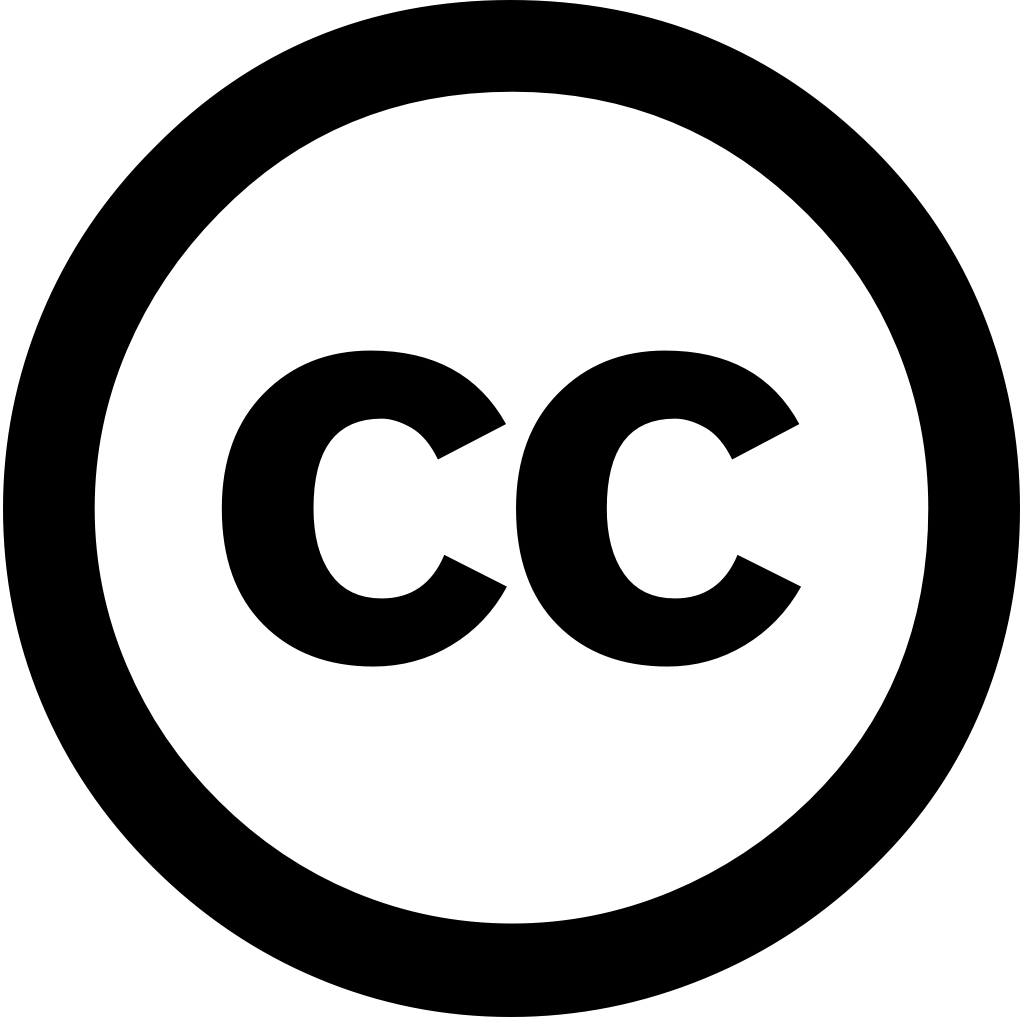
Animals, Journal Year: 2025, Volume and Issue: 15(8), P. 1127 - 1127
Published: April 13, 2025
In modern large-scale pig farming, accurately identifying sow estrus and ensuring timely breeding are crucial for maximizing economic benefits. However, the short duration of reliance on subjective human judgment pose significant challenges precise insemination timing. To enable non-contact, automated detection, this study proposes an improved algorithm, Enhanced Context-Attention YOLO (ECA-YOLO), based YOLOv11. The model utilizes ocular appearance features—eye’s spirit, color, shape, morphology—across different stages as key indicators. MSCA module enhances small-object detection efficiency, while PPA GAM modules improve feature extraction capabilities. Additionally, Adaptive Threshold Focal Loss (ATFL) function increases model’s sensitivity to hard-to-classify samples, enabling accurate stage classification. was trained validated a dataset comprising 4461 images eyes during benchmarked against YOLOv5n, YOLOv7tiny, YOLOv8n, YOLOv10n, YOLOv11n, Faster R-CNN. Experimental results demonstrate that ECA-YOLO achieves mean average precision (mAP) 93.2%, F1-score 88.0%, with 5.31M parameters, FPS reaches 75.53 frames per second, exhibiting superior overall performance. findings confirm feasibility using features highlight potential real-time, monitoring under complex farming conditions. This lays groundwork in intensive farming.
Language: Английский