 - 382
Published: April 11, 2025
The human skin acts as the body’s primary defense barrier and is vulnerable to a wide range of dermatological conditions including acne, eczema, psoriasis, dermatomyositis, cellulitis, candidiasis, scleroderma, ringworm, chickenpox, melanoma. These conditions, if left undiagnosed or untreated in their early stages, can lead serious health complications. In many developing regions, detection remains significant challenge due lack affordable accessible diagnostic tools. This project introduces deep learning–based disease system that leverages image processing techniques machine learning models provide accurate classification conditions. proposed solution uses preprocessing techniques—such resizing, deblurring, noise reduction—to prepare images for analysis. A pre-trained MobileNetV2 model employed classify into various categories, which are then grouped normal abnormal classes facilitate triage intervention. integrated user-friendly web interface, where users upload diagnosis. After classification, displays identified along with specific guidance, condition-specific Do’s Don’ts. To improve accessibility inclusiveness, website supports language translation (English Hindi) features “Find Nearby Clinics” function Google Maps user’s geolocation recommend top five nearby dermatology clinics detailed information such address, ratings, reviews. By combining practical, real-world application, this offers an innovative, low-cost detection. It especially suited deployment remote underserved diagnosis access care significantly patient outcomes reduce risk progression..
Language: Английский
Citations
0Scientific Reports, Journal Year: 2025, Volume and Issue: 15(1)
Published: May 13, 2025
Language: Английский
Citations
0