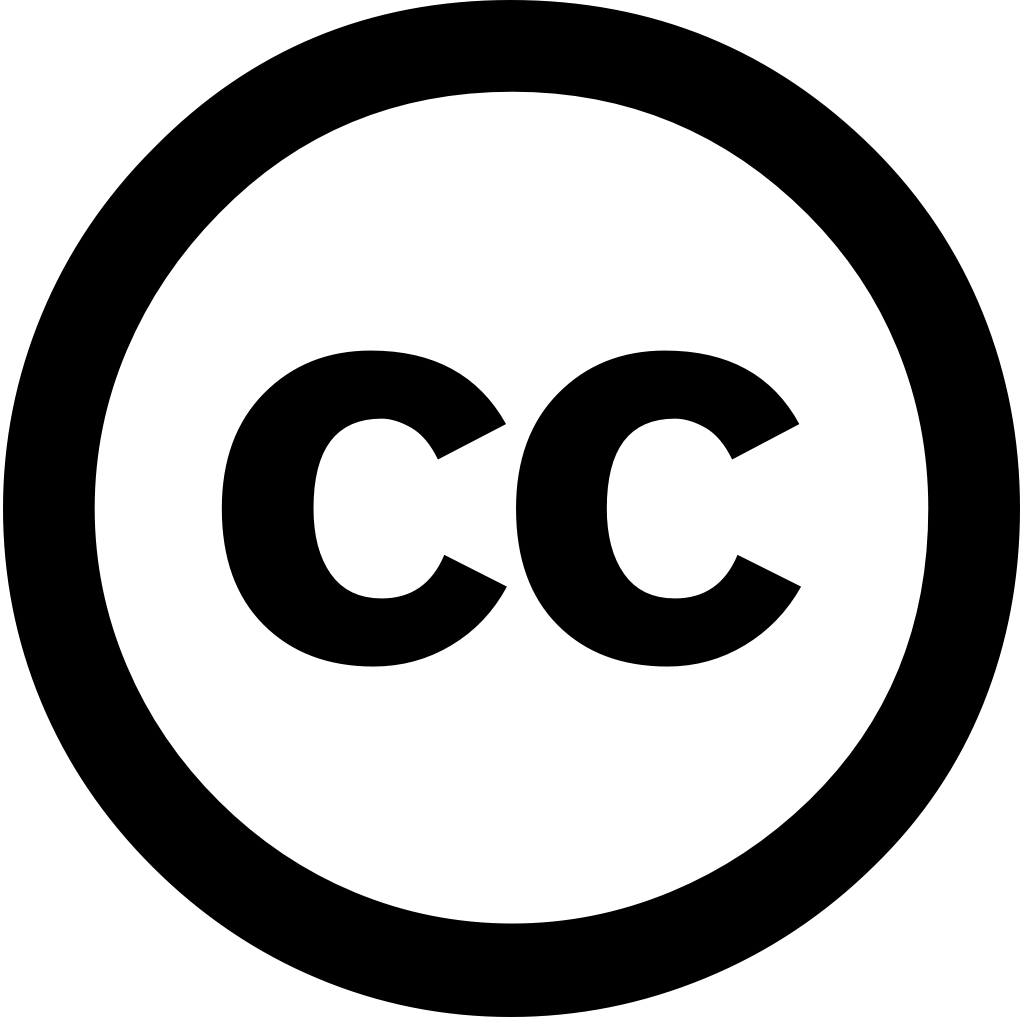
Deleted Journal, Journal Year: 2024, Volume and Issue: 6(9)
Published: Sept. 9, 2024
Language: Английский
Deleted Journal, Journal Year: 2024, Volume and Issue: 6(9)
Published: Sept. 9, 2024
Language: Английский
Journal Of Big Data, Journal Year: 2024, Volume and Issue: 11(1)
Published: Aug. 4, 2024
Abstract As the number and cleverness of cyber-attacks keep increasing rapidly, it's more important than ever to have good ways detect prevent them. Recognizing cyber threats quickly accurately is crucial because they can cause severe damage individuals businesses. This paper takes a close look at how we use artificial intelligence (AI), including machine learning (ML) deep (DL), alongside metaheuristic algorithms better. We've thoroughly examined over sixty recent studies measure effective these AI tools are identifying fighting wide range threats. Our research includes diverse array cyberattacks such as malware attacks, network intrusions, spam, others, showing that ML DL methods, together with algorithms, significantly improve well find respond We compare methods out what they're where could improve, especially face new changing cyber-attacks. presents straightforward framework for assessing Methods in threat detection. Given complexity threats, enhancing regularly ensuring strong protection critical. evaluate effectiveness limitations current proposed models, addition algorithms. vital guiding future enhancements. We're pushing smart flexible solutions adapt challenges. The findings from our suggest protecting against will rely on continuously updating stay ahead hackers' latest tricks.
