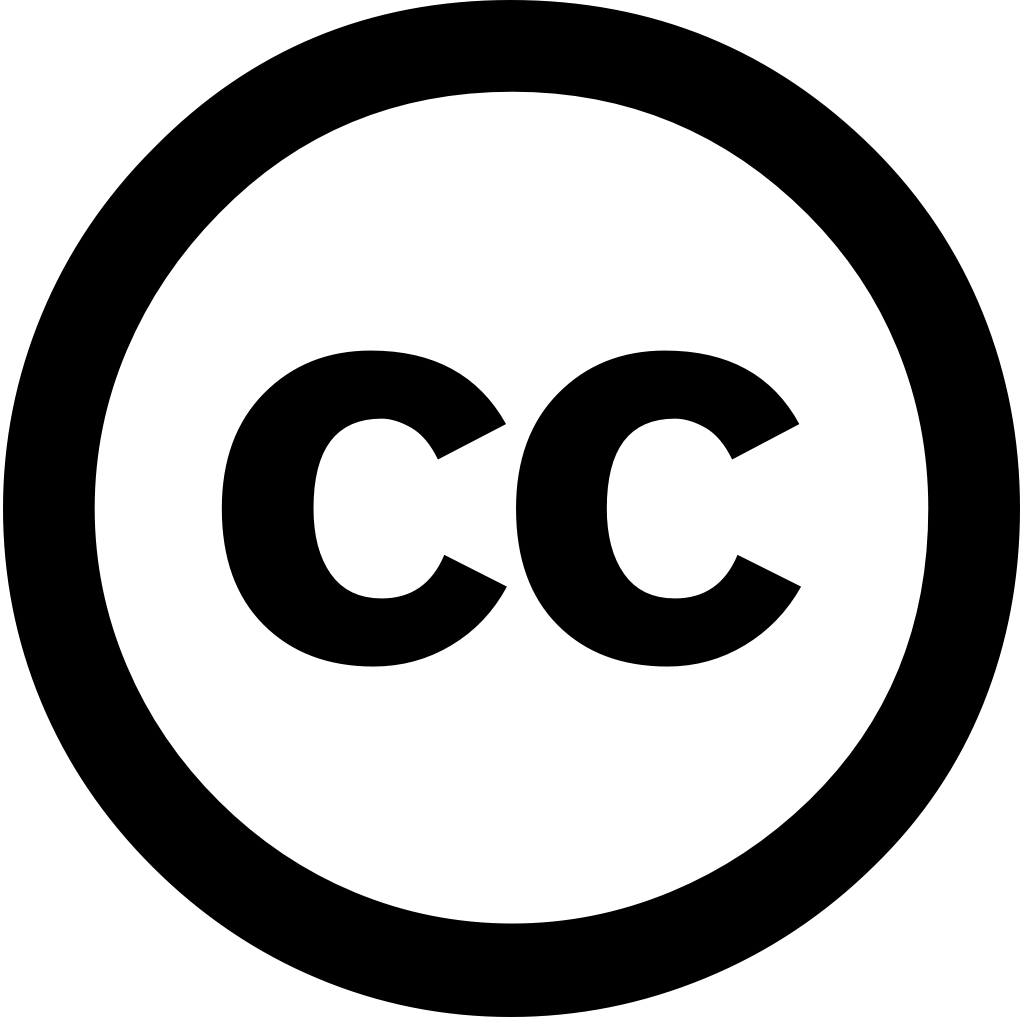
Systems, Journal Year: 2025, Volume and Issue: 13(3), P. 187 - 187
Published: March 7, 2025
Within globalization, the significance of urban innovation cooperation has become increasingly evident. However, faces challenges due to various factors—social, economic, and spatial—making it difficult for traditional methods uncover intricate nonlinear relationships among them. Consequently, this research concentrates on cities within Yangtze River Delta region, employing an explainable machine learning model that integrates eXtreme Gradient Boosting (XGBoost), SHapley Additive exPlanations (SHAP), Partial Dependence Plots (PDPs) investigate interactive effects multidimensional factors impacting cooperation. The findings indicate XGBoost outperforms LR, SVR, RF, GBDT in terms accuracy effectiveness. Key results are summarized as follows: (1) Urban exhibits different phased characteristics. (2) There exist between factors, them, Scientific Technological dimension contributes most (30.59%) significant positive promoting effect later stage after surpassing a certain threshold. In Social Economic (23.61%), number Internet Users (IU) individually. Physical Space (20.46%) generally mutation points during early stages development, with overall predominantly characterized by trends. (3) Through application PDP, is further determined IU synergistic per capita Foreign Direct Investment (FDI), public library collections (LC), city night light data (NPP), while exhibiting negative antagonistic Average Annual Wage Staff (AAS) Enterprises above Designated Size Industry (EDS). (4) For at developmental stages, tailored development proposals should be formulated based single-factor contribution multifactor interaction effects. These insights enhance our understanding elucidate influencing factors.
Language: Английский