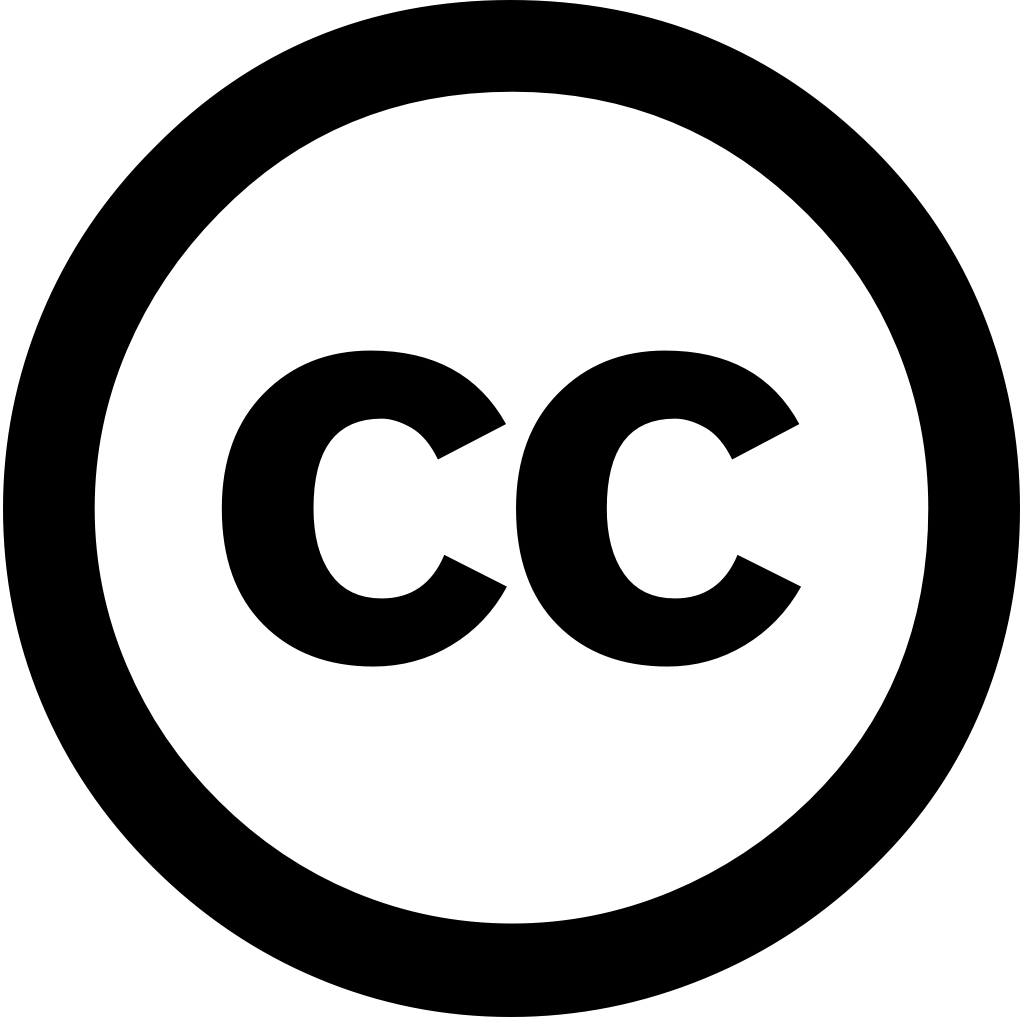
Agronomy, Journal Year: 2025, Volume and Issue: 15(5), P. 1125 - 1125
Published: May 2, 2025
To address the challenge of precise picking point localization in morphologically diverse safflower plants, this study proposes PointSafNet—a novel three-stage 3D cloud analysis framework with distinct architectural and methodological innovations. In Stage I, we introduce a multi-view reconstruction pipeline integrating Structure from Motion (SfM) Multi-View Stereo (MVS) to generate high-fidelity plant clouds. II develops dual-branch architecture employing Star modules for multi-scale hierarchical geometric feature extraction at organ level (filaments frui balls), complemented by Context-Anchored Attention (CAA) mechanism capture long-range contextual information. This synergistic learning approach addresses morphological variations, achieving 86.83% segmentation accuracy (surpassing PointNet++ 7.37%) outperforming conventional models. III an optimized combining dual-centroid spatial vectorization Oriented Bounding Box (OBB)-based proximity analysis, resolving coordinate across plants 90% positioning 68.82% mean IoU (13.71% improvement). The experiments demonstrate that PointSafNet systematically integrates reconstruction, learning, reasoning provide visual guidance robotic harvesting systems complex canopies. framework’s dual emphasis on innovation modeling offers generalizable solution precision agriculture tasks involving safflowers.
Language: Английский