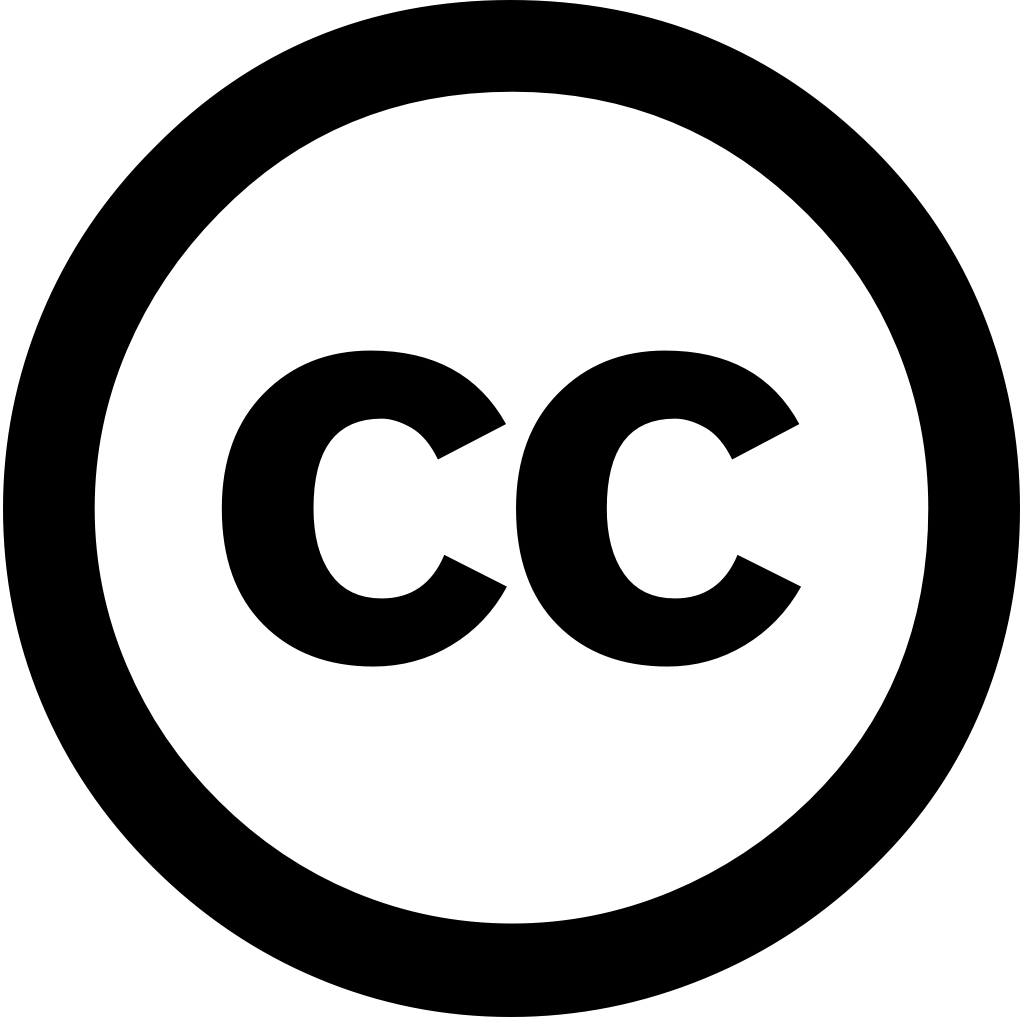
Journal of Experimental Orthopaedics, Journal Year: 2024, Volume and Issue: 11(4)
Published: Oct. 1, 2024
Language: Английский
Journal of Experimental Orthopaedics, Journal Year: 2024, Volume and Issue: 11(4)
Published: Oct. 1, 2024
Language: Английский
Current Reviews in Musculoskeletal Medicine, Journal Year: 2024, Volume and Issue: 17(6), P. 185 - 206
Published: April 8, 2024
Language: Английский
Citations
4Arthroscopy The Journal of Arthroscopic and Related Surgery, Journal Year: 2025, Volume and Issue: unknown
Published: Feb. 1, 2025
Language: Английский
Citations
0Frontiers in Oncology, Journal Year: 2025, Volume and Issue: 15
Published: Feb. 28, 2025
Purpose This meta-analysis was conducted to assess the diagnostic performance of artificial intelligence (AI) based on imaging for detecting lymph node metastasis (LNM) among cervical cancer patients and compare its with that radiologists. Methods A comprehensive literature search across PubMed, Embase, Web Science identify relevant studies published up October 2024. The followed Preferred Reporting Items Systematic Reviews Meta-Analyses Diagnostic Test Accuracy (PRISMA-DTA) guidelines. Studies evaluating accuracy AI models in LNM through computed tomography (CT), magnetic resonance (MRI), positron emission tomography/computed (PET/CT) were included. Pathology served as reference standard validation. bivariate random-effects model employed estimate pooled sensitivity specificity, both presented alongside 95% confidence intervals (CIs). Bias assessed revised Quality Assessment Studies-2 (QUADAS-2) tool. Study heterogeneity examined I 2 statistic. Meta-regression when significant (I > 50%) observed. Results total 23 included this meta-analysis. quality bias acceptable. However, substantial observed studies. Internal validation sets comprised 1,490 patients. sensitivity, area under curve (AUC) 0.83 (95% CI: 0.78-0.87), 0.78 0.74-0.82) 0.87 0.84-0.90), respectively. External six 298 AUC 0.70 0.56-0.81), 0.85 0.66-0.95) 0.76 0.72-0.79), For radiologists, eight 644 included; 0.54 0.42-0.66), 0.79 0.59-0.91) 0.65 0.60-0.69), Conclusions Imaging-based demonstrates higher than Prospective rigorous standardization well further research external datasets, are necessary confirm results their practical clinical applicability. Review Registration https://www.crd.york.ac.uk/PROSPERO , identifier CRD42024607074.
Language: Английский
Citations
0Arthroscopy The Journal of Arthroscopic and Related Surgery, Journal Year: 2025, Volume and Issue: unknown
Published: April 1, 2025
Language: Английский
Citations
0Current Reviews in Musculoskeletal Medicine, Journal Year: 2025, Volume and Issue: unknown
