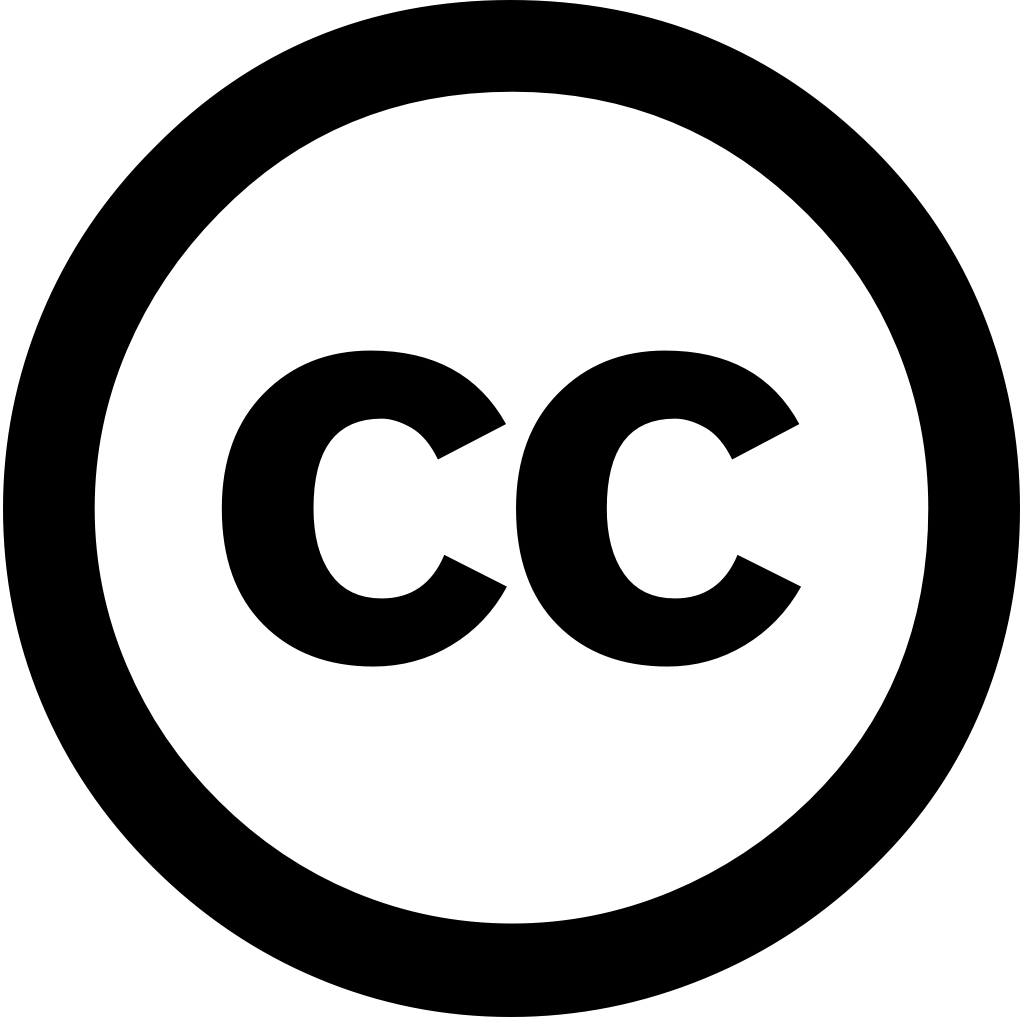
Progress in Disaster Science, Journal Year: 2024, Volume and Issue: unknown, P. 100393 - 100393
Published: Dec. 1, 2024
Language: Английский
Progress in Disaster Science, Journal Year: 2024, Volume and Issue: unknown, P. 100393 - 100393
Published: Dec. 1, 2024
Language: Английский
International Journal of Applied Earth Observation and Geoinformation, Journal Year: 2025, Volume and Issue: 136, P. 104357 - 104357
Published: Jan. 14, 2025
Language: Английский
Citations
2Geomatics Natural Hazards and Risk, Journal Year: 2025, Volume and Issue: 16(1)
Published: Feb. 13, 2025
Language: Английский
Citations
1Journal of Hydrology, Journal Year: 2025, Volume and Issue: unknown, P. 132842 - 132842
Published: Feb. 1, 2025
Language: Английский
Citations
0Physical Geography, Journal Year: 2025, Volume and Issue: unknown, P. 1 - 29
Published: Feb. 22, 2025
Language: Английский
Citations
0Remote Sensing, Journal Year: 2025, Volume and Issue: 17(9), P. 1540 - 1540
Published: April 26, 2025
Flooding is one of the most devastating natural disasters worldwide, with increasing frequency due to climate change. Traditional hydrological models require extensive data and computational resources, while machine learning (ML) struggle capture spatial dependencies. To address this, we propose a modified U-Net architecture that integrates prior knowledge permanent water bodies improve flood susceptibility mapping in Northumberland County, UK. By embedding domain-specific insights, our model achieves higher area under curve (AUC) (0.97) compared standard (0.93), also reducing training time by converging three times faster. Additionally, integrate Grad-CAM module provide visualisations explaining areas attention from model, enabling interpretation its decision-making, thus barriers practical implementation.
Language: Английский
Citations
0npj Urban Sustainability, Journal Year: 2025, Volume and Issue: 5(1)
Published: May 3, 2025
Language: Английский
Citations
0Remote Sensing, Journal Year: 2025, Volume and Issue: 17(10), P. 1660 - 1660
Published: May 8, 2025
The frequency and magnitude of natural hazards have been steadily increasing, largely due to extreme weather events driven by climate change. These pose significant global challenges, underscoring the need for accurate prediction models systematic preparedness. This study aimed predict multiple in South Korea using various machine learning algorithms. area, (100,210 km2), was divided into a grid system with 0.01° resolution. Meteorological, climatic, topographical, remotely sensed data were interpolated each cell analysis. focused on three major hazards: drought, flood, wildfire. Predictive developed two algorithms: Random Forest (RF) Extreme Gradient Boosting (XGB). analysis showed that XGB performed exceptionally well predicting droughts floods, achieving ROC scores 0.9998 0.9999, respectively. For wildfire prediction, RF achieved high score 0.9583. results integrated generate multi-hazard susceptibility map. provides foundational development hazard management response strategies context Furthermore, it offers basis future research exploring interaction effects multi-hazards.
Language: Английский
Citations
0Journal of Environmental Management, Journal Year: 2025, Volume and Issue: 387, P. 125670 - 125670
Published: May 19, 2025
Language: Английский
Citations
0Physics and Chemistry of the Earth Parts A/B/C, Journal Year: 2024, Volume and Issue: unknown, P. 103772 - 103772
Published: Oct. 1, 2024
Language: Английский
Citations
2Geomatics Natural Hazards and Risk, Journal Year: 2024, Volume and Issue: 16(1)
Published: Dec. 23, 2024
With the acceleration of climate change and urbanization, impacts floods on Chinese cities have become increasingly severe, improving urban flood resilience has an urgent issue. Although scholars in China proposed many methods to evaluate last decade, research this field not been critically reviewed thoroughly detail. Therefore, study selects high-quality original papers from previous decade focuses analyzing location, framework, data, analytical methods, limitations current assessment methods. The study's main objective is inform identification a system applicable context while revealing existing methodology's shortcomings data accuracy quality, consideration regional variability, indicator validity. Finally, finds significant challenges eight areas: talent pool, public participation, investment financing mechanisms. It provides targeted strategies, such as building multi-sectoral synergistic governance mechanism.
Language: Английский
Citations
1