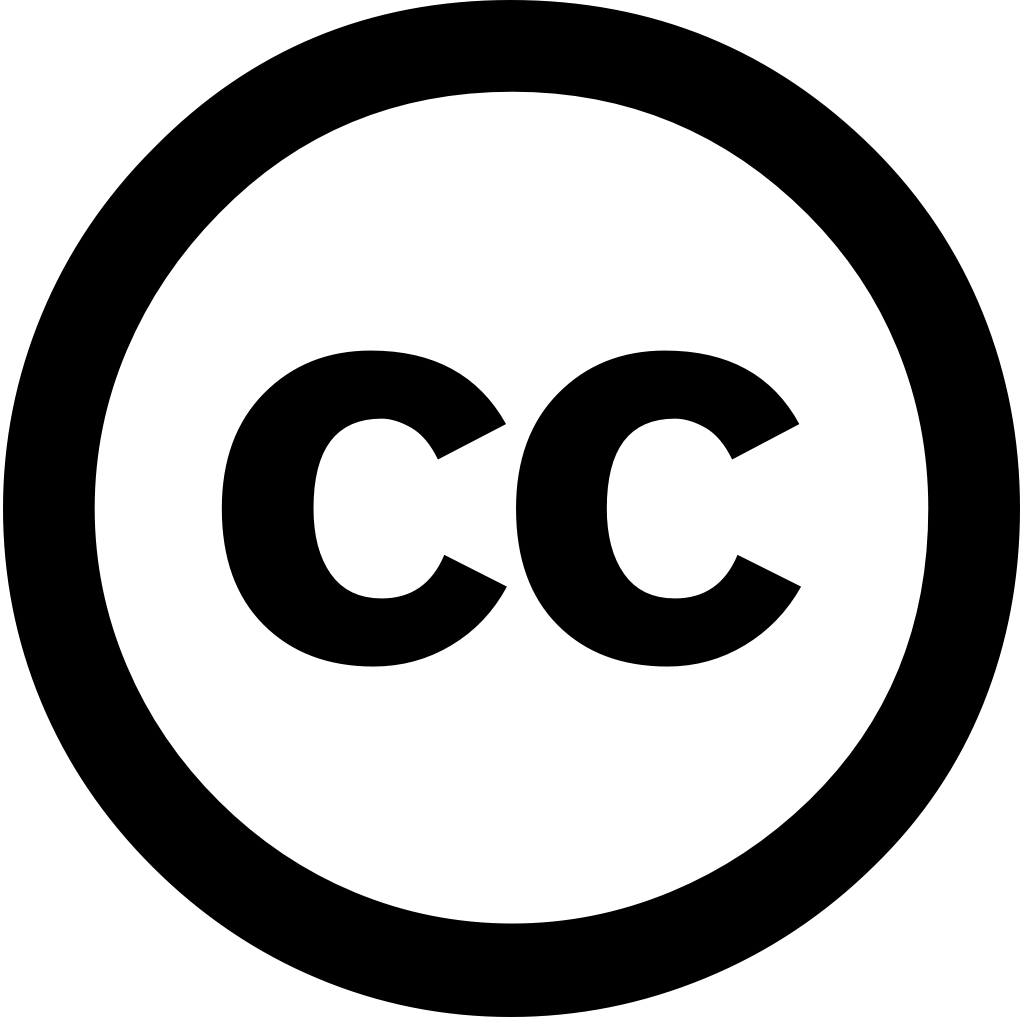
Published: Aug. 18, 2023
Language: Английский
Published: Aug. 18, 2023
Language: Английский
Academic Radiology, Journal Year: 2024, Volume and Issue: 31(9), P. 3801 - 3810
Published: Feb. 23, 2024
Language: Английский
Citations
8Rheumatology Science and Practice, Journal Year: 2025, Volume and Issue: 63(1), P. 24 - 36
Published: March 2, 2025
The article discusses the modern trends in development of digital technologies medicine, exemplified by rheumatology, especially, significance radiomics, which combines radiology, mathematical modeling, and deep machine learning. Texture analysis computed tomography images other imaging methods provides a more deeply characterization pathophysiological features tissues can be considered as non-invasive “virtual biopsy”. It is shown that radiomics enhances quality diagnostic predictive modeling. potential application radiomic models for studying predicting chest organ lesions various pathological conditions, including immune mediated inflammatory diseases, systemic vasculitis. Progress diagnosis treatment rheumatic diseases may facilitated integration omics technologies. era, opens up vast prospects advancements will undoubtedly require complex solutions to new technical, legal, ethical challenges.
