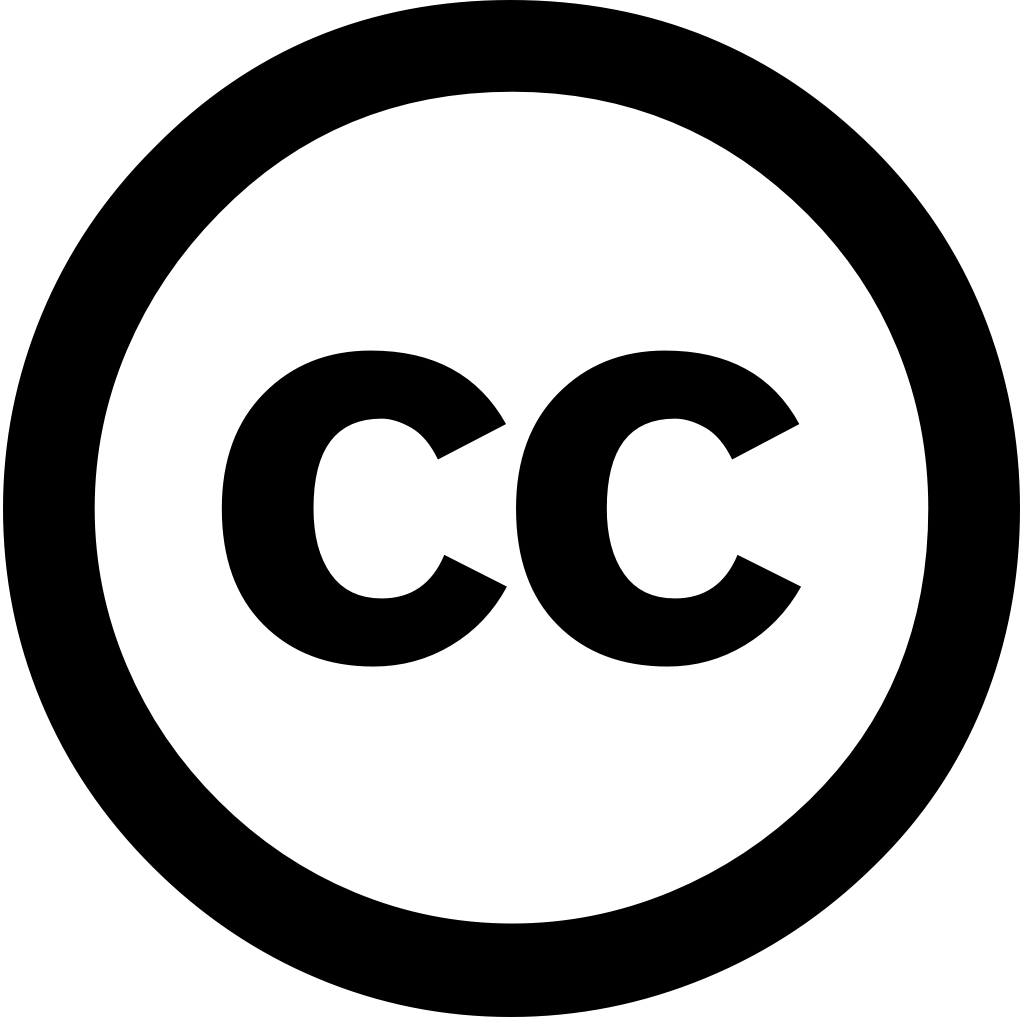
Ain Shams Engineering Journal, Journal Year: 2024, Volume and Issue: 16(1), P. 103227 - 103227
Published: Dec. 26, 2024
Language: Английский
Ain Shams Engineering Journal, Journal Year: 2024, Volume and Issue: 16(1), P. 103227 - 103227
Published: Dec. 26, 2024
Language: Английский
Plants, Journal Year: 2025, Volume and Issue: 14(5), P. 653 - 653
Published: Feb. 21, 2025
The automated recognition of disease in tomato leaves can greatly enhance yield and allow farmers to manage challenges more efficiently. This study investigates the performance YOLOv11 for leaf recognition. All accessible versions were first fine-tuned on an improved dataset consisting a healthy class 10 classes. YOLOv11m was selected further hyperparameter optimization based its evaluation metrics. It achieved fitness score 0.98885, with precision 0.99104, recall 0.98597, [email protected] 0.99197. model underwent rigorous using one-factor-at-a-time (OFAT) algorithm, focus essential parameters such as batch size, learning rate, optimizer, weight decay, momentum, dropout, epochs. Subsequently, random search (RS) 100 configurations performed results OFAT. Among them, C47 demonstrated 0.99268 (a 0.39% improvement), 0.99190 (0.09%), 0.99348 (0.76%), 0.99262 (0.07%). suggest that final works efficiently is capable accurately detecting identifying diseases, making it suitable practical farming applications.
