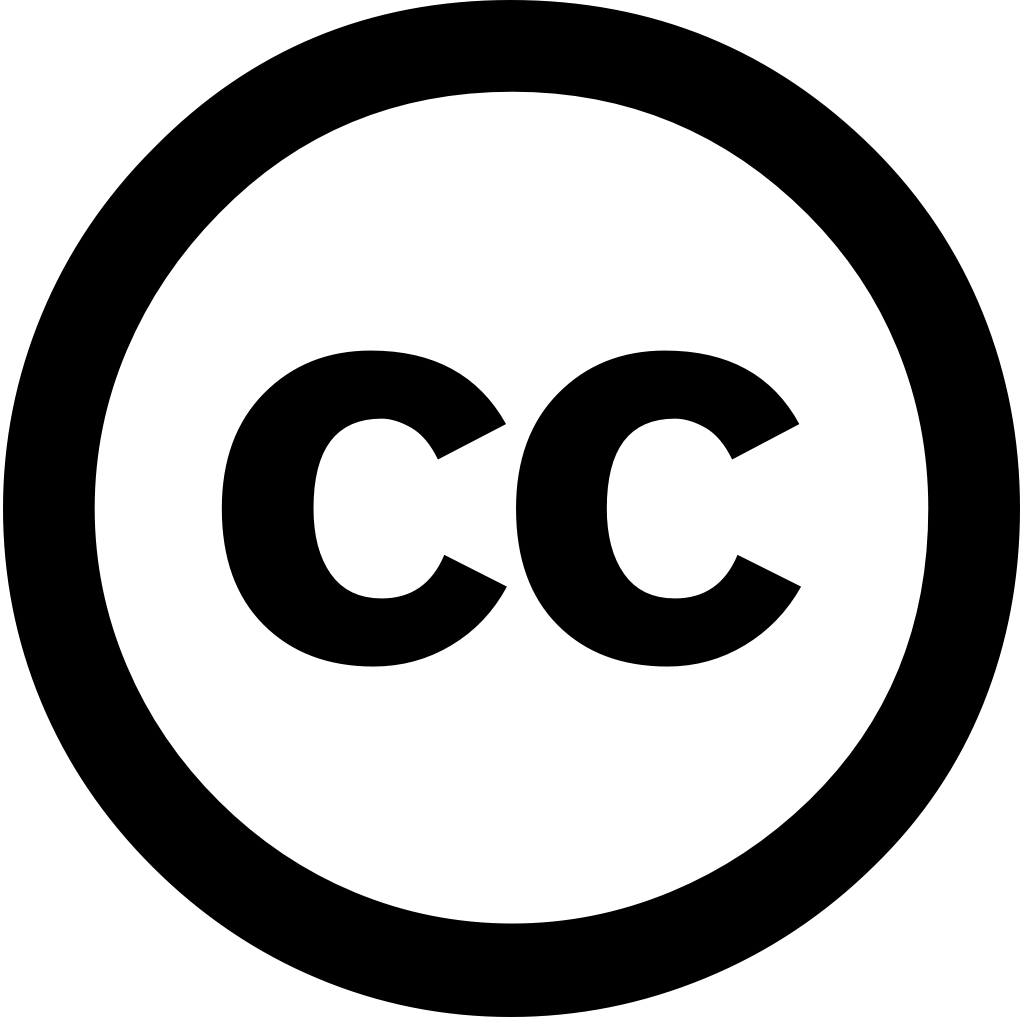
Neurotherapeutics, Journal Year: 2022, Volume and Issue: 19(1), P. 186 - 208
Published: Jan. 1, 2022
Language: Английский
Neurotherapeutics, Journal Year: 2022, Volume and Issue: 19(1), P. 186 - 208
Published: Jan. 1, 2022
Language: Английский
Ageing Research Reviews, Journal Year: 2024, Volume and Issue: 96, P. 102290 - 102290
Published: April 1, 2024
Language: Английский
Citations
12PLoS Biology, Journal Year: 2024, Volume and Issue: 22(4), P. e3002607 - e3002607
Published: April 30, 2024
Unbiased data-driven omic approaches are revealing the molecular heterogeneity of Alzheimer disease. Here, we used machine learning to integrate high-throughput transcriptomic, proteomic, metabolomic, and lipidomic profiles with clinical neuropathological data from multiple human AD cohorts. We discovered 4 unique multimodal profiles, one them showing signs poor cognitive function, a faster pace disease progression, shorter survival disease, severe neurodegeneration astrogliosis, reduced levels metabolomic profiles. found this profile be present in affected cortical regions associated higher Braak tau scores significant dysregulation synapse-related genes, endocytosis, phagosome, mTOR signaling pathways altered early late stages. cross-omics integration transcriptomic an SNCA mouse model revealed overlapping signature. Furthermore, leveraged single-nuclei RNA-seq identify distinct cell-types that most likely mediate Lastly, identified clusters uncovered cerebrospinal fluid biomarkers poised monitor progression possibly cognition. Our analyses provide novel critical insights into AD.
