Deep Learning-Based Intrusion Detection for IoT Networks: A Scalable and Efficient Approach
Abstract
The
rapid
expansion
of
the
Internet
Things
(IoT)
has
revolutionized
industries
by
enabling
seamless
connectivity,
but
it
also
introduced
significant
security
vulnerabilities,
making
IoT
networks
prime
targets
for
cyberattacks.
Traditional
intrusion
detection
systems
often
struggle
to
cope
with
high
volume
and
dynamic
nature
traffic,
necessitating
development
more
robust
intelligent
mechanisms.
This
research
presents
a
deep
learning-based
approach
real-time
threat
in
networks,
leveraging
advanced
models
such
as
1D
Convolutional
Neural
Networks
(CNNs),
Long
Short-Term
Memory
(LSTM)
Recurrent
(RNNs),
Multi-Layer
Perceptrons
(MLPs)
enhance
detection.
study
utilizes
CIC
IoT-DIAD
2024
dataset,
comprehensive
collection
flow-based
network
traffic
containing
both
benign
attack
scenarios.
proposed
were
trained
evaluated
on
feature
sets,
optimizing
hyperparameters
maximize
accuracy,
recall,
F1-score.
In
multi-class
classification,
CNN
achieved
highest
accuracy
99.12%,
followed
LSTM
(98.98%),
RNN
(98.43%),
MLP
(97.21%).
For
binary
anomaly
detection,
again
demonstrated
superior
performance
an
99.53%,
while
LSTM,
RNN,
99.52%,
99.25%,
98.78%,
respectively.
results
indicate
that
is
most
effective
model
excelling
extraction
classification.
findings
contribute
scalable
efficient
solutions,
improving
ability
detect
mitigate
cyber
threats
environments.
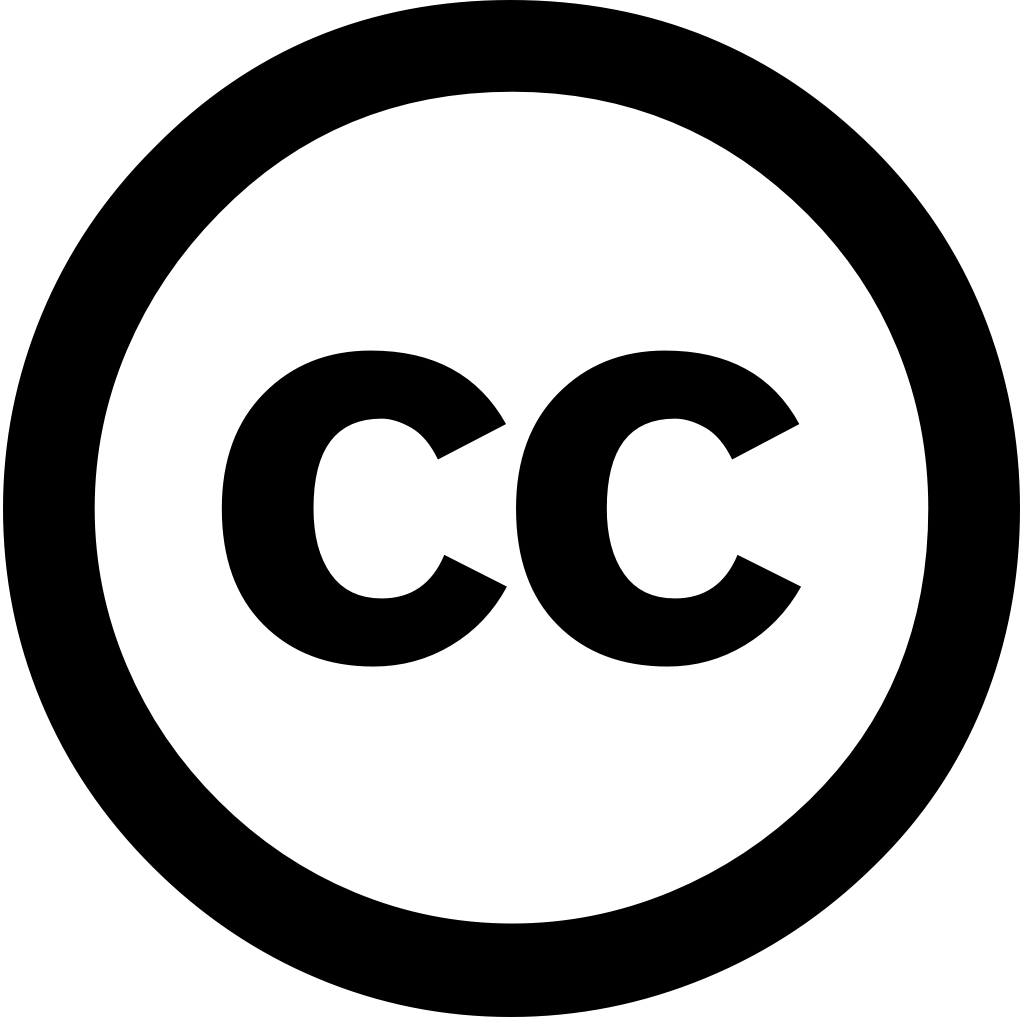
Research Square (Research Square), Journal Year: 2025, Volume and Issue: unknown
Published: March 26, 2025
Language: Английский