Language: Английский
Citations
2Agronomy, Journal Year: 2025, Volume and Issue: 15(3), P. 537 - 537
Published: Feb. 23, 2025
In order to accurately detect the maturity of chili peppers under different lighting and natural environmental scenarios, in this study, we propose a lightweight detection model, YOLOv8-CBSE, based on YOLOv8n. By replacing C2f module original model with designed C2CF module, integrates advantages convolutional neural networks Transformer architecture, improving model’s ability extract local features global information. Additionally, SRFD DRFD modules are introduced replace layers, effectively capturing at scales enhancing diversity adaptability through feature fusion mechanism. To further improve accuracy, EIoU loss function is used instead CIoU provide more comprehensive The results showed that average precision (AP) YOLOv8-CBSE for mature immature was 90.75% 85.41%, respectively, F1 scores mean (mAP) 81.69% 88.08%, respectively. Compared YOLOv8n, score mAP improved increased by 0.46% 1.16%, effect pepper scenarios improved, which proves robustness YOLOv8-CBSE. also maintains design size only 5.82 MB, its suitability real-time applications resource-constrained devices. This study provides an efficient accurate method detecting environments, great significance promoting intelligent precise agricultural management.
Language: Английский
Citations
1Lecture notes in networks and systems, Journal Year: 2025, Volume and Issue: unknown, P. 321 - 329
Published: Jan. 1, 2025
Language: Английский
Citations
0Journal of Agriculture and Food Research, Journal Year: 2025, Volume and Issue: unknown, P. 101840 - 101840
Published: March 1, 2025
Language: Английский
Citations
0Fire, Journal Year: 2025, Volume and Issue: 8(4), P. 142 - 142
Published: April 2, 2025
The increasing frequency and severity of agricultural fires pose significant threats to food security, economic stability, environmental sustainability. Traditional fire-detection methods, relying on satellite imagery ground-based sensors, often suffer from delayed response times high false-positive rates, limiting their effectiveness in mitigating fire-related damages. In this study, we propose an advanced deep learning-based framework that integrates the Single-Shot MultiBox Detector (SSD) with computationally efficient MobileNetV2 architecture. This integration enhances real-time fire- smoke-detection capabilities while maintaining a lightweight deployable model suitable for Unmanned Aerial Vehicle (UAV)-based monitoring. proposed was trained evaluated custom dataset comprising diverse fire scenarios, including various conditions intensities. Comprehensive experiments comparative analyses against state-of-the-art object-detection models, such as You Only Look Once (YOLO), Faster Region-based Convolutional Neural Network (Faster R-CNN), SSD-based variants, demonstrated superior performance our model. results indicate approach achieves mean Average Precision (mAP) 97.7%, significantly surpassing conventional models detection speed 45 frames per second (fps) requiring only 5.0 GFLOPs computational power. These characteristics make it particularly deployment edge-computing environments, UAVs remote monitoring systems.
Language: Английский
Citations
0Smart Agricultural Technology, Journal Year: 2025, Volume and Issue: unknown, P. 100966 - 100966
Published: April 1, 2025
Language: Английский
Citations
0Computers in Biology and Medicine, Journal Year: 2025, Volume and Issue: 187, P. 109778 - 109778
Published: Feb. 8, 2025
Language: Английский
Citations
0Applied Sciences, Journal Year: 2025, Volume and Issue: 15(6), P. 3164 - 3164
Published: March 14, 2025
The digitalization of historical documents is interest for many reasons, including preservation, accessibility, and searchability. One the main challenges with digitization old newspapers involves complex layout analysis, where content types document must be determined. In this context, paper presents an evaluation most recent YOLO methods analysis layouts. Initially, a new dataset called BHN was created made available, standing out as first Brazilian detection. experiments were held using YOLOv8, YOLOv9, YOLOv10, YOLOv11 architectures. For training, validation, testing models, following newspaper datasets combined: BHN, GBN, Printed BlaLet GT. Recall, precision, mean average precision (mAP) used to evaluate performance models. results indicate that best performer Recalltest 81% mAPtest 89%. This provides insights on advantages these models in detection also promotes improvement image conversion into editable accessible formats.
Language: Английский
Citations
0Neural Computing and Applications, Journal Year: 2025, Volume and Issue: unknown
Published: April 26, 2025
Language: Английский
Citations
0Case Studies in Construction Materials, Journal Year: 2024, Volume and Issue: 21, P. e04048 - e04048
Published: Nov. 29, 2024
Language: Английский
Citations
2