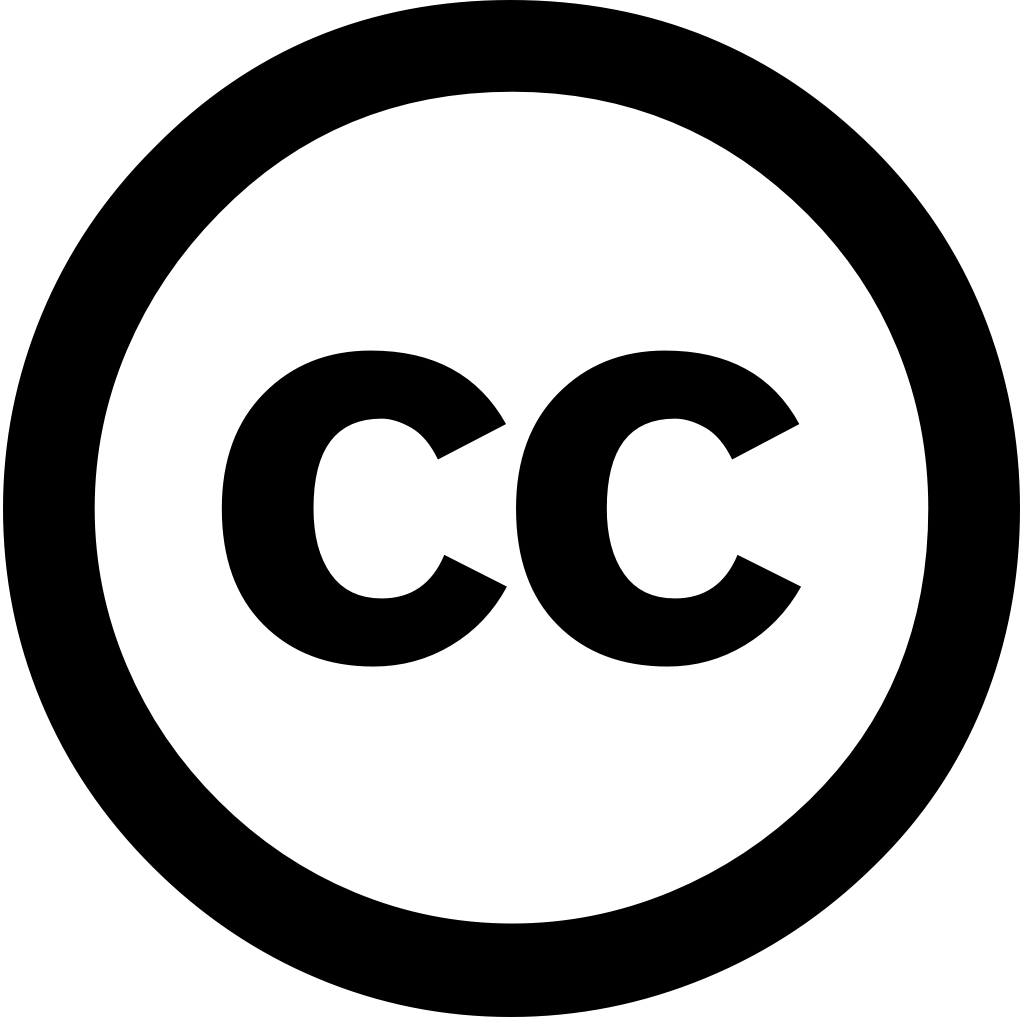
Atmosphere, Journal Year: 2025, Volume and Issue: 16(4), P. 429 - 429
Published: April 8, 2025
The escalation of industrialization has worsened air quality, underscoring the essential need for accurate forecasting to inform policies and protect public health. Current research primarily emphasized individual spatiotemporal features prediction, neglecting interconnections between these features. To address this, we proposed generative Comprehensive Scale Spatiotemporal Fusion Air Quality Predictor (CSST-AQP). novel dual-branch architecture combines multi-scale spatial correlation analysis with adaptive temporal modeling capture complex interactions in pollutant dispersion enhanced pollution forecasting. Initially, a fusion preprocessing module based on localized high-correlation encodes multidimensional quality indicators geospatial data into unified Then, core employs collaborative framework: processing branch extracts at varying granularities, an enhancement concurrently models local periodicities global evolutionary trends. feature engine hierarchically integrates spatiotemporally relevant regional scales while aggregating from related sites. In experimental results across 14 Chinese regions, CSST-AQP achieves state-of-the-art performance compared LSTM-based networks RMSE 6.11–9.13 μg/m3 R2 0.91–0.93, demonstrating highly robust 60 h capabilities diverse pollutants.
Language: Английский