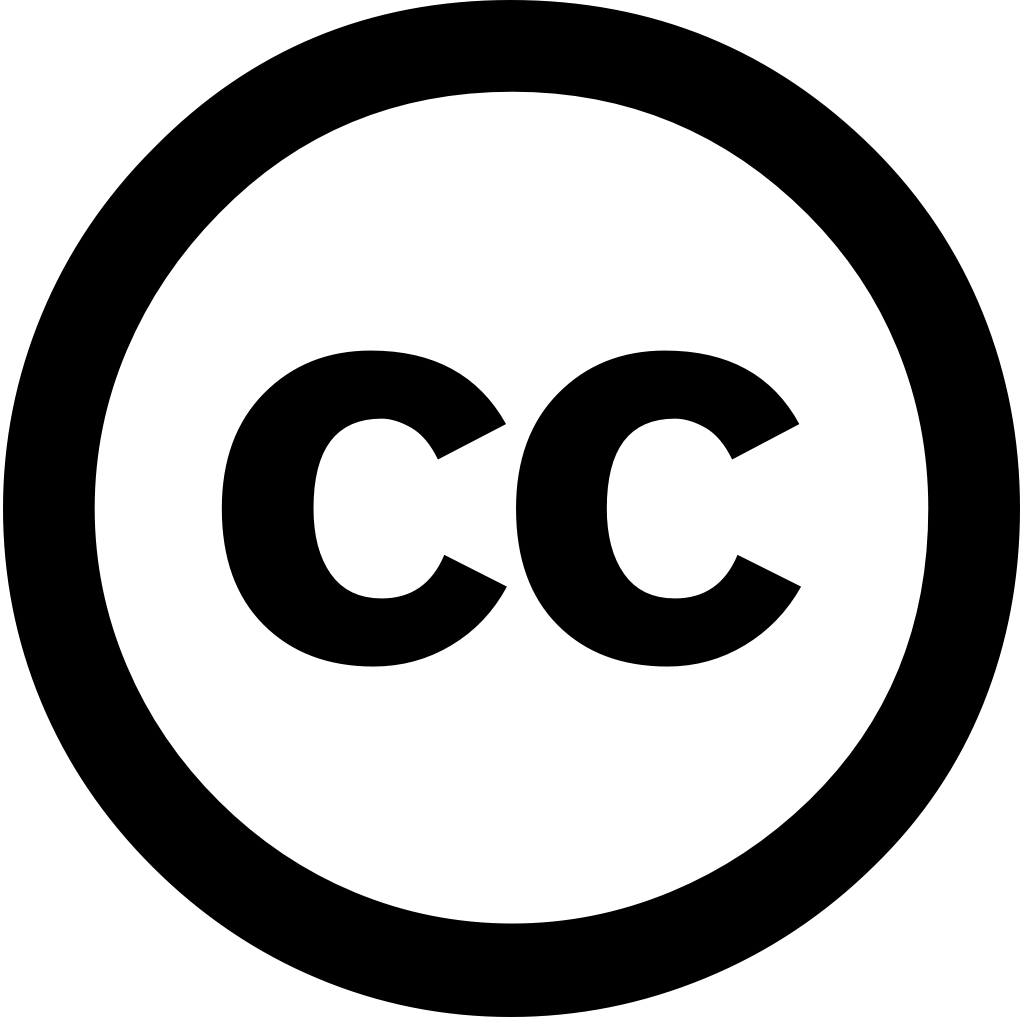
Energies, Journal Year: 2025, Volume and Issue: 18(8), P. 1961 - 1961
Published: April 11, 2025
The aim of this study is to compare the performance various deep learning models in predicting arrival and charging time an electric vehicle at a station. objective identify most precise model capable from driver’s location Initially, effort was made ascertain which type offers superior prediction accuracy, characterized by low error rates high success scores. To end, examined capabilities LSTM (Long Short-Term Memory), GRU (Gated Recurrent Unit), Prophet, ARIMA (Autoregressive Integrated Moving Average) models, were trained with historical data using metrics. These metrics included R2 (R-squared)success metric, MAE (Mean Absolute Error) MSE Squared metrics, DTW (Dynamic Time Warping) metric scores, selection. In has rate 0.38, 0.50, Prophet 0.61, 2.31. For 2.92, 3.05, 4.21, 65.54. 0.99, 0.13, −2.19. distance ratio 125.5, 126.9, 185.9, 454.9. Based on these score values, it decided that accurate estimation. After observing model, capability demonstrated through interface program for stations province Kocaeli, serves as model’s real-world application area. main contribution article LSTM, GRU, approaches, are preferred many studies, used first process estimating time.
Language: Английский