Published: April 30, 2025
Abstract Purpose of Review Artificial intelligence (AI) has rapidly proliferated though medicine with many novel applications to improve patient care and optimize healthcare delivery. This review investigates recent literature surrounding the influence AI imaging technologies on spine surgical practice diagnosis. Recent Findings Robotic-assisted pedicle screw placement been shown increase rate clinically acceptable while increasing operative time. have also promise in creating 3D reducing radiation exposure. Several models using various modalities reliably identify vertebral osteoporotic fractures, stenosis cancers. Summary Complex spinal anatomy pathology as well integration robotics make surgery a promising field for deployment AI-based technologies. Imaging-based projects show potential enhance diagnostic efficiency, facilitate trainee learning outcomes.
Language: Английский
Citations
0Arthroscopy Sports Medicine and Rehabilitation, Journal Year: 2024, Volume and Issue: 6(3), P. 100940 - 100940
Published: April 8, 2024
PurposeTo 1) develop a deep learning model for detection of Segond fractures on AP knee radiographs and 2) to compare performance that trained human experts.MethodsAP were retrieved from the Hospital Special Surgery ACL Registry, which enrolled patients between 2009 2013. All images corresponded who underwent reconstruction by one 23 surgeons included in registry data. Images categorized into two classes based radiographic evidence fracture manually annotated. Seventy percent used populate training set, while 20% 10% reserved validation test sets, respectively. set expert observers, including an orthopedic surgery sports medicine fellow fellowship-trained surgeon with over 10 years experience.ResultsA total 324 retrieved, 34 (10.4%) demonstrated fracture. The overall mean average precision (mAP) was 0.985, this maintained class (mAP = 0.978, 0.844, recall 1). 100% accuracy perfect sensitivity specificity when applied independent testing ability meet or exceed all cases. Compared fellow, required 0.3% time needed evaluate classify set.ConclusionA developed internally validated accuracy, small without fractures. superior compared observers.Clinical RelevanceDeep can be automated identification radiographs, leading improved diagnosis easily missed concomitant injuries, lateral meniscus tears. Automated also enable large-scale studies incidence clinical significance these fractures, may lead management outcomes injuries.
Language: Английский
Citations
1Journal of Experimental Orthopaedics, Journal Year: 2024, Volume and Issue: 11(4)
Published: Oct. 1, 2024
Language: Английский
Citations
0