Language: Английский
Citations
22Journal of King Saud University - Computer and Information Sciences, Journal Year: 2023, Volume and Issue: 35(10), P. 101820 - 101820
Published: Nov. 6, 2023
The Internet of Things (IoT) has transformed many aspects modern life, from healthcare and transportation to home automation industrial control systems. However, the increasing number connected devices also led an increase in security threats, particularly botnets. To mitigate these various machine learning (ML) deep (DL) techniques have been proposed for IoT botnet attack detection. This systematic review aims identify most effective ML DL detecting botnets by delving into benchmark datasets, evaluation metrics, data pre-processing detail. A comprehensive search was conducted multiple databases primary studies published between 2018 2023. Studies were included if they reported use or After screening 1,567 records, 25 final review. findings suggest that show promising results attacks, outperforming traditional signature-based methods. effectiveness varied depending on dataset, features, metrics used. Based synthesis findings, this proposes a taxonomy detection provides recommendations future research area. illuminates considerable potential approaches bolstering thereby offering valuable insights researchers involved domain security.
Language: Английский
Citations
28Scientific Reports, Journal Year: 2024, Volume and Issue: 14(1)
Published: April 3, 2024
Abstract The rapid expansion of AI-enabled Internet Things (IoT) devices presents significant security challenges, impacting both privacy and organizational resources. dynamic increase in big data generated by IoT poses a persistent problem, particularly making decisions based on the continuously growing data. To address this challenge environment, study introduces specialized BERT-based Feed Forward Neural Network Framework (BEFNet) designed for scenarios. In evaluation, novel framework with distinct modules is employed thorough analysis 8 datasets, each representing different type malware. BEFSONet optimized using Spotted Hyena Optimizer (SO), highlighting its adaptability to diverse shapes malware Thorough exploratory analyses comparative evaluations underscore BEFSONet’s exceptional performance metrics, achieving 97.99% accuracy, 97.96 Matthews Correlation Coefficient, 97% F1-Score, 98.37% Area under ROC Curve(AUC-ROC), 95.89 Cohen’s Kappa. This research positions as robust defense mechanism era security, offering an effective solution evolving challenges decision-making environments.
Language: Английский
Citations
10PLoS ONE, Journal Year: 2024, Volume and Issue: 19(2), P. e0299334 - e0299334
Published: Feb. 29, 2024
This research addresses the pressing challenge of intrusion detection and prevention in Wireless Sensor Networks (WSNs), offering an innovative comprehensive approach. The leverages Support Vector Regression (SVR) models to predict number barriers necessary for effective while optimising their strategic placement. paper employs Ant Colony Optimization (ACO) algorithm enhance precision barrier placement resource allocation. integrated approach combines SVR predictive modelling with ACO-based optimisation, contributing advancing adaptive security solutions WSNs. Feature ranking highlights critical influence count attributes, regularisation techniques are applied model robustness. Importantly, results reveal substantial percentage improvements accuracy metrics: a 4835.71% reduction Mean Squared Error (MSE) ACO-SVR1, 862.08% improvement Absolute (MAE) 86.29% enhancement R-squared (R2) ACO-SVR1. ACO-SVR2 has 2202.85% MSE, 733.98% MAE, 54.03% R-squared. These considerable verify method's effectiveness enhancing WSNs, ensuring reliability resilience infrastructure. concludes performance comparison emphasises remarkable efficacy regularisation. It also underscores practicality precise estimation optimised placement, WSNs against potential threats.
