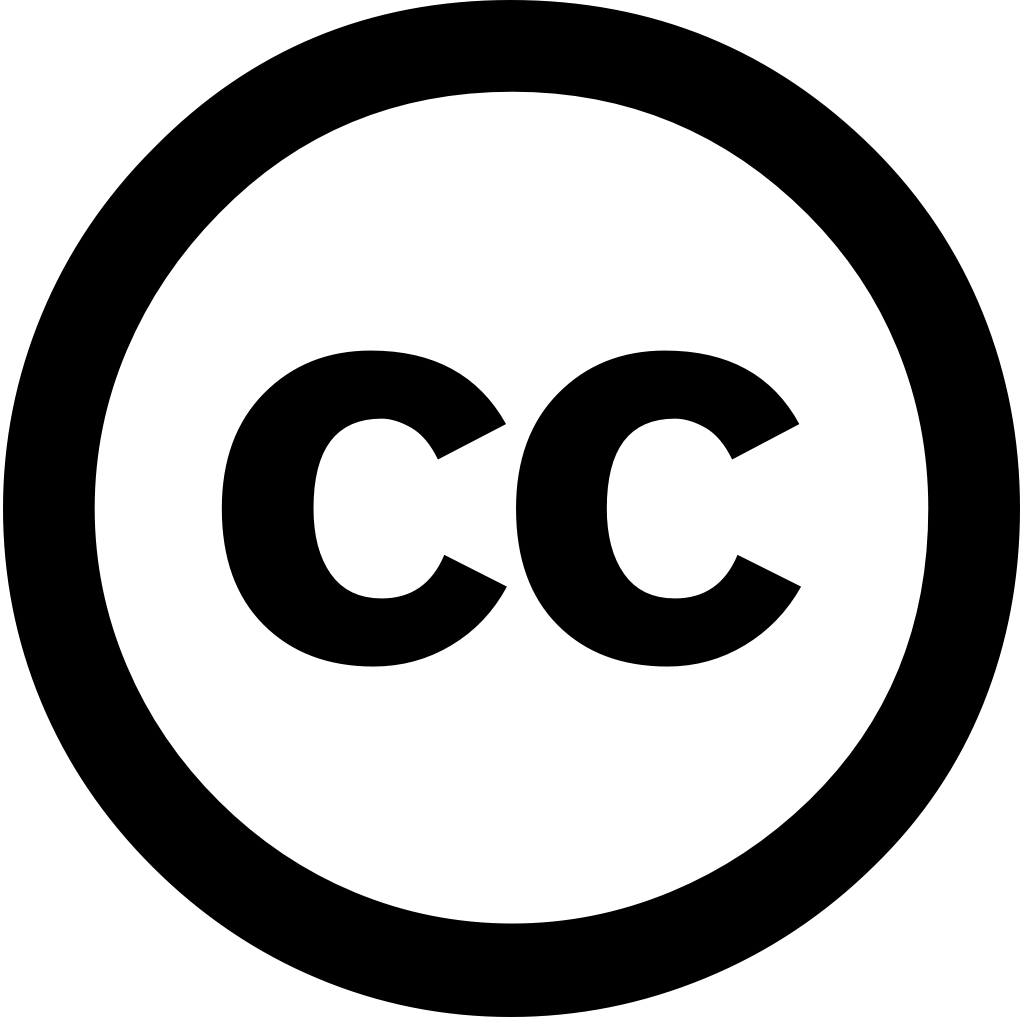
Radiotherapy and Oncology, Journal Year: 2024, Volume and Issue: 203, P. 110667 - 110667
Published: Dec. 13, 2024
Language: Английский
Radiotherapy and Oncology, Journal Year: 2024, Volume and Issue: 203, P. 110667 - 110667
Published: Dec. 13, 2024
Language: Английский
Annals of Surgical Treatment and Research, Journal Year: 2025, Volume and Issue: 108(3), P. 135 - 135
Published: Jan. 1, 2025
During transoral endoscopic thyroidectomy, preserving the recurrent laryngeal nerve (RLN) is a major challenge because visualization of this often obstructed by thyroid itself, increasing risk serious complications. This study explores application an augmented reality (AR) system to facilitate easier identification RLN during thyroidectomy. Three patients scheduled for thyroidectomy were enrolled in proof-of-concept study. Preoperative computed tomography scans used create AR model that included thyroid, trachea, veins, arteries, and RLN. The was overlaid onto real-time camera images live surgeries. Manual registration performed using customized controller. aligned with surgical landmarks such as trachea common carotid artery. accuracy assessed Dice similarity coefficient (DSC) evaluate alignment between real model. 3 female (mean age, 33.3 ± 15.7 years), mean tumor size 1.0 0.3 cm. All underwent right lobe. Final histopathological diagnoses comprised 2 papillary carcinomas one follicular adenoma. manual 0.60, 0.70, 0.57 1, 2, 3, respectively, value 0.6 0.1. proved feasible demonstrated potential improving localization anatomical structures, particularly RLN, indicated moderate DSC.
