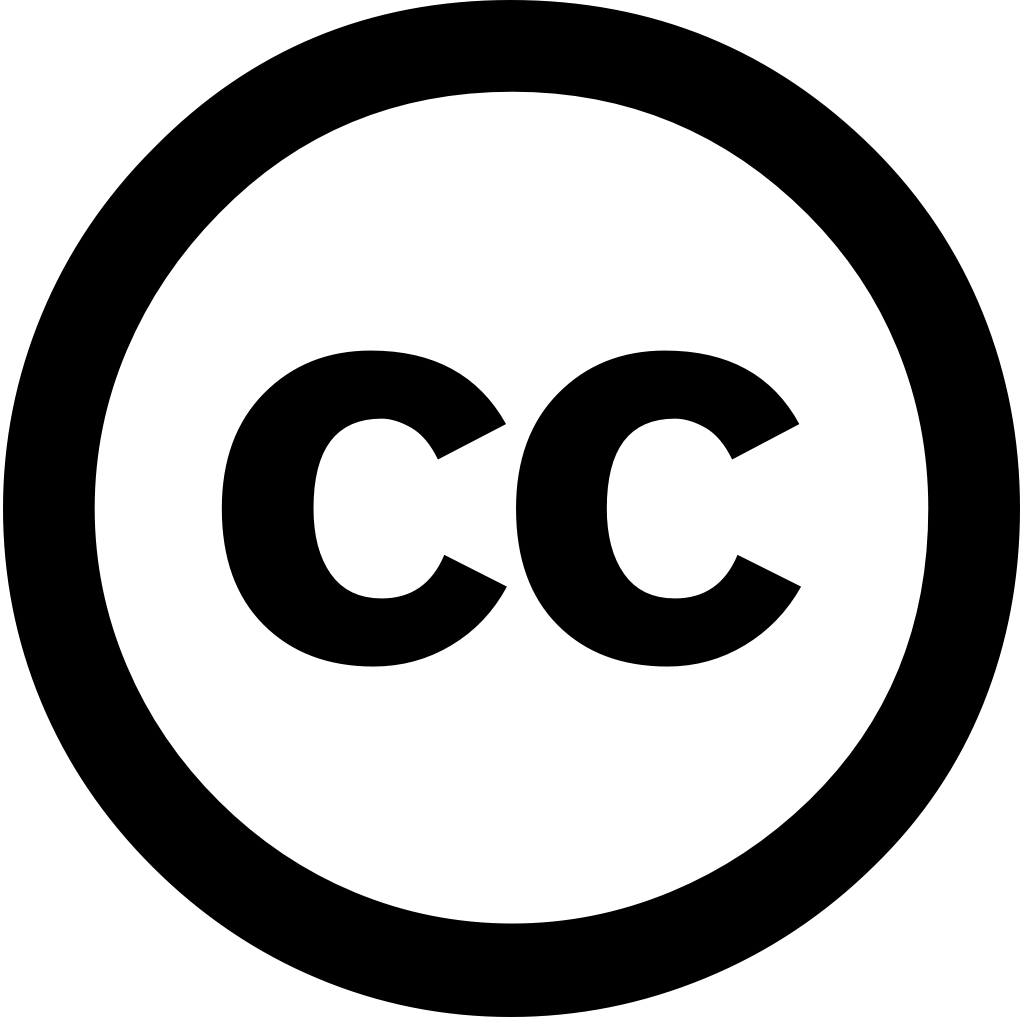
IntechOpen eBooks, Journal Year: 2025, Volume and Issue: unknown
Published: March 19, 2025
Advancements in artificial intelligence (AI) and machine learning (ML) have transformed biosensing technologies, enhancing data acquisition, analysis, interpretation biomedical diagnostics. This chapter explores AI integration into biosensing, focusing on natural language processing (NLP), large models (LLMs), augmentation, various paradigms. These technologies improve biosensor sensitivity, precision, real-time adaptability. NLP automates text extraction, while LLMs facilitate complex decision-making using vast datasets. Data augmentation mitigates dataset limitations, strengthening ML model training reducing overfitting. Supervised drives predictive for disease detection, whereas unsupervised uncovers hidden biomarker patterns. Reinforcement optimizes sensor operations, calibration, autonomous control dynamic environments. The discusses case studies, emerging trends, challenges AI-driven biosensing. AI’s convergence with edge computing Internet of Things (IoT)-enabled biosensors enhances processing, latency expanding accessibility resource-limited settings. Ethical concerns, including privacy, interpretability, regulatory compliance, must be addressed responsible applications Future research should focus developing resilient to bias, capable continuous learning, optimized low-power, portable biosensors. Addressing these will enable AI-powered advance precision medicine global healthcare outcomes. Through interdisciplinary approaches, continue drive the evolution next-generation diagnostic solutions.
Language: Английский