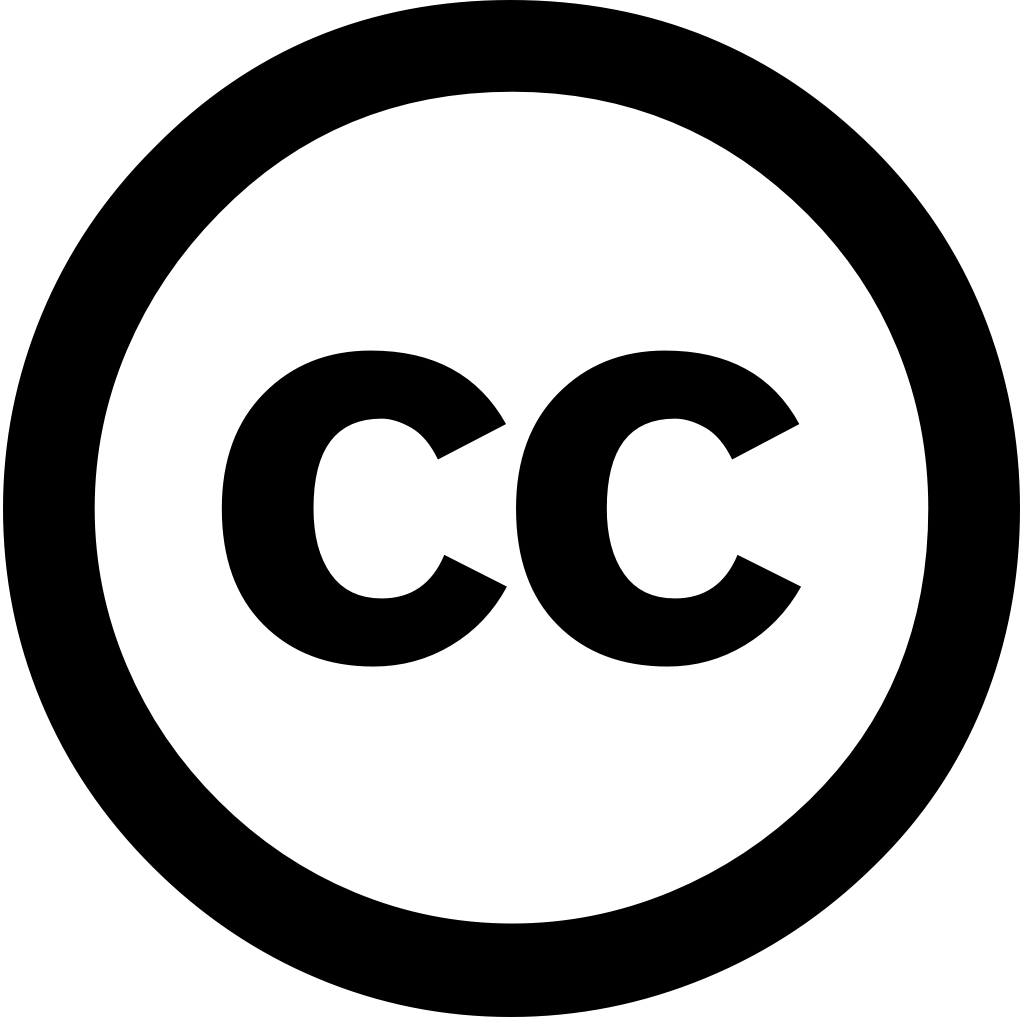
Research Square (Research Square), Journal Year: 2024, Volume and Issue: unknown
Published: Dec. 16, 2024
Language: Английский
Research Square (Research Square), Journal Year: 2024, Volume and Issue: unknown
Published: Dec. 16, 2024
Language: Английский
Sensors, Journal Year: 2025, Volume and Issue: 25(1), P. 288 - 288
Published: Jan. 6, 2025
The increasing demand for hazelnut kernels is favoring an upsurge in cultivation worldwide, but ongoing climate change threatens this crop, affecting yield decreases and subject to uncontrolled pathogen parasite attacks. Technical advances precision agriculture are expected support farmers more efficiently control the physio-pathological status of crops. Here, we report a straightforward approach monitoring trees open field, using aerial multispectral pictures taken by drones. A dataset 4112 images, each having 2Mpixel resolution per tree covering RGB, Red Edge, near-infrared frequencies, was obtained from 185 located two different orchards Piedmont region (northern Italy). To increase accuracy, especially reduce false negatives, image divided into nine quadrants. For quadrant, vegetation indices (VIs) were computed, parallel, quadrant tagged as “healthy/unhealthy” visual inspection. Three supervised binary classification algorithms used build models capable predicting VIs predictors. Out considered, only five (GNDVI, GCI, NDREI, NRI, GI) good predictors, while NDVI SAVI, RECI, TCARI not. Using them, model accuracy about 65%, with 13% negatives reached way that rather independent algorithms, demonstrating some allow inferring condition these trees. These achievements use drone-captured images performing rapid, non-destructive physiological characterization This offers sustainable strategy supporting their decision-making process during agricultural practices.
Language: Английский
Citations
1Published: July 15, 2024
Language: Английский
Citations
1Research Square (Research Square), Journal Year: 2024, Volume and Issue: unknown
Published: Dec. 16, 2024
Language: Английский
Citations
0