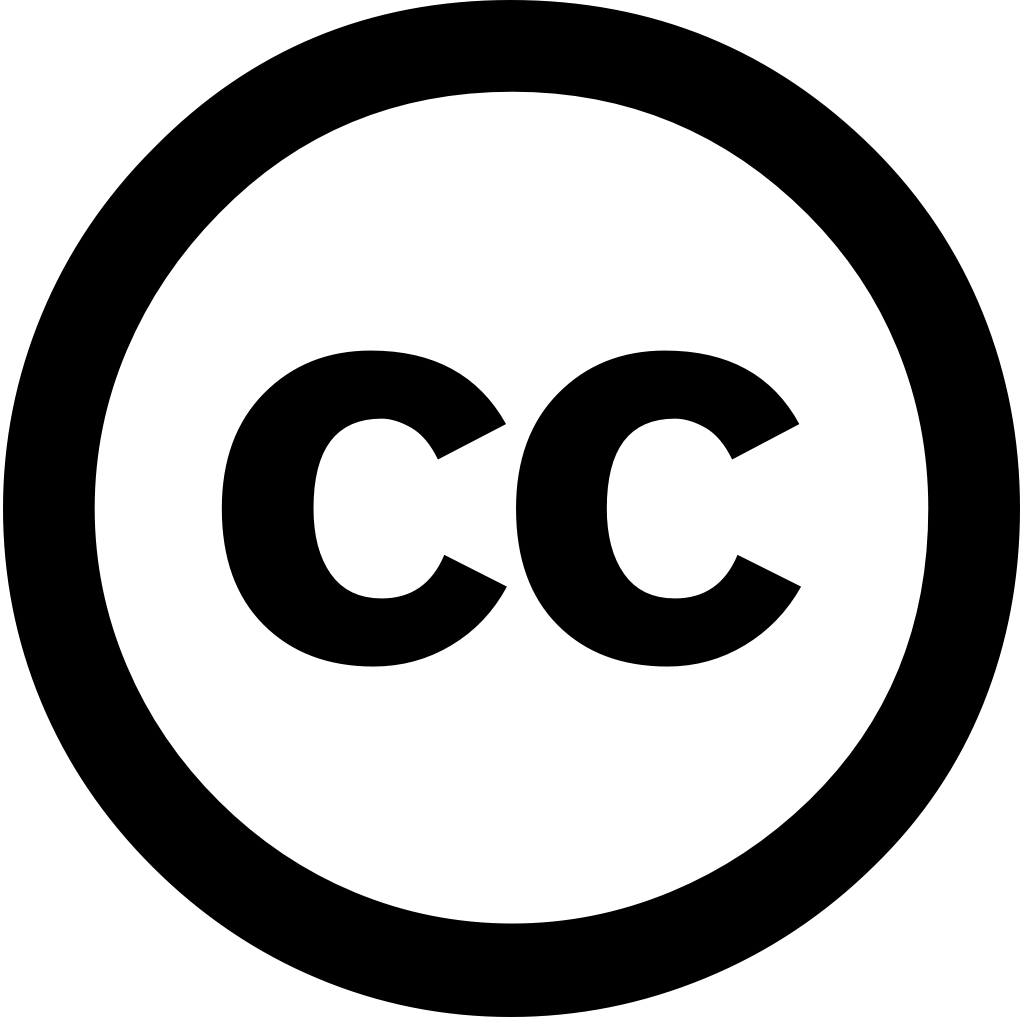
Journal of Chemical Theory and Computation, Journal Year: 2025, Volume and Issue: unknown
Published: Feb. 17, 2025
A key step in interpreting gas-phase ion mobility coupled with mass spectrometry (IM-MS) data for unknown structure prediction involves identifying the most favorable protonated structure. In gas phase, site of protonation is determined using proton affinity (PA) measurements. Currently, and ab initio computation methods are widely used to evaluate PA; however, both resource-intensive time-consuming. Therefore, there a critical need efficient estimate PA, enabling rapid identification complex organic molecules multiple binding sites. this work, we developed fast accurate method PA by descriptors combination machine learning (ML) models. Using comprehensive set 186 descriptors, our model demonstrated strong predictive performance, an R2 0.96 MAE 2.47 kcal/mol, comparable experimental uncertainty. Furthermore, designed quantum circuits as feature encoders classical neural network. To effectiveness hybrid quantum-classical model, compared its performance traditional ML models reduced derived from full set. correlation analysis showed that quantum-encoded representations have stronger positive target values than original features do. As result, outperformed counterpart achieved consistent same on noiseless simulator real hardware, highlighting potential predictions.
Language: Английский