Language: Английский
Citations
8International Journal of Computational and Experimental Science and Engineering, Journal Year: 2025, Volume and Issue: 11(1)
Published: Feb. 28, 2025
Internet of Things (IoT) applications have made inroads into different domains, providing unique solutions—Internet technology offers seamless integration physical and digital worlds. However, the broad nature technologies protocols used in IoT has increased vulnerability from malicious attackers. Hence, protecting cyber-attacks is imperative. Researchers implemented intrusion detection systems to overcome this issue improve cybersecurity scenarios. With new threats cybercrime emerging, a continuous effort required enhance security applications. To address pressing need, we present our study that proposes deep learning-based framework bolster at use cases level by exploiting power transfer learning ensembling it models pre-trained larger datasets. Deep attain high performance with help hyperparameter tuning, achieve through PSO proposed system. Our ensemble system shows how individual can outperform using best-performing as constituents approach. We introduce an algorithm called — Optimized Ensemble Learning-Based Intrusion Detection (OEL-ID). This leverages corresponding optimization strategies boost for improved cyber Using UNSW-NB15 benchmark dataset, empirical demonstrates method, compared some existing models, obtained accuracy 98.89%, which, turn, provided highest comparative accuracy. Therefore, be allows significant system's underlying
Language: Английский
Citations
1Internet of Things, Journal Year: 2024, Volume and Issue: unknown, P. 101361 - 101361
Published: Sept. 1, 2024
Language: Английский
Citations
6The Journal of Supercomputing, Journal Year: 2023, Volume and Issue: 80(6), P. 7876 - 7905
Published: Nov. 10, 2023
Language: Английский
Citations
14Mathematics, Journal Year: 2023, Volume and Issue: 11(19), P. 4080 - 4080
Published: Sept. 26, 2023
The Internet of Things (IoT) is the most extensively utilized technology nowadays that simple and has advantage replacing data with other devices by employing cloud or wireless networks. However, cyber-threats cyber-attacks significantly affect smart applications on these IoT platforms. effects intrusions lead to economic physical damage. conventional security approaches are unable handle current problems since threats attacks continuously evolving. In this background, Artificial Intelligence (AI) knowledge, particularly Machine Learning (ML) Deep (DL) solutions, remains key delivering a dynamically improved modern system for next-generation systems. Therefore, manuscript designs Honey Badger Algorithm an Optimal Hybrid Belief Network (HBA-OHDBN) technique cyberattack detection in blockchain (BC)-assisted environment. purpose proposed HBA-OHDBN algorithm lies its accurate recognition classification cyberattacks BC-assisted platform. technique, feature selection using HBA implemented choose optimal set features. For intrusion detection, applies HDBN model. order adjust hyperparameter values model, Dung Beetle Optimization (DBO) utilized. Moreover, BC also applied improve network security. performance was validated benchmark NSLKDD dataset. extensive results indicate model outperforms recent models, maximum accuracy 99.21%.
Language: Английский
Citations
13Journal of Reliable Intelligent Environments, Journal Year: 2025, Volume and Issue: 11(2)
Published: April 9, 2025
Language: Английский
Citations
0Ad Hoc Networks, Journal Year: 2025, Volume and Issue: unknown, P. 103869 - 103869
Published: April 1, 2025
Language: Английский
Citations
0