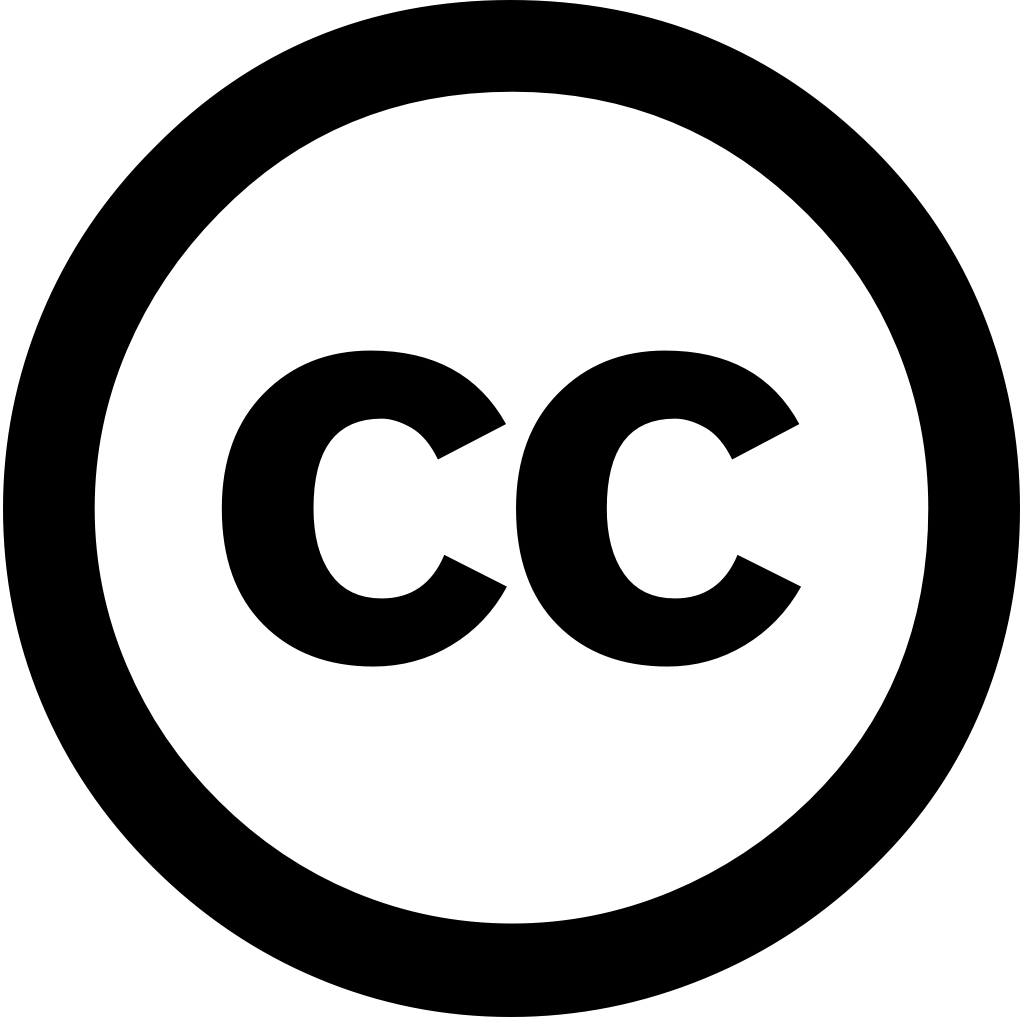
Journal of Hydrology Regional Studies, Journal Year: 2025, Volume and Issue: 58, P. 102271 - 102271
Published: Feb. 27, 2025
Language: Английский
Journal of Hydrology Regional Studies, Journal Year: 2025, Volume and Issue: 58, P. 102271 - 102271
Published: Feb. 27, 2025
Language: Английский
Environmental Modelling & Software, Journal Year: 2024, Volume and Issue: 174, P. 105971 - 105971
Published: Feb. 10, 2024
Language: Английский
Citations
77Agricultural Water Management, Journal Year: 2023, Volume and Issue: 280, P. 108232 - 108232
Published: Feb. 15, 2023
In years of increasing impact climate change effects, a reliable characterization the spatiotemporal evolutionary dynamics evapotranspiration can enable significant improvement in water resource management, especially as regards irrigation activities. Sicily, an insular region Southern Italy, has exceptionally valuable agricultural production and high needs. this study, ETo reference Sicily was first evaluated on basis historical future parameters, referring for values to two scenarios characterized by different Representative Concentration Pathways: RCP 4.5 8.5. Then, Hierarchical algorithm used divide into three homogeneous regions, each specific features. addition, some Machine Learning (ML) algorithms were develop forecasting models based only data. Support Vector Regression (SVR) predict Tmin Tmax, while ensemble model Multilayer Perceptron (MLP) M5P Tree developed forecasting. Predictions made with MLP-M5P compared computed 8.5 scenarios. During forecast period, from 2001 2091, increases observed all clusters. For cluster C1, along coast, percentage 7.52%, 14.64% 10.78%, 4.5, 8.5, MLP-M5P, respectively, while, C3, inland, higher equal 8.12%, 16.71%, 14.98%, respectively. The led intermediate trends between showing correlation latter (R2 0.93 0.98). approach, both clustering algorithms, provided comprehensive analysis evapotranspiration, detection regions and, at same time, evaluation trends, coastal inland areas.