Language: Английский
Citations
0Information, Journal Year: 2025, Volume and Issue: 16(3), P. 215 - 215
Published: March 11, 2025
As yet, there is no systematic review focusing on benefits and issues of commercial deep learning-based auto-segmentation (DLAS) software for prostate cancer (PCa) radiation therapy (RT) planning despite that NRG Oncology has underscored such necessity. This article’s purpose to systematically DLAS product performances PCa RT their associated evaluation methodology. A literature search was performed with the use electronic databases 7 November 2024. Thirty-two articles were included as per selection criteria. They evaluated 12 products (Carina Medical LLC INTContour (Lexington, KY, USA), Elekta AB ADMIRE (Stockholm, Sweden), Limbus AI Inc. Contour (Regina, SK, Canada), Manteia Technologies Co. AccuContour (Jian Sheng, China), MIM Software ProtégéAI (Cleveland, OH, Mirada Ltd. DLCExpert (Oxford, UK), MVision.ai Contour+ (Helsinki, Finland), Radformation AutoContour (New York, NY, RaySearch Laboratories RayStation Siemens Healthineers AG AI-Rad Companion Organs RT, syngo.via Image Suite DirectORGANS (Erlangen, Germany), Therapanacea Annotate (Paris, France), Varian Systems, Ethos (Palo Alto, CA, USA)). Their results illustrate can delineate organs at risk (abdominopelvic cavity, anal canal, bladder, body, cauda equina, left (L) right (R) femurs, L R pelvis, proximal sacrum) four clinical target volumes (prostate, lymph nodes, bed, seminal vesicle bed) clinically acceptable outcomes, resulting in delineation time reduction, 5.7–81.1%. Although recommended each centre perform its own prior implementation, seems more important due methodological respective single studies, e.g., small dataset used, etc.
Language: Английский
Citations
0Advances in Radiation Oncology, Journal Year: 2025, Volume and Issue: 10(4), P. 101749 - 101749
Published: April 1, 2025
Language: Английский
Citations
0Cancers, Journal Year: 2024, Volume and Issue: 16(17), P. 2988 - 2988
Published: Aug. 28, 2024
In spinal oncology, integrating deep learning with computed tomography (CT) imaging has shown promise in enhancing diagnostic accuracy, treatment planning, and patient outcomes. This systematic review synthesizes evidence on artificial intelligence (AI) applications CT for tumors. A PRISMA-guided search identified 33 studies: 12 (36.4%) focused detecting malignancies, 11 (33.3%) classification, 6 (18.2%) prognostication, 3 (9.1%) 1 (3.0%) both detection classification. Of the classification studies, 7 (21.2%) used machine to distinguish between benign malignant lesions, evaluated tumor stage or grade, 2 (6.1%) employed radiomics biomarker Prognostic studies included three that predicted complications such as pathological fractures AI's potential improving workflow efficiency, aiding decision-making, reducing is discussed, along its limitations generalizability, interpretability, clinical integration. Future directions AI oncology are also explored. conclusion, while technologies promising, further research necessary validate their effectiveness optimize integration into routine practice.
Language: Английский
Citations
2Deleted Journal, Journal Year: 2024, Volume and Issue: 1(1)
Published: Jan. 1, 2024
Abstract Radiation therapy is a localized cancer treatment that relies on precise delineation of the target to be treated and healthy tissues guarantee optimal effect. This step, known as contouring or segmentation, involves identifying both volumes organs at risk imaging modalities like CT, PET, MRI guide radiation delivery. Manual however, time-consuming highly subjective, despite presence guidelines. In recent years, automated segmentation methods, particularly deep learning models, have shown promise in addressing this task. However, challenges persist their clinical use, including need for robust quality assurance (QA) processes risks associated with use models. review examines considerations adoption auto-segmentation radiotherapy, focused volume. We discuss potential (eg, over- under-segmentation, automation bias, appropriate trust), mitigation strategies human oversight, uncertainty quantification, education professionals), we highlight importance expanding QA include geometric, dose-volume, outcome-based performance monitoring. While offers significant benefits, careful attention rigorous measures are essential its successful integration practice.
Language: Английский
Citations
2Physics in Medicine and Biology, Journal Year: 2024, Volume and Issue: 69(20), P. 205013 - 205013
Published: Sept. 27, 2024
To demonstrate the feasibility of integrating fully-automated online adaptive proton therapy strategies (OAPT) within a commercially available treatment planning system and underscore what limits their clinical implementation. These leverage existing deformable image registration (DIR) algorithms state-of-the-art deep learning (DL) networks for organ segmentation dose prediction.
Language: Английский
Citations
1Multimodal Technologies and Interaction, Journal Year: 2024, Volume and Issue: 8(12), P. 114 - 114
Published: Dec. 20, 2024
As yet, no systematic review on commercial deep learning-based auto-segmentation (DLAS) software for breast cancer radiation therapy (RT) planning has been published, although NRG Oncology highlighted the necessity such. The purpose of this is to investigate performances DLAS packages RT and methods their performance evaluation. A literature search was conducted with use electronic databases. Fifteen papers met selection criteria were included. included studies evaluated eight (Limbus Contour, Manteia AccuLearning, Mirada DLCExpert, MVision.ai Contour+, Radformation AutoContour, RaySearch RayStation, Siemens syngo.via Image Suite/AI-Rad Companion Organs RT, Therapanacea Annotate). Their findings show that could contour ten organs at risk (body, contralateral breast, esophagus-overlapping area, heart, ipsilateral humeral head, left right lungs, liver, sternum trachea) three clinical target volumes (CTVp_breast, CTVp_chestwall, CTVn_L1) up clinically acceptable standard. This can contribute 45.4%–93.7% contouring time reduction per patient. Although NRO suggested every center should conduct its own evaluation before implementation, such testing appears particularly crucial Contour+ as a result methodological weaknesses corresponding studies, small datasets collected retrospectively from single centers
Language: Английский
Citations
1Forum, Journal Year: 2024, Volume and Issue: 39(4), P. 264 - 268
Published: Aug. 8, 2024
Citations
0Research Square (Research Square), Journal Year: 2024, Volume and Issue: unknown
Published: Sept. 3, 2024
Language: Английский
Citations
0Published: Oct. 23, 2024
Language: Английский
Citations
0