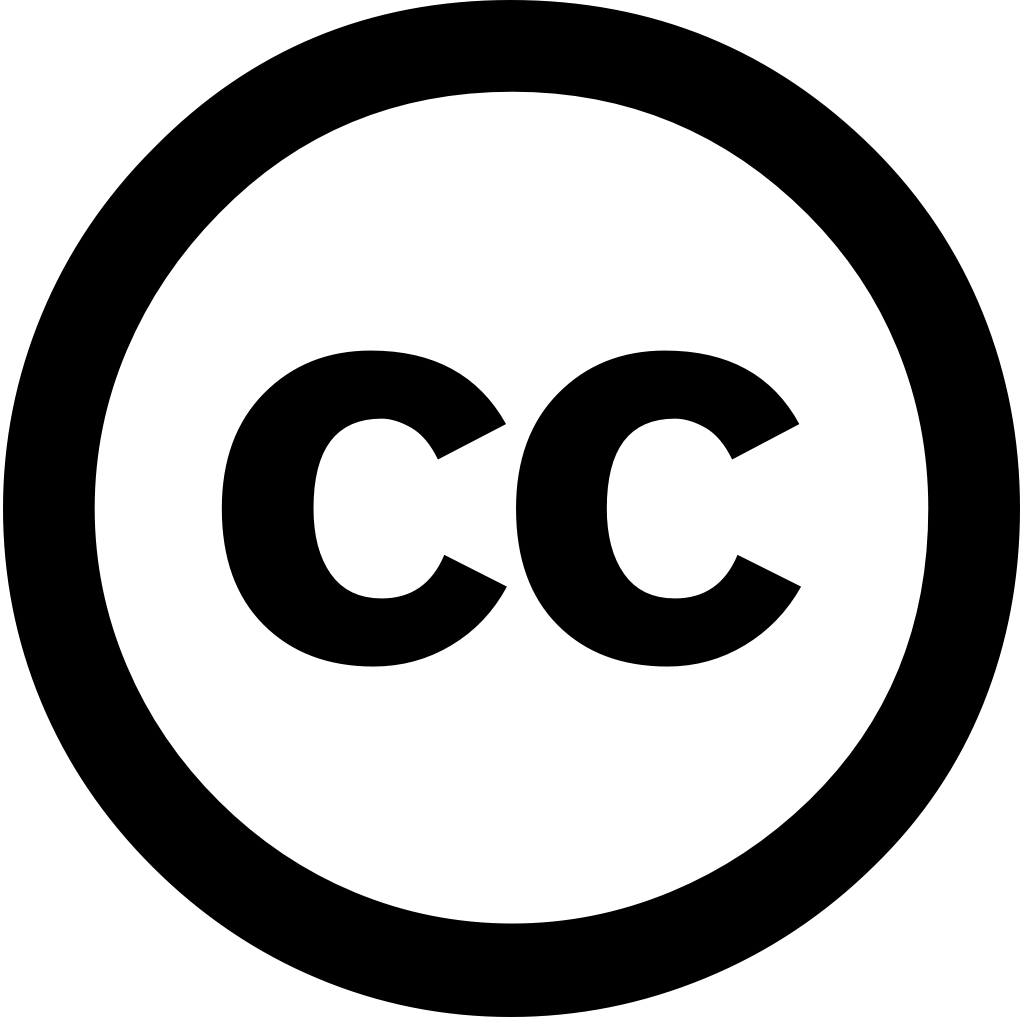
Journal of Hydrology Regional Studies, Journal Year: 2025, Volume and Issue: 58, P. 102271 - 102271
Published: Feb. 27, 2025
Language: Английский
Journal of Hydrology Regional Studies, Journal Year: 2025, Volume and Issue: 58, P. 102271 - 102271
Published: Feb. 27, 2025
Language: Английский
Results in Engineering, Journal Year: 2025, Volume and Issue: unknown, P. 104254 - 104254
Published: Feb. 1, 2025
Language: Английский
Citations
1Engineering Applications of Artificial Intelligence, Journal Year: 2022, Volume and Issue: 117, P. 105545 - 105545
Published: Nov. 10, 2022
Language: Английский
Citations
38Journal of Hydrology, Journal Year: 2022, Volume and Issue: 617, P. 128947 - 128947
Published: Dec. 14, 2022
Language: Английский
Citations
35Expert Systems with Applications, Journal Year: 2023, Volume and Issue: 237, P. 121512 - 121512
Published: Sept. 13, 2023
Language: Английский
Citations
22Journal of Hydrology, Journal Year: 2023, Volume and Issue: 620, P. 129418 - 129418
Published: March 22, 2023
Language: Английский
Citations
19Renewable and Sustainable Energy Reviews, Journal Year: 2023, Volume and Issue: 185, P. 113645 - 113645
Published: Aug. 15, 2023
Language: Английский
Citations
18Scientific Reports, Journal Year: 2024, Volume and Issue: 14(1)
Published: July 1, 2024
Electrical conductivity (EC) is widely recognized as one of the most essential water quality metrics for predicting salinity and mineralization. In current research, EC two Australian rivers (Albert River Barratta Creek) was forecasted up to 10 days using a novel deep learning algorithm (Convolutional Neural Network combined with Long Short-Term Memory Model, CNN-LSTM). The Boruta-XGBoost feature selection method used determine significant inputs (time series lagged data) model. To compare performance Boruta-XGB-CNN-LSTM models, three machine approaches-multi-layer perceptron neural network (MLP), K-nearest neighbour (KNN), extreme gradient boosting (XGBoost) were used. Different statistical metrics, such correlation coefficient (R), root mean square error (RMSE), absolute percentage error, assess models' performance. From years data in both rivers, 7 (2012-2018) training set, 3 (2019-2021) testing models. Application model forecasting day ahead showed that stations, can forecast parameter better than other models test dataset (R = 0.9429, RMSE 45.6896, MAPE 5.9749 Albert River, R 0.9215, 43.8315, 7.6029 Creek). Considering this 3-10 EC. results very capable next days. by increasing horizon from days, slightly decreased. study show be good soft computing accurately how will change rivers.
Language: Английский
Citations
8Earth Science Informatics, Journal Year: 2023, Volume and Issue: 16(3), P. 2475 - 2495
Published: July 19, 2023
Language: Английский
Citations
16Atmospheric Pollution Research, Journal Year: 2023, Volume and Issue: 14(6), P. 101752 - 101752
Published: April 20, 2023
Language: Английский
Citations
14Applied Computing and Geosciences, Journal Year: 2024, Volume and Issue: 24, P. 100206 - 100206
Published: Nov. 9, 2024
Language: Английский
Citations
5