Language: Английский
Citations
0Academic Radiology, Journal Year: 2023, Volume and Issue: 31(4), P. 1686 - 1697
Published: Oct. 5, 2023
Language: Английский
Citations
9Academic Radiology, Journal Year: 2024, Volume and Issue: 31(8), P. 3427 - 3437
Published: March 7, 2024
•We employed a cascading approach of two 2D networks to achieve the functionality 3D image pulmonary nodules super-resolution target reconstruction CT among three axes.•We used pre-trained model for mapping from normal-resolution images high-resolution images, which enhanced stability and convergence speed results.•In addition evaluation we also relevant diagnostic indicators, makes our results more valuable clinical application. Rationale ObjectivesTo develop Dual generative-adversarial-network (GAN) Cascaded Network (DGCN) generating computed tomography (SRCT) (NRCT) evaluate performance DGCN in multi-center datasets.Materials MethodsThis retrospective study included 278 patients with chest hospitals between January 2020 June 2023, each patient had all NRCT (512 × 512 matrix resolution 0.70 mm, mm,1.0 mm), (HRCT, 1024 0.35 ultra-high-resolution (UHRCT, 0.17 0.5 mm) examinations. Initially, deep residual network (DCRN) was built generate HRCT NRCT. Subsequently, DCRN as training further enhance along axes, ultimately yielding SRCT. PSNR, SSIM, FID, subjective scores, objective parameters related nodule segmentation testing set were recorded analyzed.ResultsDCRN obtained PSNR 52.16, SSIM 0.9941, FID 137.713, an average diameter difference 0.0981 mm. 46.50, 0.9990, 166.421, mm on 39 cases. There no significant differences SRCT UHRCT evaluation.ConclusionOur exhibited enhancement outperformed established methods regarding quality accuracy across both internal external datasets. To This analyzed. evaluation. Our
Language: Английский
Citations
1Academic Radiology, Journal Year: 2024, Volume and Issue: 31(10), P. 4244 - 4255
Published: April 15, 2024
Language: Английский
Citations
1Clinical Imaging, Journal Year: 2024, Volume and Issue: 114, P. 110275 - 110275
Published: Sept. 2, 2024
Language: Английский
Citations
1Academic Radiology, Journal Year: 2024, Volume and Issue: 32(2), P. 1067 - 1077
Published: Sept. 21, 2024
Language: Английский
Citations
1F1000Research, Journal Year: 2023, Volume and Issue: 12, P. 1319 - 1319
Published: Dec. 1, 2023
Language: Английский
Citations
2F1000Research, Journal Year: 2023, Volume and Issue: 12, P. 1319 - 1319
Published: Oct. 12, 2023
Language: Английский
Citations
1Journal of Computer Assisted Tomography, Journal Year: 2024, Volume and Issue: unknown
Published: Aug. 12, 2024
Objective To evaluate the methodological quality and predictive performance of artificial intelligence (AI) for predicting programmed death ligand 1 (PD-L1) expression epidermal growth factor receptors (EGFR) mutations in lung cancer (LC) based on systematic review meta-analysis. Methods AI studies PET/CT, CT, PET, immunohistochemistry (IHC)–whole-slide image (WSI) were included to predict PD-L1 or EGFR LC. The modified Quality Assessment Diagnostic Accuracy Studies (QUADAS-2) tool was used quality. A comprehensive meta-analysis conducted analyze overall area under curve (AUC). Cochrane diagnostic test I 2 statistics assess heterogeneity Results total 45 included, which 10 35 mutations. Based analysis using QUADAS-2 tool, 37 achieved a high-quality score 7. In levels, AUCs IHC-WSI 0.80 (95% confidence interval [CI], 0.77–0.84), 0.74 CI, 0.69–0.77), 0.95 0.93–0.97), respectively. For mutation status, PET 0.85 0.81–0.88), 0.83 0.80–0.86), 0.75 0.71–0.79), Test revealed an value exceeding 50%, indicating substantial meta-analyses. When combined with clinicopathological features, enhancement not substantial, whereas prediction showed improvement compared CT models, albeit significantly so PET/CT models. Conclusions LC has promising clinical implications.
Language: Английский
Citations
0