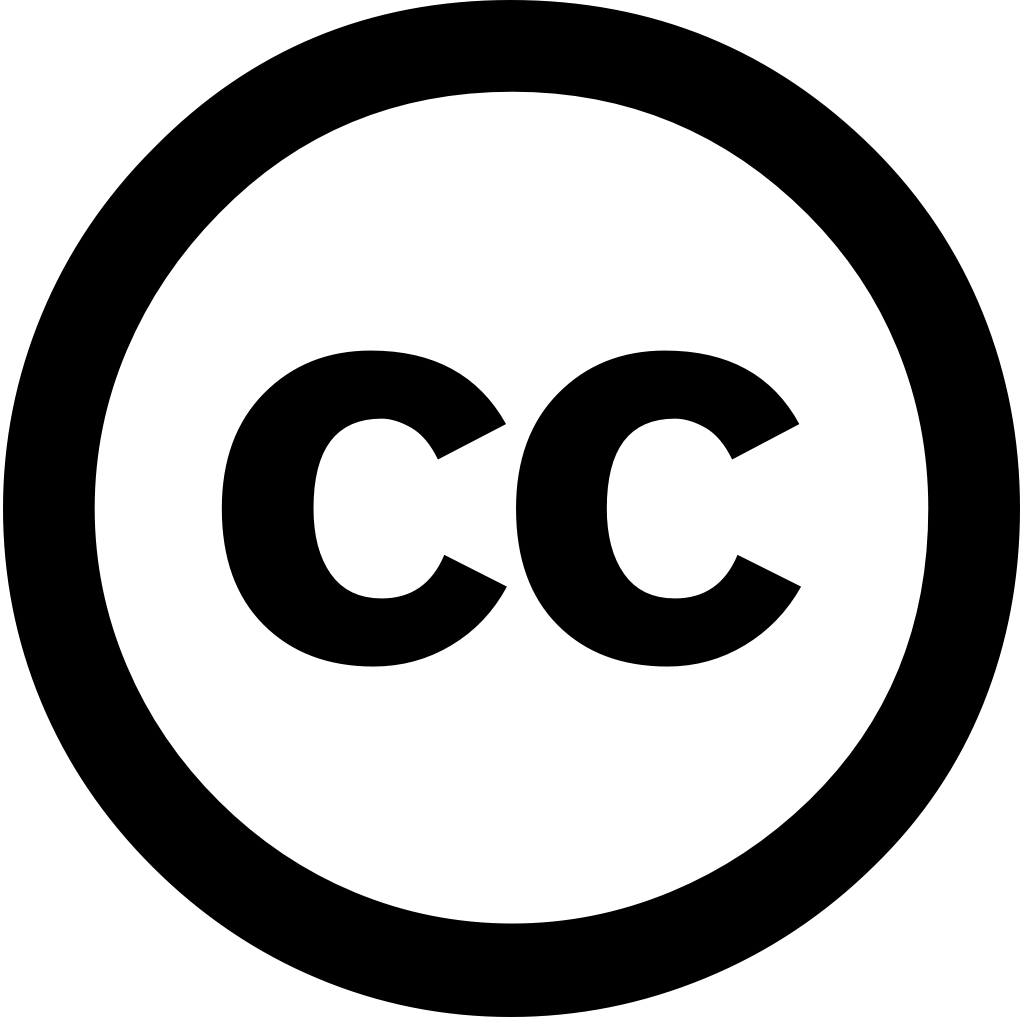
Computers, Journal Year: 2025, Volume and Issue: 14(4), P. 124 - 124
Published: March 27, 2025
Federated Learning (FL) is a transformative decentralized approach in machine learning and deep learning, offering enhanced privacy, scalability, data security. This review paper explores the foundational concepts, architectural variations of FL, prominent aggregation algorithms like FedAvg, FedProx, FedMA, diverse innovative applications thermal comfort optimization, energy prediction, healthcare, anomaly detection within smart buildings. By enabling collaborative model training without centralizing sensitive data, FL ensures privacy robust performance across heterogeneous environments. We further discuss integration with advanced technologies, including digital twins 5G/6G networks, demonstrate its potential to revolutionize real-time monitoring, optimize resources. Despite these advances, still faces challenges, such as communication overhead, security issues, non-IID handling. Future research directions highlight development adaptive methods, measures, hybrid architectures fully leverage FL’s driving innovative, secure, efficient intelligence for next generation
Language: Английский