Language: Английский
Citations
46Journal of Hydrology, Journal Year: 2024, Volume and Issue: 636, P. 131275 - 131275
Published: May 7, 2024
Language: Английский
Citations
17Applied Soft Computing, Journal Year: 2025, Volume and Issue: unknown, P. 112779 - 112779
Published: Jan. 1, 2025
Language: Английский
Citations
2Ocean Engineering, Journal Year: 2025, Volume and Issue: 323, P. 120590 - 120590
Published: Feb. 8, 2025
Language: Английский
Citations
2Computers and Electronics in Agriculture, Journal Year: 2023, Volume and Issue: 209, P. 107836 - 107836
Published: April 28, 2023
Language: Английский
Citations
41Agricultural Water Management, Journal Year: 2023, Volume and Issue: 279, P. 108175 - 108175
Published: Feb. 7, 2023
This paper established hybrid prediction models based on variational mode decomposition (VMD), empirical (EMD), and ensemble (EEMD) combined with the backpropagation neural network model (BPNN) to improve accuracy of reference crop evapotranspiration (ET0) time series characteristics nonlinearity instability. The daily ET0 data 11 representative stations in Xinjiang from 1993 2016 were selected for training testing compared results support vector regression (SVR) gradient boosting tree (GBRT) as two machine learning models. indicated superiority VMD-BPNN EMD-BPNN EEMD-BPNN terms stability, root mean square error (RMSE) = 0.405 mm/d, absolute (MAE) 0.268 coefficient determination (R2) 0.979. When employing forecast seven days, RMSE Nash-Sutcliffe efficiency (NSE) 0.588 mm/d 0.952, respectively, high precision reliability. was significantly higher than that single models, such BPNN, SVR, GBRT. MAE values more 60% smaller GBRT R2 NSE approximately 18% respectively. demonstrates effectiveness VMD method reducing non-stationarity original data. BPNN predicted decomposed series, stability enhanced. indicates reliability its capability Xinjiang.
Language: Английский
Citations
35Ecological Informatics, Journal Year: 2023, Volume and Issue: 75, P. 102122 - 102122
Published: May 9, 2023
Language: Английский
Citations
31Ecological Informatics, Journal Year: 2024, Volume and Issue: 80, P. 102455 - 102455
Published: Jan. 4, 2024
Developing reliable streamflow forecasting models is critical for hydrological tasks such as improving water resource management, analyzing river patterns, and flood forecasting. In this research, the first time, an emerging multi-level TOPSIS (technique order preference by similarity to ideal solution) based hybridization comprised of Boruta classification regression tree (Boruta-CART) feature selection, multivariate variational mode decomposition (MVMD), a hybrid Convolutional Neural Network (CNN) Bidirectional Gated Recurrent Unit (CNN-BiGRU) deep learning was adopted multi-temporal (one three days ahead) forecast daily in Rivers Prince Edward Island, Canada. For aim, step, Boruta-CART selection technique determines most effective lagged components among all antecedent two-day information (i.e., t-1 t-2) hydro-meteorological features (from 2015 2020), including level, mean air temperature, heat degree days, total precipitation, dew point relative humidity Bear Winter Afterwards, (MVMD) decomposes input time series decrease complexity non-linearity non-stationary ones before feeding (DL) models. Here, CNN-GRU employed primary DL model, along with kernel extreme machine method (KELM), random function link (RVFL), CNN bidirectional recurrent neural network (CNN-BiRNN) comparative A scheme applying several performance measures like correlation coefficient (R), root square error (RMSE), reliability designed robustness assessment (MVM-CNN-BiGRU, MVM-CNN-BiRNN, MVM-RVFL, MVM-KELM) standalone The computational outcomes revealed that River, MVM-CNN-BiGRU, owing its best day ahead: score 1, R = 0.960, RMSE 0.098, 65.082; 0.999, 0.924, 0.33) outperformed other models, followed MVM-KELM, respectively. Moreover, MVM-CNN-BiGRU terms (one-day 0.890, 0.955, 0.274, 34.004; three-days 0.686, 0.330) superior provided expert system could be vital local decision-making process, absence modeling, during seasons reduce damage residential areas.
Language: Английский
Citations
11Engineering Applications of Artificial Intelligence, Journal Year: 2024, Volume and Issue: 135, P. 108744 - 108744
Published: June 3, 2024
Language: Английский
Citations
9