Language: Английский
Citations
12Alzheimer s Research & Therapy, Journal Year: 2025, Volume and Issue: 17(1)
Published: Jan. 7, 2025
Alzheimer's disease (AD) is a complex neurodegenerative disorder with largely unexplored epigenetic landscape. This study employs an innovative approach that integrates multi-omics analysis and explainable machine learning to explore the regulatory mechanisms underlying signature of PRRT1 implicated in AD. Through comprehensive DNA methylation transcriptomic profiling, we identified distinct signatures associated gene expression AD patient samples compared healthy controls. Utilizing interpretable models ELMAR analysis, dissected relationships between these patterns, revealing novel elements pathways. Finally, genes were investigated experimentally. ten signatures, constructed diagnostic model, utilized various bioinformatics methods create epigenomic map. Subsequently, R package was used integrate data identify upstream transcription factor MAZ for PRRT1. experiments confirmed interaction PRRT1, which mediated apoptosis autophagy adopts strategy molecular experiments, providing new insights into demonstrating importance elucidating mechanisms.
Language: Английский
Citations
1Reviews in Cardiovascular Medicine, Journal Year: 2025, Volume and Issue: 26(1)
Published: Jan. 8, 2025
Background: This study aimed to analyze the metabolic risk factors for microcirculation disorders in patients with unstable angina (UA) after percutaneous coronary intervention (PCI), evaluating their predictive value developing disorders. Methods: A single-center retrospective design was used, which included 553 UA who underwent PCI. The angiographic microcirculatory resistance (AMR) index calculated based on angiography data. Patients were divided into two groups according post-PCI AMR values: a ≤2.50 group and >2.50 group. Variables multivariate regression model through univariate variance inflation factor (VIF) screening. Subgroup analyses conducted by sex further evaluate of selected variables overall sample. total sample randomly split 7:3 ratio training validation sets. nomogram sets then constructed visualize these predictions. discrimination calibration prediction evaluated using receiver operating characteristic (ROC) curve curve. Results: had higher percentage females, increased incidence diabetes, elevated fasting blood glucose (FBG), glycated hemoglobin (HbA1c), triglyceride (TG), cholesterol (TC), low-density lipoprotein (LDL-C), very (VLDL-C), lipoprotein(a) (Lp(a)) levels (p < 0.05). Logistic analysis identified HbA1c, TG, LDL-C, Lp(a) as independent predictors adjusting confounders. confirmed no significant interaction between > set, area under (AUC) ROC 0.824 set 0.746 set. curves showed good fit (training set: p = 0.219; 0.258). Conclusions: are post-PCI. provides accuracy.
Language: Английский
Citations
1Neurotherapeutics, Journal Year: 2022, Volume and Issue: 19(1), P. 186 - 208
Published: Jan. 1, 2022
Language: Английский
Citations
35